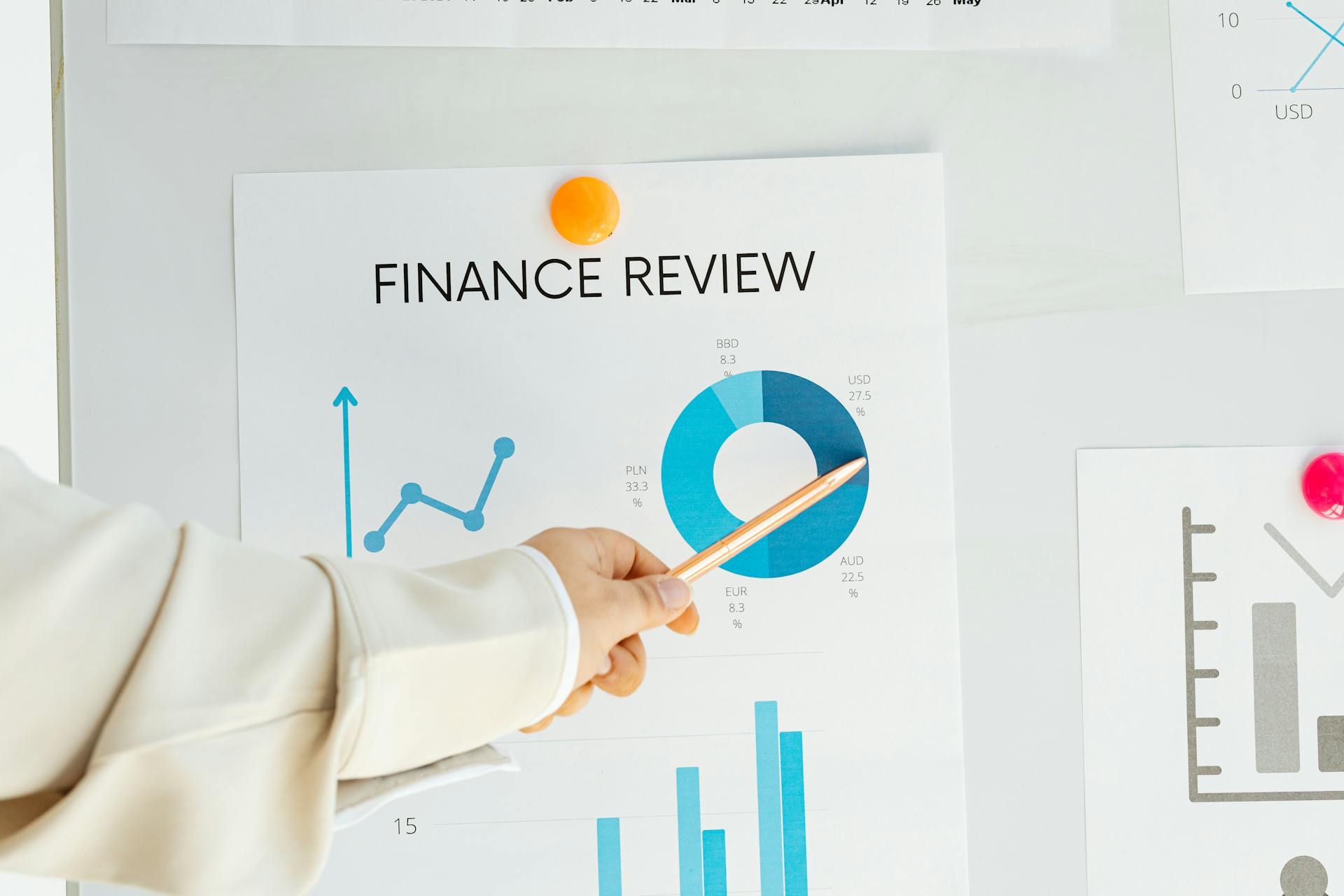
Audit AI software is a game-changer for businesses, offering unparalleled efficiency and accuracy in auditing processes.
With AI-powered audit software, you can automate routine tasks, freeing up staff to focus on high-value tasks that require human judgment.
According to recent studies, AI-powered audit software can reduce audit times by up to 90%, allowing businesses to respond faster to market changes and stay ahead of the competition.
