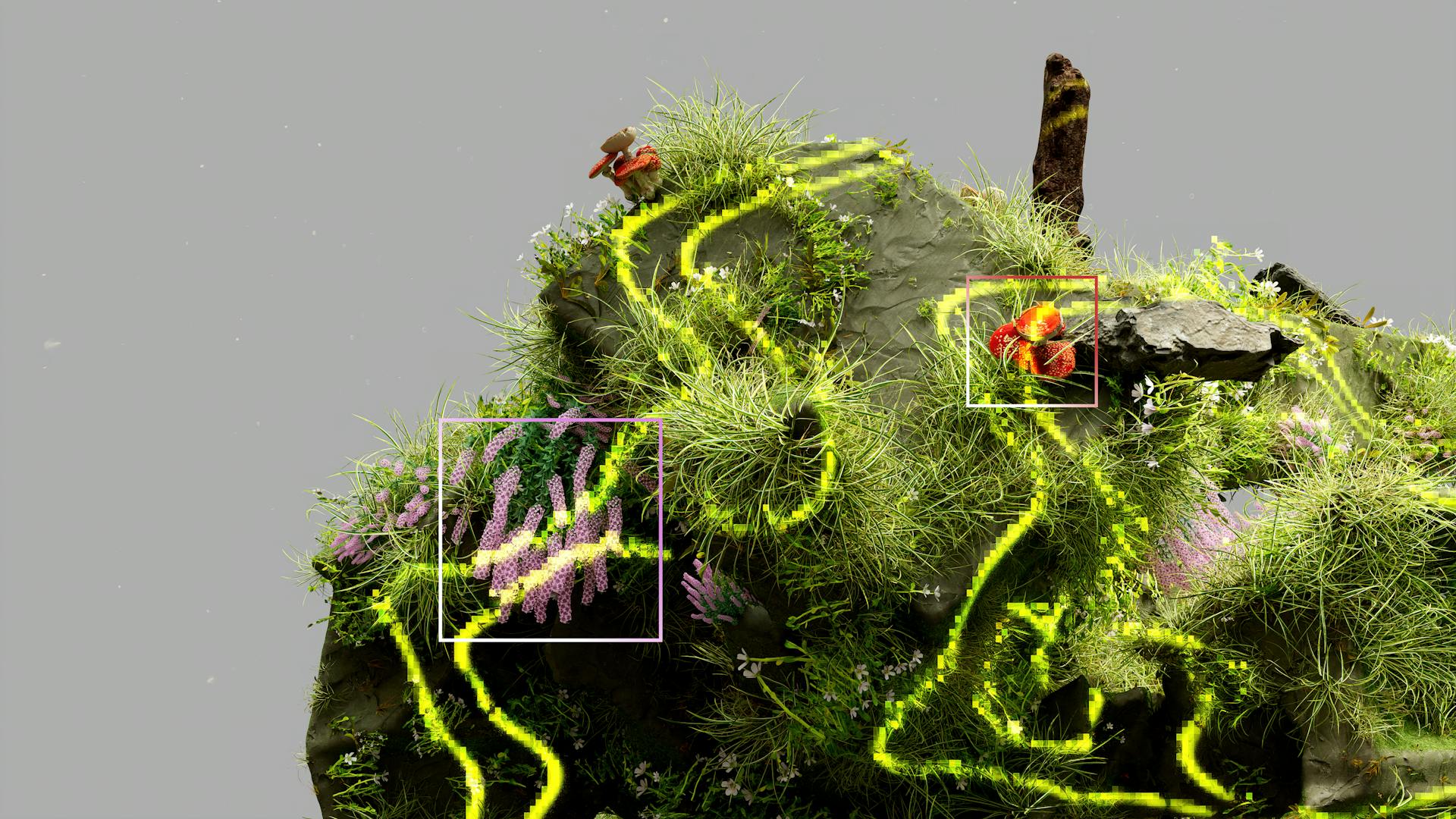
Being an AI ML Product Manager is a complex role that requires a unique blend of technical expertise, business acumen, and leadership skills.
To succeed in this role, you need to have a strong understanding of machine learning and artificial intelligence, including their applications and limitations.
A good AI ML Product Manager should be able to communicate technical concepts to non-technical stakeholders, making them an essential part of the product development process.
