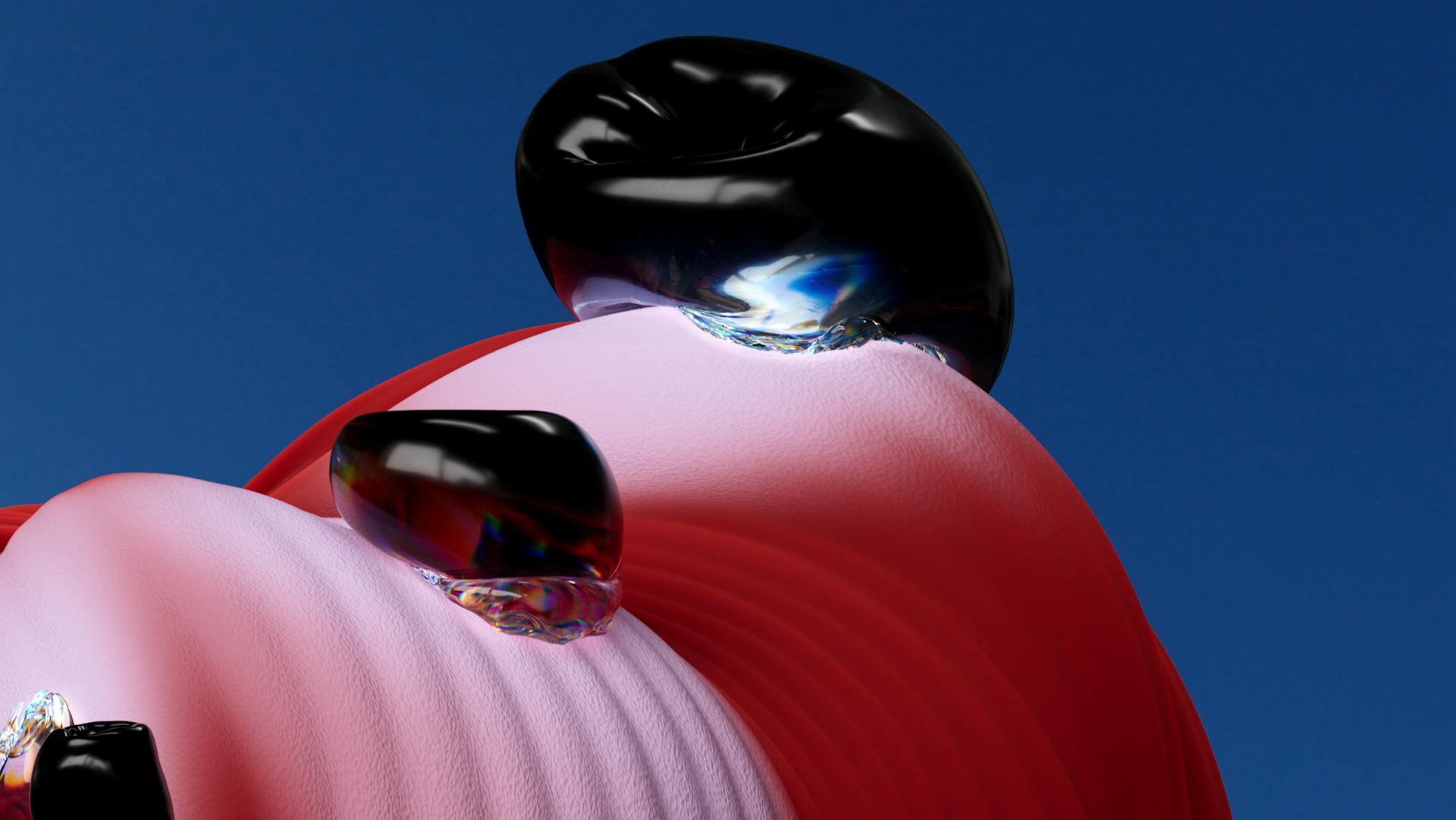
As AI and machine learning continue to advance, we're faced with a pressing question: how do we balance progress with responsibility? The truth is, AI systems are only as good as the data they're trained on, and biased data can lead to biased outcomes.