This significant reduction in audit time also leads to cost savings, as businesses can allocate resources more effectively and reduce the need for manual labor.
What Is Audit AI Software?
Audit AI software is a type of technology that uses artificial intelligence to automate and streamline the audit process.
It can automate repetitive tasks such as data collection and analysis, freeing up auditors to focus on higher-level tasks like risk assessment and decision-making.
Audit AI software can analyze large amounts of data quickly and accurately, reducing the time and effort required to complete an audit.
Related reading: Software for Ai Data Analysis Free
This can lead to more efficient and effective audits, as well as improved accuracy and reduced risk of human error.
Some audit AI software uses machine learning algorithms to identify patterns and anomalies in data, allowing auditors to focus on areas of high risk.
By automating routine tasks and providing auditors with more time to focus on high-level tasks, audit AI software can help organizations reduce the cost and time required to complete an audit.
Benefits and Limitations
Audit AI software offers numerous benefits, including risk mitigation and increased reliability. Neglecting AI audit initiatives can impose different risks on your AI ventures.
AI audit is crucial for organizations to attain legal compliance and address algorithmic bias. Meeting different regulatory compliances while remaining profitable is a challenge many businesses face.
Industry leaders recognize the importance of AI auditing, with Hired's CTO, Dave Walters, stating that the company already intended to audit its systems for fairness and transparency in 2022. This highlights the growing awareness of the need for AI auditing.
Some benefits of AI auditing include:
- Risk mitigation
- Attaining legal compliance
- Addressing algorithmic bias
- Increased reliability
- Accountability
Benefits of
The benefits of AI auditing are numerous and significant. It's a new field, but it's gaining momentum in the business world.
Risk mitigation is one of the key benefits of AI auditing. By identifying and addressing potential risks, organizations can protect themselves from costly mistakes.
Attaining legal compliance is another crucial benefit. AI auditing helps organizations meet regulatory requirements and avoid fines or penalties.
Algorithmic bias is a significant issue that AI auditing can help address. By examining AI systems, organizations can identify and correct biases that may be discriminatory or unfair.
Increased reliability is also a benefit of AI auditing. By testing and validating AI systems, organizations can ensure that they are functioning as intended.
Accountability is another key benefit. AI auditing helps organizations take responsibility for their AI systems and ensure that they are transparent and fair.
Here are the benefits of AI auditing in a nutshell:
- Risk mitigation
- Attaining legal compliance
- Addressing algorithmic bias
- Increased reliability
- Accountability
These benefits are essential for organizations looking to build sustainable AI systems with better accountability, trust, and compliance.
Limitations of
Algorithm bias can be a significant limitation of AI. Biases in the training data or biased assumptions made during the algorithm development process can cause an aberration in the output of machine learning algorithms.
This can lead to worse outcomes than human biases, as AI may not have the same self-awareness to recognize and correct its mistakes. Biases in society have a significant impact on algorithmic AI bias.
AI may not always be able to recognize and correct its own biases, making it a limitation of its ability to make fair and unbiased decisions.
Reduce Bias
Reducing bias in AI systems is crucial to ensure fairness and equality in decision-making processes. AI audits aim to reduce AI bias by ensuring an AI system's algorithms are unbiased and do not discriminate against any entity or group.
Biases in AI systems can arise from biased assumptions made during the algorithm development process or from the training data used. Our mind has blind spots or preconceived notions based on the standards and values of our culture, which can be reflected in algorithmic AI bias.
To mitigate algorithmic bias, organizations should prioritize AI auditing implementation to build sustainable AI systems with better accountability, trust, and compliance. AI audits help understand KPIs associated with AI technology and can identify potential biases in AI systems.
Here are some key benefits of reducing bias in AI systems:
- Risk mitigation
- Attaining legal compliance
- Addressing algorithmic bias
- Increased reliability
- Accountability
By reducing bias in AI systems, organizations can build trust with their customers and stakeholders, and ensure that their AI systems are fair and transparent.
Functionality and Impact
AI audit software is revolutionizing the way auditors work. It significantly increases efficiency and precision, allowing them to scrutinize vast amounts of data across various systems and processes.
For auditors, AI tools are adept at uncovering risks and anomalies that might elude manual reviews. This is especially useful when dealing with complex financial statements and IT infrastructure.