Effective collaboration with cross-functional teams, including engineering, design, and data science, is crucial for delivering successful AI ML products.
For another approach, see: Generative Ai Product Prototype
Understanding AI/ML Product Management
Product managers need to appreciate the potential and potential pitfalls of AI and machine learning to drive innovation and competitive advantage.
To be effective in directing and guiding your team, you should grasp the basics of how algorithms work, the data science principles involved, and the challenges in training and deploying models. This clarity empowers you to steer the product direction, set realistic expectations, and handle stakeholder interactions.
Understanding AI and ML empowers product managers to create adaptable, high-performance products that meet dynamic market demands and provide exceptional user experiences.
You might enjoy: Generative Ai Overview for Project Managers Answers
Why Understand
Understanding AI/ML is crucial for product managers to drive innovation and competitive advantage. Appreciating the potential and pitfalls of these technologies empowers PMs to create adaptable, high-performance products that meet dynamic market demands and provide exceptional user experiences.
Data is the lifeblood of AI/ML systems, and its quality, quantity, and relevancy determine the effectiveness of even the most sophisticated algorithms. Recognizing the pivotal role of data is paramount for product managers.
Structured data is organized and formatted in relational databases, making it easily searchable, while unstructured data lacks a specific form or structure, making it more challenging to analyze and process. Both types are invaluable in AI/ML.
The journey of utilizing data in AI/ML involves several critical steps, starting with data collection, followed by cleaning, processing, and feeding into algorithms. Each step requires meticulous attention to detail to ensure reliable and robust AI models.
Large Language Models, like ChatGPT, are built to process and produce human-like text, and are an example of semi-supervised learning.
A fresh viewpoint: Evolutionary Algorithms
Understanding the Tech
Understanding the tech behind AI/ML is crucial for product managers to effectively direct and guide their teams. You should grasp the basics of how algorithms work, the data science principles involved, and the challenges in training and deploying models.
To be effective, product managers should have a clear understanding of the mechanics of ML. This includes knowing how algorithms work, the data science principles involved, and the challenges in training and deploying models. The clearer you are about the mechanics of ML, the better you can steer the product direction, set realistic expectations, and handle stakeholder interactions.
Data is the lifeblood of AI/ML systems, with the quality, quantity, and relevancy of data determining the effectiveness of even the most sophisticated algorithms. Data serves as the foundational layer upon which models are trained, and it is the nuances within this data that these models learn to interpret, predict, and act upon.
Readers also liked: Generative Ai for Product Managers
Data can be broadly classified into structured and unstructured types. Structured data is organized and formatted in a way that makes it easily searchable in relational databases, while unstructured data lacks a specific form or structure, often making it more challenging to analyze and process.
Here's a breakdown of the types of data:
- Structured data: user names, purchase history, addresses
- Unstructured data: emails, social media posts, videos, images
The journey of utilizing data in AI/ML involves several critical steps, starting with data collection. This process involves gathering relevant information from various sources, which could be sensors, user interactions, databases, or even external platforms. Once collected, the data undergoes a cleaning process to identify and rectify inconsistencies, errors, and anomalies that could distort AI/ML model outcomes.
Prioritization
Prioritization is a skill that you acquire over time, and it's essential for maintaining smart, efficient, and effective workflows in your product teams.
A good machine learning product manager doesn't get overwhelmed or intimidated by a long to-do list. You must look at your list of tasks and make use of your own insightful and dynamic thinking abilities to decide which tasks are the most important and prioritize them accordingly.
For another approach, see: Generative Ai Product Design
Management training courses can help you develop this skill, but it's also something that you can start working on by looking at tasks and duties on a deeper level and understanding the importance of each one as well as why you need to get them done.
Ranking each task based on urgency and importance is a great way to point yourself in the right direction and stay focused on what needs to be accomplished.
Ethical Considerations
Ethical considerations are crucial in AI/ML product management, ensuring that these technologies are used to respect human rights, ensure privacy, and work towards the common good.
The rise of AI and ML has ushered in a new era of technological prowess, but it also brings the imperative to use these technologies ethically. AI's potential to affect societies, economies, and personal lives makes its ethical deployment a paramount concern.