The consequences of unchecked AI development are already being felt, with AI systems perpetuating existing social inequalities. For instance, facial recognition technology has been shown to be less accurate for people of color, leading to misidentification and potential harm.
To mitigate these risks, developers must prioritize transparency and accountability in their AI systems. This includes providing clear explanations of how AI decisions are made and being open to feedback and criticism.
Ultimately, the future of AI depends on our ability to navigate these complex issues and create systems that benefit everyone, not just a select few.
See what others are reading: Data Labeling in Machine Learning with Python
Ethics in AI and ML
Ethics in AI and ML is a crucial aspect of developing and using machine learning systems. Transparency and accountability are essential to ensure that ML systems are fair, unbiased, and respect users' rights.
ML systems often operate in a "black box", making it difficult to understand how they work and how they make decisions. This lack of transparency can lead to accountability issues, as it's hard to determine who is responsible for errors or harm caused by the system.
The UNESCO Recommendation on the Ethics of Artificial Intelligence provides a framework for ethical values and principles in AI development. These principles include autonomy, fairness, respect for human rights, and transparency. ML actors should adhere to these principles to ensure that their systems are fair, unbiased, and respect users' rights.
Fairness is a key aspect of ethics in AI and ML. ML actors should minimize and avoid reinforcing or perpetuating bias and discrimination, particularly against vulnerable and historically marginalized groups. This includes bias based on gender, race, age, and other factors.
Here are some key principles to keep in mind:
- Transparency: ML systems should be transparent in their decision-making processes and provide explanations for their actions.
- Accountability: ML actors should be accountable for the actions of their systems and take responsibility for errors or harm caused.
- Fairness: ML actors should strive to create systems that are fair and unbiased, and avoid perpetuating discrimination or bias.
- Respect for human rights: ML actors should respect users' rights and ensure that their systems do not infringe on these rights.
By following these principles, we can create ML systems that are fair, unbiased, and respect users' rights.
Transparency
Transparency is a crucial aspect of AI and ML, and it's not just about being honest, it's about being accountable. Transparency in AI and ML involves providing clear and understandable explanations of how decisions are made and why certain outcomes occur.
Researchers are working to develop explainable AI, which helps characterize a model's fairness, accuracy, and potential bias. This is particularly important in critical domains like healthcare and autonomous vehicles, where transparency is vital to ensure accountability.
In healthcare, the use of complex AI methods often results in models described as "black-boxes" due to the difficulty in understanding how they work. The decisions made by such models can be hard to interpret, as it's challenging to analyze how input data is transformed into output.
Transparency is about users and stakeholders having access to the information they need to make informed decisions about ML. It's a holistic concept, covering both ML models themselves and the process or pipeline by which they go from inception to use.
See what others are reading: Machine Learning Healthcare Applications
Three key components of transparency in ML are:
- Traceability: Those who develop or deploy machine learning systems should clearly document their goals, definitions, design choices, and assumptions.
- Communication: Those who develop or deploy machine learning systems should be open about the ways they use machine learning technology and about its limitations.
- Intelligibility: Stakeholders of machine learning systems should be able to understand and monitor the behavior of those systems to the extent necessary to achieve their goals.
The lack of transparency in AI and ML systems is often referred to as the "black-box problem", which is particularly prevalent with more complex ML approaches such as neural networks.
Robot Rights
Robot rights is a concept that suggests people should have moral obligations towards their machines, similar to human rights or animal rights. This idea has been explored by the Institute for the Future and the U.K. Department of Trade and Industry.
The notion of robot rights raises questions about whether machines should have a right to exist and perform their intended functions. Some argue that this could be linked to a duty to serve humanity, similar to human rights being linked to human duties.
In 2017, the android Sophia was granted citizenship in Saudi Arabia, but this move was seen by some as a publicity stunt rather than a meaningful legal recognition. The gesture was also criticized for potentially denigrating human rights and the rule of law.
If this caught your attention, see: Human in the Loop Approach
The philosophy of sentientism grants moral consideration to sentient beings, including humans and many non-human animals. If artificial or alien intelligence demonstrates sentience, this philosophy suggests they should be treated with compassion and granted rights.