AI auditing tools also play a crucial role in preserving the integrity of financial and operational data. They ensure ongoing transparency and assist in maintaining compliance with evolving regulations. This safeguards against financial discrepancies and fraud.
Here's a summary of the benefits of AI audit software for both auditors and companies:
- For auditors: increased efficiency, precision, and ability to uncover risks and anomalies
- For companies: preservation of financial and operational data integrity, ongoing transparency, and compliance with regulations
Functionality and Impact
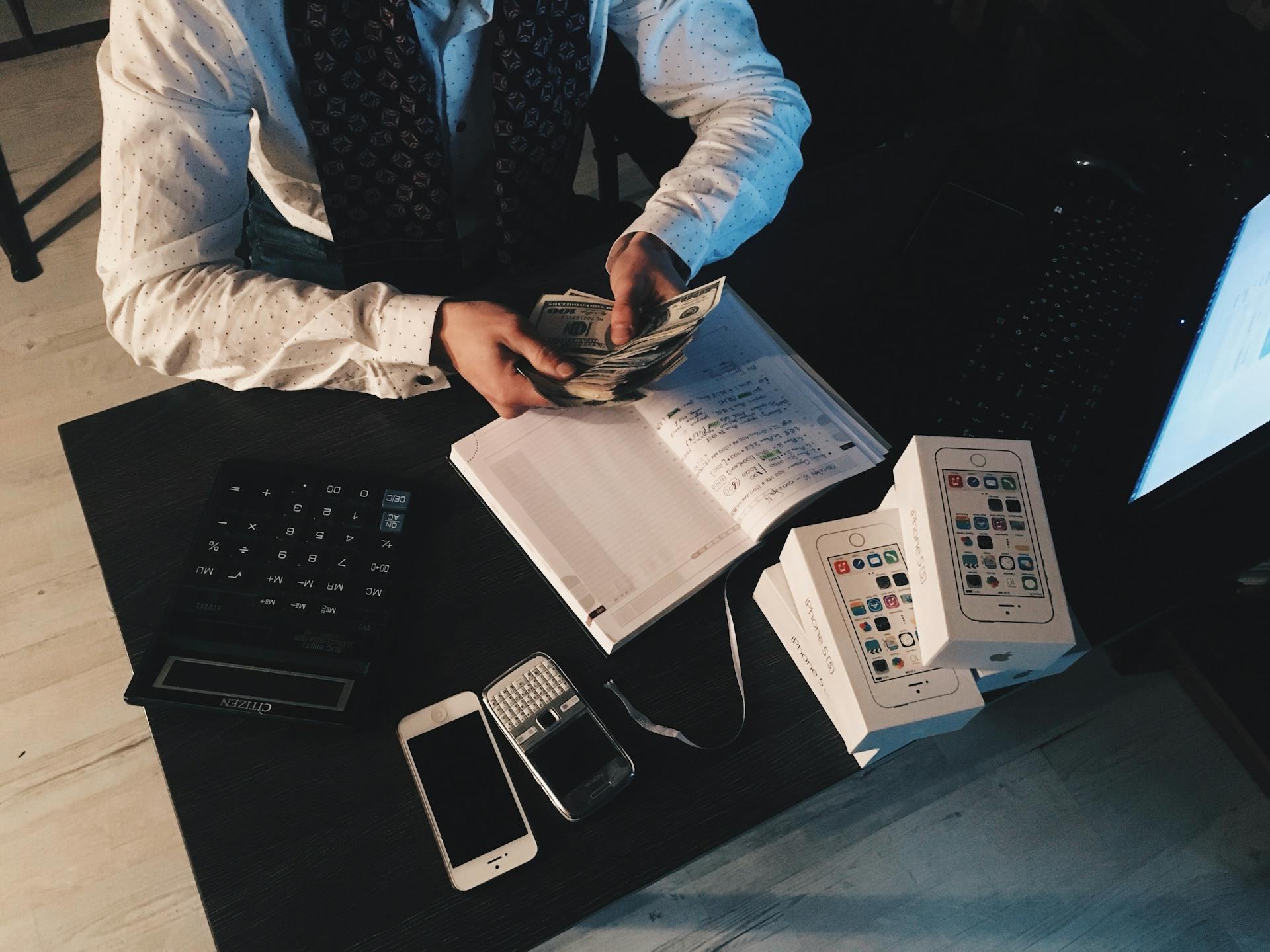
AI auditing tools are revolutionizing the way auditors and finance teams work. They significantly increase the efficiency and precision of audits by scrutinizing vast amounts of data across various systems and processes.