Biases in AI systems are often a reflection of the biases present in society, and can seep into AI models through skewed data, biased training, or objectives. A recruitment AI trained on historical corporate data might favor a particular demographic over others.
A fresh viewpoint: Ethical Implications of Ai
Ensuring fairness means recognizing these biases and actively working to mitigate them throughout the AI system's design and implementation. This includes diverse representation within AI product teams and periodic audits of AI/ML models.
Robust AI/ML governance is paramount to advance ethical decision-making in AI product management. Organizations should frame clear guidelines and principles that direct AI/ML development.
Product managers emerge as key players in this governance framework, acting as the conduit between technical teams, stakeholders, and end-users, guaranteeing that ethical imperatives are consistently prioritized throughout the product's lifecycle.
Role and Responsibilities
As an AI ML Product Manager, your role is multifaceted and requires a unique blend of technical and business skills. You'll be responsible for overseeing various products and product lines, managing production, and working on the product roadmap based on the product lifecycle.
Machine learning product managers can have multiple roles within an organization, depending on the industry, size of the company, and product lines. They work with various product teams, development teams, engineering teams, and other stakeholders.
Some of the key responsibilities of an AI ML Product Manager include:
- Data Literacy: Understanding machine learning concepts, basic AI concepts, and data science fundamentals.
- Problem Mapping: Identifying present problems and determining which can be solved using machine learning.
- Explainability: Explaining complex machine learning processes in simple terms to customers, upper management, and other stakeholders.
- Acceptance Criteria: Determining what things need attention to set up machine-learning algorithms and models.
- Communication: Working with all other teams to ensure ML processes are understood and working.
In addition to these responsibilities, AI ML Product Managers also need to be able to build and manage AI models, work closely with data scientists and engineers, and ensure that AI tech is implemented in a responsible way. They must also be able to collaborate with internal teams and external partners to deliver AI/ML solutions to meet customer needs.
Some of the specific duties and tasks of an AI ML Product Manager include:
- Overseeing various products and product lines, and managing production.
- Working on the product roadmap based on the product lifecycle.
- Assisting the product team in the development, creation, and management of new products.
- Collaborating with internal teams and external partners to deliver AI/ML solutions.
- Defining data and annotation strategies for all machine learning projects.
Ultimately, as an AI ML Product Manager, you'll be responsible for driving the product vision, strategy, and roadmap, and working closely with engineering, sales, marketing, and customer success teams to deliver AI products and ensure their market success.
Developing and Implementing
Developing and implementing AI products requires a deep understanding of various AI technologies, including Generative AI, Machine Learning, Computer Vision, Robotic Process Automation, and Deep Learning. These technologies can be used to create user-friendly and market-ready solutions.
If this caught your attention, see: Ai Ml Technology
AI PMs must be aware of the potential and limitations of each technology to effectively align them with business objectives. For example, Generative AI can be used to create Large Language Models like GPT-4 or Google’s Gemini, which can understand and generate human-like text.
Developing AI products involves incorporating these technologies in a way that meets business needs. Here are some examples of AI products that leverage the Unique Value Propositions (UVPs) of the companies that made them:
By understanding the capabilities and limitations of these technologies, AI PMs can develop and implement AI products that meet business needs and deliver value to customers.
Developing
Developing AI products is a complex task that requires understanding different types of AI technology. These include Generative AI, Machine learning, Computer vision, Robotic Process Automation, and Deep learning.
AI PMs must understand the potential and limitations of these technologies to align them with business objectives effectively. This means knowing how to use tools like Large Language Models (LLMs) to create user-friendly solutions.
To develop AI products, you need to incorporate various AI technologies. Here are some examples of AI products that leverage the Unique Value Propositions (UVPs) of the companies that made them:
- Generative AI: Large Language Models (LLMs), like GPT-4 or Google’s Gemini, which are capable of understanding and generating human-like text.
- Machine learning
- Computer vision
- Robotic Process Automation
- Deep learning
By understanding these technologies and their applications, you can create AI products that meet the needs of your business and customers.
User Flow Template
A well-designed user flow template is essential for developing and implementing AI products. It breaks down a complicated process into clearly defined flows from the initial entry point to the generated output.