Joanna Bryson has argued that creating AI that requires rights is both unnecessary and unethical, as it would impose a burden on both the AI agents and human society.
Social Implications
Fake news and misinformation are a significant concern in today's digital age, with AI algorithms being exploited to spread false information and manipulate public opinion.
Technologies like deepfakes, capable of generating realistic yet fabricated audiovisual content, pose a risk to election interference and political stability.
Job Displacement
Job displacement is a pressing concern as AI automation advances, potentially replacing human jobs and exacerbating economic inequalities.
The potential for job displacement is significant, with AI having the potential to replace jobs that were previously held by humans.
However, some experts argue that while AI may replace knowledge workers, it also has the potential to create far more jobs than it destroys.
Social Manipulation
Social Manipulation is a serious issue that can have far-reaching consequences. Fake news, misinformation, and disinformation are commonplace in politics and business.
AI algorithms can be exploited to spread misinformation and manipulate public opinion. This can lead to social divisions and even election interference.
Deepfakes, which can generate realistic yet fabricated audiovisual content, pose a significant risk to election stability. These technologies are a major concern in the fight against misinformation.
Vigilance and countermeasures are required to address this challenge effectively. We need to be aware of the risks and take steps to prevent the spread of misinformation.
Privacy
Privacy is a major concern in AI and machine learning, as it often relies on large volumes of personal data. This can lead to issues like discrimination and repression of certain ethnic groups, as seen in China's use of facial recognition technology for surveillance.
AI systems can undermine privacy without users' knowledge or consent, either through explicit surveillance or as a byproduct of intended use. For instance, a system with access to a user's video camera can potentially infringe on their privacy.
Data collection, storage, and utilization are critical aspects of AI that require robust safeguards against data breaches and unauthorized access. This includes protecting sensitive information from extensive surveillance. China's extensive surveillance network is a prime example of this.
Large language models can "leak" personal data, and even legitimate data collection can be compromised through reverse engineering or inference-style attacks. These attacks can de-anonymize model training data, violating users' privacy.
The accuracy of AI predictions can also pose risks, such as identifying, fingerprinting, or correlating user activity. Furthermore, using AI to infer sensitive personal data from non-sensitive data, like inferring sexuality from content preferences, is a significant privacy concern.
Jurisdictions like the EU provide a "right to be forgotten", which could include being removed from ML training data. This highlights the need for ML systems to ensure that users' data is protected throughout the life cycle of the application.
ML actors should be accountable for protecting users' data and conduct adequate privacy impact assessments. They should also implement privacy by design approaches to ensure that data is collected, used, shared, archived, and deleted in ways consistent with local and international law.
You might like: Machine Learning Data Labeling
Machine Learning Issues
Machine learning issues are a significant concern in AI and machine learning. The application of machine learning can lead to harms and raise ethical questions.
Bias is a major issue in AI systems, and it can creep in through biased real-world data and algorithm design. External factors, such as biased third-party AI systems, can also influence the AI building process.
Biased real-world data is a significant problem, as it transfers the existing bias in humans to the AI system. For example, real-world data may not include fair scenarios for all population groups, leading to skewed results.
For your interest: Data Labeling in Machine Learning with Python Pdf
System Vulnerabilities
Machine learning systems are not immune to vulnerabilities, and understanding these weaknesses is crucial for their safe and responsible development.
Data input is a critical entry point for bias, which can seep into AI systems from biased real-world data.
Algorithm design is another key area where bias can creep in, often due to a lack of detailed guidance or frameworks for bias identification.
Curious to learn more? Check out: A Survey on Bias and Fairness in Machine Learning
External factors, such as biased third-party AI systems, can also influence the AI building process, but these are beyond an organization's control.
System vulnerabilities can lead to harms, raising ethical questions about the application of machine learning.
Machine learning systems are not foolproof, and their potential benefits must be weighed against their potential risks.
Accuracy
Accuracy is a good thing, and high accuracy is generally a desirable outcome in machine learning models. However, it's not always that simple.
In areas like facial recognition, high accuracy can lead to risks to privacy and autonomy, such as mass surveillance. This is a trade-off that developers must consider.