For auditors, AI tools are adept at uncovering risks and anomalies that might elude manual reviews. They can scrutinize financial statements and IT infrastructure with ease.
These tools play a crucial role in preserving the integrity of financial and operational data. They ensure ongoing transparency and assist in maintaining compliance with evolving regulations.
AI auditing tools help safeguard against financial discrepancies and fraud by providing critical support in navigating the complexities of today's financial ecosystems. They automate routine tasks, detect patterns in large datasets, and identify anomalies.
For companies, AI auditing tools are essential in maintaining compliance with regulations and preserving the integrity of financial data. They assist in ongoing transparency and risk management.
By leveraging artificial intelligence, AI auditing tools enhance the auditing process, making it more efficient and effective. They provide critical support to auditors and finance teams alike.
Data Preprocessing
Data preprocessing is a crucial step in the AI development process. It's all about getting your data in the right shape for your AI model to learn from.
Data imputation methods are used to handle missing values. This is especially important when working with real-world data, which often has gaps or inconsistencies.
Methods like random stratification and cross-validation are applied to select training and test datasets. This ensures that your AI model is trained and tested on a diverse range of data.
Data normalization and standardization are also essential steps in data preprocessing. This helps to prevent features with large ranges from dominating the model.
Here are some common data preprocessing methods:
- Data imputation methods used to handle missing values
- Methods applied to select training and test datasets such as random stratification, or cross-validation
- Methods used to normalize and standardize data
These methods can make a big difference in the performance of your AI model. By taking the time to properly preprocess your data, you can ensure that your model is learning from the best possible information.
ML Modeling
ML modeling is a crucial aspect of AI development, and it's essential to understand the techniques and considerations involved.
To address a given problem, other ML techniques can be explored, such as those listed: What other ML techniques address the given problem?What are the outcomes?Reasons to justify the chosen AI strategy.
ML algorithms must be refined using specific criteria and assumptions, which can be a complex process.
Criteria used to refine ML algorithms include the problem being addressed, the data available, and the desired outcomes.
Existing and ready-to-use packages can be used to code ML algorithms, but sometimes coding from scratch is necessary.
Ultimately, the chosen AI strategy should be justified based on the specific problem being addressed and the desired outcomes.
Model Monitoring in Machine Learning
Model monitoring in machine learning is crucial for ensuring the accuracy and reliability of AI systems. It involves monitoring the performance of machine learning models, detecting potential biases and errors, and making necessary adjustments to maintain their effectiveness.
ML monitoring is essential to prevent model drift, where the model's performance degrades over time due to changes in data or algorithms. This can lead to incorrect predictions and decisions, which can have serious consequences.
A well-designed ML monitoring process should include regular checks on model performance, data quality, and algorithm updates. This can be achieved through techniques such as data validation, model validation, and model retraining.
Some key considerations for ML monitoring include:
- Monitoring model performance and detecting potential biases and errors
- Regularly checking data quality and updating algorithms as needed
- Ensuring compliance with laws and regulatory standards
- Aligning with organizational goals and demonstrating ethical and social responsibility
By implementing effective ML monitoring, organizations can ensure that their AI systems are accurate, reliable, and trustworthy, and that they are making informed decisions based on high-quality data and models.
A Path to Trust
Transparency and explainability are key to building trust in AI systems. Utilizing AI tools with explainable AI (XAI) principles is crucial to overcoming the "black box" nature of some AI solutions.
Auditing AI systems is essential for fostering users' trust and confidence in AI. A fair, responsible, and auditable AI system is a hallmark of trustworthy AI.
Data privacy is a top concern for consumers, and businesses must prioritize it. Defining data access procedures and permissions constitute a better practice for data privacy compliance.
Implementing privacy standards, protecting consumer rights and interests, and adhering to legal aspects around data usage are all critical components of data privacy.
Strategy and Planning
To create a successful AI strategy, you need a clear understanding of your business objectives and how AI can help you achieve them. This involves confirming that your AI strategy aligns with your desired business goals and justifies your AI expenditures.