The AI User Flow Template is a useful tool for product managers to visualize and streamline the user experience. By applying essential steps to real-world examples, you can create a clear and intuitive flow that guides users through your product.
A good user flow template should include essential steps such as defining the initial entry point, identifying user goals, and determining the generated output. These steps help to create a clear and logical flow that meets user needs.
By applying the AI User Flow Template to real-world examples, you can create a user flow that is both efficient and effective. This can help to improve user engagement and satisfaction with your product.
Prompt Template
Developing and implementing effective AI-powered chatbots requires a solid understanding of AI prompt templates.
A well-crafted AI prompt template can help ensure quality results from natural language processing chatbots.
To engage effectively with these chatbots, you need to consider the following key points:
- Session replays can be analyzed using AI-powered products to identify patterns, pain points, and areas for improvement.
- AI can prioritize feature development by analyzing trends and sentiments in user feedback.
- Product managers can interact with data in intuitive ways without needing to run complex commands.
By using these AI-powered tools, product managers can make data-driven decisions swiftly and efficiently.
Strategy and Planning
As an AI/ML product manager, strategy and planning are crucial to the success of your product. You must have a deep understanding of both the business context and the potential of AI to drive innovation and solve specific challenges.
To develop a solid AI product strategy, you need to identify suitable AI applications and define product features that deliver tangible value to end-users. This involves translating AI capabilities into functionalities that ensure seamless integration into existing workflows.
Overseeing the model training and deployment process is also vital to ensure that AI models are accurate, reliable, scalable, and optimized for real-world performance. You should collaborate closely with data engineers to leverage data science principles such as bias mitigation, data integrity, and model interpretability.
Your AI product should build on the existing products, data, or market position of your company. This means leveraging proprietary data or subject matter expertise to create a Unique Value Proposition (UVP) that sets your product apart.
Here are some key considerations to keep in mind when developing your AI product strategy:
- Identifying suitable AI applications requires a deep understanding of both the business context and the potential of AI to drive innovation and solve specific challenges.
- Defining product features involves translating AI capabilities into functionalities that deliver tangible value to end-users while ensuring seamless integration into existing workflows.
- Overseeing the model training and deployment process is crucial to ensure that AI models are not only accurate and reliable but also scalable and optimized for real-world performance.
- Collaborating closely with data engineers is crucial for translating complex AI capabilities into user-friendly, ethical, and valuable product features.
As a product manager, you must be prepared to adapt to changes and challenges in the continuous learning process. This involves thinking outside the box and anticipating possible problems that may arise. By developing your strategic thinking and risk management skills, you can become a more effective problem solver and product manager.
Collaboration with Cross-Functional Teams
Collaboration with cross-functional teams is a crucial aspect of a product manager's role, especially when working on AI products. This complexity increases significantly, involving numerous stakeholders such as data scientists, data engineers, machine learning scientists, and business intelligence professionals.
As a product manager, you're already accustomed to working with multiple stakeholders, but AI product development requires coordination and communication with even more teams. This adds complexity to the product development process, increasing the time required for product iterations.
Effective communication within the product team is key to ensuring that everyone follows the plan and aligns with the initial product vision. Poor communication can lead to poor prioritization of tasks, inability to meet deadlines, failed product features, and unsuccessful product launches.
To streamline communication, AI can help by automating replies, tracking team work, identifying work overlap, and optimizing meetings. AI-powered knowledge management systems like Guru can also enhance information sharing.
Collaborating effectively with AI/ML teams is crucial for product managers. A key best practice is to cultivate a deep respect and understanding for each role in the AI/ML team, from data scientists to ML engineers and researchers.
Here are some common AI/ML team roles:
- Data Engineer: Manages and prepares vast amounts of data for analysis.
- Data Scientist: Uses data to derive insights and create predictive models.
- Machine Learning Engineer: Designs, implements, and deploys optimized machine learning algorithms.
- Research Scientist: Explores theoretical AI/ML aspects and works on innovative techniques.
- AI Architect: Designs the structural foundation for AI applications.
- MLOps Engineer: Focuses on efficient deployment, management, and continuous integration of ML models.
- Validation & QA Specialist: Monitors and tests AI systems for consistent performance.
- Visualization Specialist: Translates AI/ML data and findings into clear visual formats.