A unique perspective: Ai Business Software
A thorough AI audit plan is essential to prevent different failures and help your business grow by reaping the benefits of AI capabilities. This includes exploring more opportunities, market growth, smoother operations, and a competitive advantage.
To start auditing AI, you should define the scope of the audit and identify the AI system(s) under examination. This will help you build a strategy that fosters communication with various subject matter experts.
Planning a Strategy
Planning a strategy for AI adoption is crucial for businesses to achieve their desired objectives. A clear understanding of business objectives and AI's potential to achieve those is necessary.
Decision-makers should confirm that their AI strategy is aligned with desired business goals and justifies AI expenditures. This involves conducting an AI audit plan to check AI-driven business outcomes and preventing potential failures.
A thorough AI audit plan will help the business grow by reaping the benefits of AI capabilities to explore more opportunities, market growth, smoother operations, and competitive advantage. David Yakobovitch mentions this in his LinkedIn article, where he interviewed Veljko Krunic, the author of AI, on the HumAIn Podcast.
Krunic highlighted that businesses must act on the results of the AI technology, and executives must find out the benefits AI brings to the table.
To define the scope of an AI audit, consider the following:
- Clearly define the scope of the audit.
- Identify the AI system(s) under examination.
Communication is also key in planning a strategy. Build a strategy that fosters communication with various subject matter experts to ensure a successful AI audit.
Get Value with Centraleyes for Free
You can start getting value with Centraleyes for free, and see how it exceeds traditional GRC systems.
The Centraleyes platform eliminates the need for manual processes and spreadsheets.
You can run a full risk assessment in less than 30 days, giving you immediate value.
Centraleyes is free for 30 days, allowing you to try it out and see its benefits firsthand.
If this caught your attention, see: Ai Voice Software Free Voice Changer
Best Practices and Roadmap
To audit AI software effectively, you need to adopt the right AI auditing framework and practices. This involves accepting, using, and adapting AI auditing framework and regulations.
To ensure a smooth auditing process, it's essential to have a transparent communication system with stakeholders. This includes ensuring that all stakeholders are informed and involved in the process.
To finalize the scope of the AI system, you need to implement well-informed practices about application design and architecture. This will help you understand what needs to be audited and how to do it.
Transparency is key in the iterative development process of AI systems. This means that changes should be tracked and documented to ensure that the system remains secure and compliant.
To maintain control and governance over the AI system, you need to ensure that all stakeholders are on the same page. This includes involving domain experts and specialists, as needed, and ensuring cross-team transparency with documentation and communication.
Here are some best practices for successful AI auditing:
- Accept, use, and adapt AI auditing framework and regulations
- Ensure a transparent communication system with stakeholders
- Implement well-informed practices about application design and architecture to finalize its scope
- Ensure transparency in the iterative development process
- Ensure control and governance
- Include all stakeholders
- Involve domain experts and specialists, as needed
- Ensure cross-team transparency with documentation and communication
One effective practice of accommodating ML system changes is complete ML pipeline monitoring. This automates tracking the performance of production ML models and the entire ML pipeline, making it easier to set up and configure monitors to track drift, data quality issues, and performance metrics.
Addressing Challenges
Auditing AI systems can be a complex task, and many organizations face challenges in this area. The $71,000 Innovation Challenge aims to design and create applied tools that can assess whether deployed AI systems exhibit bias or carry potential for discrimination.
To overcome these challenges, many AI auditing frameworks have been defined by global organizations. These frameworks can help you audit AI-enabled initiatives.
The auditing process checks the AI system's compliance with regulatory, ethical, and governance requirements, helping mitigate business risks posed by AI applications.
Integration Challenges
Integration challenges can arise when AI audit tools operate in isolation from the broader enterprise systems, complicating their integration into existing workflows.
Successful adoption of AI audit tools requires them to be fully compatible with an organization's data ecosystem, ensuring a smooth flow from data collection through to analysis and reporting.
This compatibility is crucial for effective integration, as AI audit tools often need to interact with various systems and tools to function properly.
Challenges and Roadmap
The challenges in auditing AI are numerous and complex. One of the main issues is the lack of mature or nonexistent frameworks specific to AI audits.
There are limited precedents and historical context to draw from, making it difficult to establish a clear understanding of what constitutes a successful AI audit. This ambiguity surrounding the definition of AI also adds to the complexity.
The highly dynamic nature of AI means that auditors must be able to adapt quickly to new technologies and methodologies. This steep learning curve for AI auditors can be a significant barrier to entry.
To overcome these challenges, many global organizations have defined AI auditing frameworks that can help guide the process.
Risk Mitigation
Auditing AI systems is crucial to mitigate business risks posed by AI applications. This process helps predict potential unforeseen risks and strategize risk mitigation plans.
The auditing process checks the AI system's compliance with regulatory, ethical, and governance requirements. This systematic approach and documentation foster confidence and trust in the AI system.
Related reading: Generative Ai Risks
AI audits aim to reduce AI bias by ensuring that an AI system's algorithms are unbiased and do not discriminate against any entity or group. This is a key objective of AI audits.
Biases in AI systems can be caused by biased assumptions made during the algorithm development process or biased training data. These biases can lead to aberrations in the output of machine learning algorithms.
Auditing AI systems helps mitigate business risks by identifying potential biases and developing strategies to address them. This is essential for building trust in AI systems and ensuring their safe and responsible deployment.
Challenge Details
The $71,000 Innovation Challenge is a call to action for designing better AI audits. The challenge aims to create applied tools that can assess whether deployed AI systems exhibit bias or carry potential for discrimination.
The challenge invites teams to submit models, solutions, datasets, and tools to improve people's ability to audit AI systems for illegal discrimination. Submissions can either assess commercially deployed AI systems or open source AI systems that are known to be used within industry.
Related reading: Claude Ai Tool
Entrants will be evaluated by a jury, with points awarded for each of the following criteria: insights, alignment, impact, ease of use, scalability, replicability, documentation, and sustainability. This evaluation process ensures that the winning submissions demonstrate practical and effective solutions for AI auditing.
The challenge is open to any legal entity, including natural persons or groups of legal entities, except public administrations. Applications were open from July 11 to October 10, 2022, 11:59 pm, Pacific Daylight Time.
The evaluation criteria can be broken down as follows:
- Insights: What did we learn using the tool?
- Alignment: How well anchored is the audit with legal and policy needs?
- Impact: How many people would benefit from the tool?
- Ease of use: Is the tool usable for our target audience?
- Scalability: Can the tool be used at scale and/or used in different contexts?
- Replicability: Can the results be replicated by other users using the same systems?
- Documentation: How well-explained are the findings?
- Sustainability: Is the tool financially and environmentally sustainable?
Compliance and Oversight
Advanced AI auditing solutions are not just about detecting anomalies, but also about ensuring compliance with legal requirements and protocols. Modern AI applications are helping grow businesses with new opportunities, but each country and continent has its well-defined legal framework and regulations to measure damages stemming from AI-based decision-making.
To assure compliance of AI-driven models with legal requirements, AI audits offer a way to measure up. This involves embedding continuous auditing deeply within a company’s own processes, promoting a more integrated, continuous feedback loop within daily operations.
Here's an interesting read: What Is Blue J Legal Ai Software
AI systems are also prone to security attacks and data compromise, making security controls a top priority. Compliance with external security certifications, subscribing to security advisories, and implementing model governance policy are just a few ways to strengthen AI security posture.
Here are some essential security controls to consider:
- Compliance with external security certifications
- Subscribe to security advisories to receive alerts
- Code review by the team, and at least one of the reviewers is not the author of the code
- Implement model governance policy
- Document policies-processes and contracts for dealing with third parties, breach reporting, and escalation.
- Staff training to understand breach reporting policy and procedures
- Track changes made to AI system design, broader analysis of complaints, and justification to reduce the risk of the next attack
Legal Compliance
Legal compliance is crucial for businesses using AI applications, as each country and continent has its own well-defined legal framework and regulations to measure damages stemming from AI-based decision-making.
AI audits offer a way to assure compliance of AI-driven models with legal requirements and specified protocols, helping businesses avoid potential legal issues.
To ensure compliance, it's essential to understand the legal framework and regulations in your region, and to regularly review and update your AI systems to ensure they meet these requirements.
Here are some key steps to take:
- Regularly review and update your AI systems to ensure they meet legal requirements and specified protocols.