Product managers should invest time in regular sync-ups, encourage open communication, and involve these specialists early and often in the product development process. Co-creation workshops can be beneficial in brainstorming ideas collaboratively.
Challenges and Solutions
Developing AI/ML products can be a daunting task, but understanding the challenges can help you prepare and find solutions. Specialized knowledge is a major challenge, requiring AI Product Managers to have a deep understanding of data science to guide development.
This is because ML products need skilled data scientists and engineers, which can be hard to find and retain. Infrastructure demands are also a challenge, as ML models require significant computational resources and data storage for large-scale deployment.
To overcome these challenges, it's essential to have a solid plan in place. Here are some key considerations:
- Develop a robust understanding of data science to effectively guide development.
- Plan for significant computational resources and data storage for large-scale deployment.
- Be prepared for longer development timelines and plan accordingly.
- Address transparency issues by implementing explainable AI models.
Challenges
As an AI product manager, you'll face several challenges that can make or break your project. One of the biggest hurdles is the need for specialized knowledge. You'll need to have and maintain a deep understanding of data science to effectively guide the development of AI-powered products.
Developing ML products requires significant computational resources and data storage for large-scale deployment. This is a major infrastructure demand that can be costly and time-consuming to set up. In my experience, it's essential to plan for this from the outset to avoid costly delays later on.
ML products often have longer development timelines, requiring careful planning and patience. This can be frustrating, especially if you're used to working on traditional software projects. However, the payoff is worth it, as ML products can be incredibly powerful and valuable.
Consider reading: Ai and Ml Development Services
The complex nature of ML models can make them difficult to explain and justify. This transparency issue can be a major challenge, especially when it comes to communicating with stakeholders or justifying the cost of development.
Here are some of the key challenges you'll face as an AI product manager:
- Specialized knowledge: data science expertise
- Infrastructure demands: computational resources and data storage
- Development cycles: longer timelines and planning required
- Transparency issues: explaining and justifying ML models
Addressing Bias
Addressing bias is a critical skill for AI Product Managers. Bias occurs when machine learning algorithms produce anomalous outputs due to assumptions made during algorithmic development or biases in the training data.
A biased dataset can lead to inaccurate model outputs. For instance, a model prioritizing resumés for interview selection may have a biased dataset if it only includes data from candidates who applied and progressed through the process.
Ensuring a balanced dataset is essential to mitigate bias and improve model accuracy. This can be achieved by collecting data from a diverse range of sources and individuals.
A balanced dataset helps to reduce the risk of anomalous outputs, which can have serious consequences. For example, a biased model may incorrectly prioritize resumés based on irrelevant factors, leading to unfair hiring decisions.
For more insights, see: Energy Based Model
Becoming a Great
To become a great AI ML product manager, you don't need to be a tech expert, but it's essential to grasp AI/ML basics.
Dive into data to understand its significance in AI ML product management, and partner with data scientists to leverage their expertise.
Prioritizing user needs is crucial, as it helps you create products that meet real-world problems.
Grasping AI/ML basics will help you communicate effectively with your team and stakeholders, and make informed decisions about product development.
Dive into data to understand its significance in AI ML product management.
Tools and Resources
As a product manager, you know how overwhelming it can be to stay on top of everything. There are 21 AI tools that can make your life easier, specifically designed for product managers and their teams.
These tools can help you save time and take your AI products to the next level. You can access free templates to get started.
Product managers can make their lives easier with time-saving AI tools.
Curious to learn more? Check out: Ai Time Magazine
Best Practices and Techniques
As an AI ML product manager, it's essential to stay organized and focused on the project's goals. Prioritize tasks based on business value and technical feasibility.
To ensure a smooth product development cycle, collaborate closely with cross-functional teams, including engineering, design, and research. This collaboration will help identify potential roadblocks and opportunities for innovation.
Regularly review and update the product roadmap to reflect changes in market trends and customer needs. This will help you make data-driven decisions and stay competitive in the market.
Consider reading: Generative Artificial Intelligence Market
What Sets Apart
So, what sets apart AI/ML products from traditional software? They're unique because they evolve with the data they process, unlike traditional software, which is deterministic and based on set algorithms.