- Conduct AI audits to assure compliance of AI-driven models with legal requirements and specified protocols.
By prioritizing legal compliance, businesses can avoid potential legal issues and ensure that their AI applications are used responsibly and in accordance with the law.
Financial Oversight Tools
Financial Oversight Tools are revolutionizing the way companies approach auditing. Advanced AI financial auditing solutions are designed for deep integration with a company's financial systems and learn from unique company data, offering insights and assessments highly relevant to each organization's operations.
With advanced AI financial auditing solutions, continuous and real-time data monitoring becomes possible, allowing for proactive anomaly detection and resolution. This means no more periodic data reviews, but rather an ongoing financial co-pilot that scrutinizes financial transactions and provides immediate feedback on data accuracy and consistency.
Internal integration and company-centric auditing are key features of these advanced systems, which advocate for embedding continuous auditing deeply within a company's own processes. This approach promotes a more integrated, continuous feedback loop within daily operations, reducing reliance on external audit services.
Advanced AI solutions also help distribute the auditing workload more evenly across time, alleviating the end-of-period rush that can lead to oversights. By enabling real-time transaction analysis, they support a more balanced and diligent auditing process throughout the month.
Here are some key benefits of advanced AI financial auditing solutions:
- Tailored insights and personalization
- Continuous and real-time data monitoring
- Internal integration and company-centric auditing
- Distributing workload evenly over time
- Leveraging cross-company data for broader insights
Understanding and Evaluation
More than 50% of organizations are already using AI in their operations. This highlights the growing importance of auditing AI solutions.
According to KPMG's poll, 45% of respondents planned to perform an audit of their AI solutions. This suggests that many organizations recognize the need for regular assessments of their AI systems.
In fact, 90% of respondents agreed that AI applications should be subjected to internal audits. This shows a clear understanding of the importance of auditing AI solutions to ensure they are functioning as intended.
Organizations are still unsure about their AI audit approach, with 70% of respondents agreeing they were not clear on what their AI audit approach should be. This highlights the need for guidance and best practices in auditing AI software.
Data Dependency and Learning
AI tools are only as good as the data they're trained on, and not all organizations have the clean, structured data these tools require.
Data preprocessing is a crucial step in getting AI models to work effectively, involving methods like data imputation to handle missing values, and normalization to standardize data.
AI models can be resource-intensive to set up and train, necessitating a commitment to training these systems on specific organizational data and processes.
Here are some data preprocessing methods that can be used:
- Data imputation methods used to handle missing values
- Methods applied to select training and test datasets such as random stratification, or cross-validation
- Methods used to normalize and standardize data
In some cases, AI models may not be able to learn and improve their accuracy without extensive datasets, potentially limiting their effectiveness.
What Sets This Project Apart From FAccT?
This project stands out from conferences like ACM FAccT in its focus on directly funding the use and demonstration of technical tools for auditing publicly deployed systems and open source models.
The challenge targets risk areas such as bias and illegal discrimination, which are significant concerns in the tech industry.
For instance, word embeddings used by language tools like Gmail's SmartReply and Google Translate often classify terms as being inherently closer to males or females, highlighting the need for more nuanced auditing tools.