Machine learning products can deliver exceptional user experiences, providing capabilities that traditional methods can't achieve. This is often referred to as the "wow" factor.
These products adjust to changes in user behavior and conditions, maintaining their effectiveness over time. This adaptability is key to their success.
ML products can expand to serve broader audiences without losing performance. This scalability is a major advantage over traditional software.
Mastering machine learning can give a business a sustainable edge in the market. It's a competitive advantage that can be hard to match.
Applying UX Best Practices
Many AI systems influence user experiences in unconscious ways, often by leveraging user information to enhance their experience without requiring explicit input. This can be seen in search engines, where users are shown different results based on their gender information.
Consider the example of two users searching for the same word, "shoes." One user is shown men's shoes, while the other is shown women's shoes, due to the AI tailoring the results based on user information.
As a product manager, it's crucial to consider what information you use to improve the customer experience and be mindful of potential privacy concerns and ethical considerations.
To guide user actions effectively, you must design your interface to nudge users in the direction you want them to take. This involves simplifying the user interface and ensuring there are at most one or two clear actions for users to follow.
By being opinionated and aligning the interface with your desired outcomes, you can enhance the user experience and achieve your product's objectives.
A unique perspective: What Is One of the Key Challenges Faced by Genai
Marketing and Sales
As an AI ML product manager, understanding marketing and sales is crucial to the success of your product. A solid understanding of marketing and sales principles is necessary to devise and implement business objectives and strategies.
Having a good level of knowledge of how to market and sell your product is essential, especially in industries like healthcare. You'll need to study trends and new ideas, be aware of the best branding techniques, and more.
Improving your marketing skills can be done by taking marketing and sales courses, working alongside sales professionals, and utilizing online resources.
If this caught your attention, see: Ai and Ml in Digital Marketing
Marketing and Sales
To be a successful AI product manager, you need to have a solid understanding of marketing and sales skills. This involves studying trends and new ideas in the industry, being aware of the best branding techniques, and more.
Marketing and sales skills are becoming increasingly important for product managers, especially those overseeing AI products. In today's world, making incredible AI products isn't enough; you also have to sell those products and get customers interested and engaged.
To improve your marketing skills, take marketing and sales courses. This will help you learn the principles of marketing and sales and how to apply them to your AI product. You can also spend time working alongside sales professionals to gain practical experience.
Creating impactful product copy is crucial to driving adoption of your AI product. This can be done using AI-powered tools like Userpilot, which enables product and customer success teams to create quality microcopy for in-app communication and user onboarding.
To use Userpilot, simply press space in the text box of your tooltip or modal, enter your prompt, and the tool will generate content from zero. The more specific your prompt, the better the results will be.
How to Leverage SaaS
To leverage SaaS effectively, you need to understand how to use AI to your advantage as a product manager. This involves leveraging AI product management in your SaaS, as discussed earlier.
You can start by identifying areas where AI can help streamline processes and improve customer experiences. For example, using AI to personalize product recommendations can significantly boost sales.
AI can also help you gather valuable insights from customer data, enabling you to make more informed product development decisions. By leveraging AI in this way, you can create a more customer-centric product that meets their evolving needs.
To get started, you'll need to determine which AI tools and technologies are best suited for your organization and product. This may involve experimenting with different solutions and evaluating their effectiveness.
By doing so, you can unlock the full potential of AI in your SaaS and drive business growth through more efficient and effective marketing and sales strategies.
Frequently Asked Questions
Is AI Product Manager a good career?
AI Product Manager is a promising career with growing opportunities, offering a unique blend of technical and business skills. If you're interested in AI and product development, this role could be a great fit for you
How to become a ML Product Manager?
To become a successful ML Product Manager, start by building a strong foundation in machine learning fundamentals and technical skills, then develop practical experience and cross-functional communication skills. By following these steps, you'll be well-equipped to drive innovation and make informed decisions in the field of machine learning product development.
Sources
- https://productschool.com/blog/artificial-intelligence/guide-ai-product-manager
- https://producthq.org/career/ai-product-manager/ai-product-manager-skills/
- https://producthq.org/career/machine-learning-product-manager/
- https://akfpartners.com/growth-blog/ai-ml-for-product-managers
- https://userpilot.com/blog/ai-product-management/
Featured Images: pexels.com