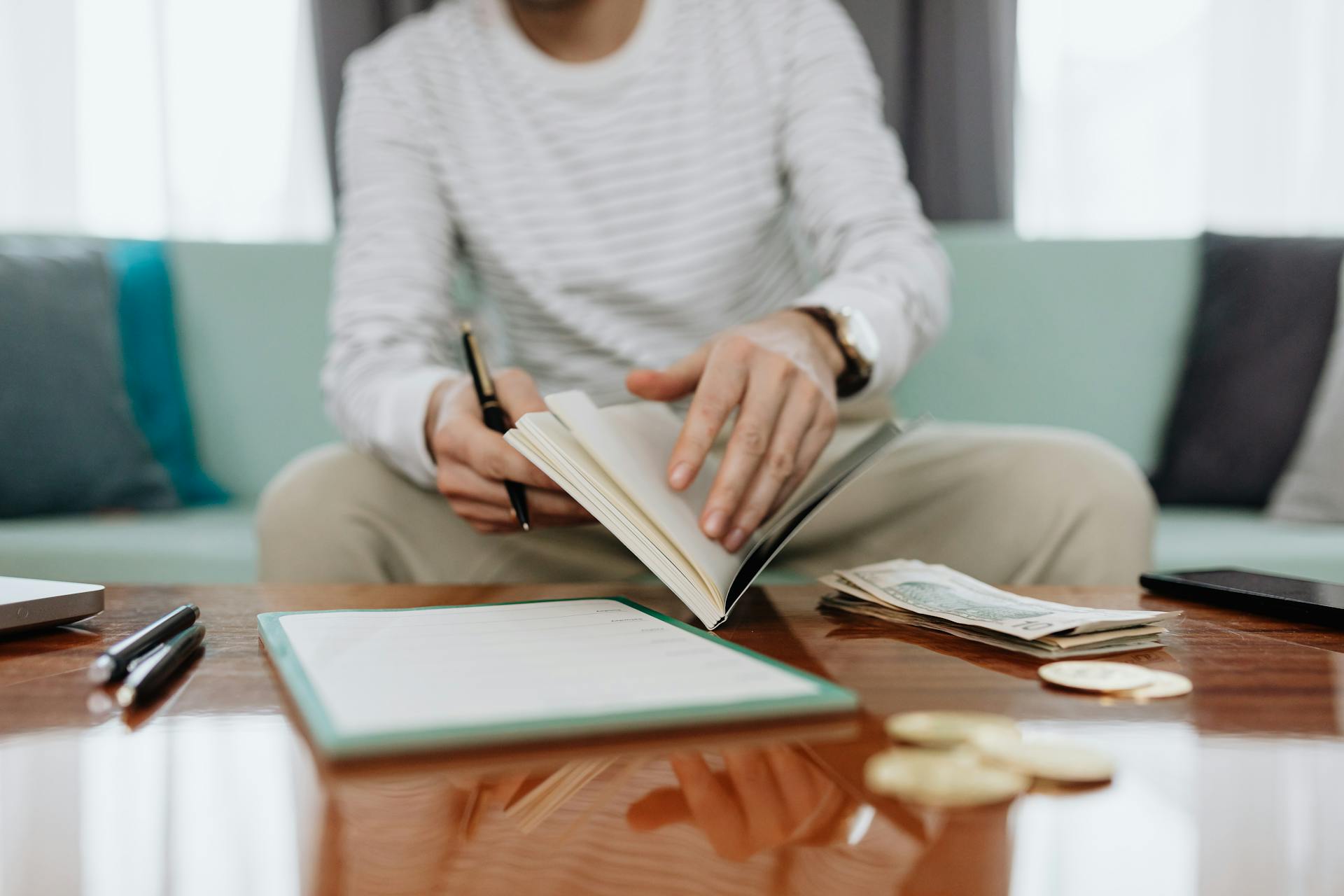
The project's focus on developing software, code, and tools that allow people to test publicly available algorithms and deployed models for bias and discrimination is a key differentiator.
These tools are designed to be useful and actionable for regulators, civil society, and journalists, who are often on the frontlines of addressing these issues.
Here are some examples of the types of biases and discriminatory practices that these tools aim to detect:
- Word embeddings classifying "football" as being closer to males and "receptionist" as being closer to females.
- Emotion recognition tools from Microsoft and Face consistently interpreting images of Black people as being angrier than white players, even controlling for their degree of smiling.
- Computer vision software from Amazon, Microsoft, and IBM performing significantly worse on people of color.
Frequently Asked Questions
Which AI is best for auditing?
For auditing, MindBridge AI Auditor and AutoEntry are top AI tools, offering advanced features to streamline audit processes and improve accuracy.
How is AI used in auditing?
AI in auditing helps auditors refine their scope and planning by analyzing data and identifying high-risk areas. It can also transform tasks like journal entry testing, making audits more efficient and effective.
Sources
- https://safebooks.ai/resources/financial-audit/how-ai-audit-tools-are-changing-the-financial-landscape/
- https://www.centraleyes.com/glossary/ai-auditing/
- https://censius.ai/blogs/ai-audit-guide
- https://hai.stanford.edu/policy/ai-audit-challenge
- https://blog.cloudflare.com/cloudflare-ai-audit-control-ai-content-crawlers
Featured Images: pexels.com