Increasing accuracy in credit-scoring or loan approval might require access to too much personal data. This raises concerns about the balance between accuracy and data protection.
Accuracy may be a useful measure in areas with clear, objective ground truth, like vehicle license-plate recognition. But in areas of human judgment, accuracy can be too reductive a measure, neglecting the nuances and complexities of real-world situations.
False positives and false negatives can have different impacts in different contexts. For example, false positives in cancer detection can lead to additional lab work, while false negatives can delay treatment.
What matters most is lowering the risk of false positives in sensitive areas like the judicial system, where sending innocents behind bars is a serious concern.
If this caught your attention, see: Is Transfer Learning Different than Deep Learning
Web ML
Web ML is a crucial aspect of machine learning, and it's essential to understand the ethical principles that guide its development and implementation.
The UNESCO Recommendation on the Ethics of Artificial Intelligence provides a set of high-level values and more detailed principles that are being adopted in the context of Web Machine Learning.
These values and principles were developed through a global, multi-stakeholder process and have been ratified by 193 countries.
The four high-level values include guidance on how to interpret them in the W3C web machine learning context.
Here are the four high-level values:
- Value 1: Respect for human rights and dignity
- Value 2: Human well-being and safety
- Value 3: Inclusivity, diversity, and non-discrimination
- Value 4: Transparency and explainability
The UNESCO principles also include an additional explicit principle of 'Autonomy' which is being added to the existing principles.
The UNESCO principles should drive the development, implementation, and adoption of specifications for Web Machine Learning.
The next section provides further guidance on how to operationalize the principles and turn them into specific risks and mitigations.
Prepare Balanced Data
Preparing a balanced data set is crucial to avoid bias in machine learning models. This involves addressing sensitive data features such as gender and ethnicity, and related correlations.
Sensitive data features like gender and ethnicity can drive bias in AI systems, as seen in Example 3, where residential areas may be dominated by certain ethnic groups. If an AI system tasked with approving loan applications makes decisions based on residential areas, the results can be biased.
To prepare a balanced data set, it's essential to have a representative number of items from all groups of the population. For instance, if your data set has too many examples from a particular ethnic group, it may skew the results.
Appropriate data-labeling methods are also crucial in preparing a balanced data set. This involves carefully labeling the data to ensure that it accurately reflects the real world.
Different weights can be applied to data items as needed to balance the data set. This is a deliberate effort to ensure that no single group dominates the data.
Here are some key considerations for preparing a balanced data set:
- Sensitive data features such as gender and ethnicity, and related correlations are addressed.
- The data are representative for all groups of the population in terms of number of items.
- Appropriate data-labeling methods are used.
- Different weights are applied to data items as needed to balance the data set.
- Data sets and collection methods are independently reviewed for bias before use.
Governance and Policy
Governance and policy play a crucial role in ensuring the responsible development and deployment of AI and machine learning technologies. Many organizations are working together to establish guidelines and regulations for the use of AI.
The Partnership on AI to Benefit People and Society is a non-profit organization formed by Amazon, Google, Facebook, IBM, and Microsoft to develop best practices for AI technologies. Apple joined the partnership in 2017.
The IEEE has also established a Global Initiative on Ethics of Autonomous and Intelligent Systems to create guidelines for the development and use of autonomous systems. The Foundation for Responsible Robotics is dedicated to promoting moral behavior and responsible robot design and use.
Governmental initiatives are also underway to ensure AI is ethically applied. The Obama administration released a Roadmap for AI Policy, and the White House has instructed NIST to begin work on Federal Engagement of AI Standards.
For more insights, see: Generative Ai Policy
Regulation is a key aspect of governance, with 82% of Americans believing that robots and AI should be carefully managed. Concerns include surveillance, deep fakes, cyberattacks, data privacy, hiring bias, autonomous vehicles, and drones.
The European Commission has published its "Policy and investment recommendations for trustworthy Artificial Intelligence" and proposed the Artificial Intelligence Act. The OECD, UN, EU, and many countries are working on strategies for regulating AI.
Key initiatives include the European Commission's High-Level Expert Group on Artificial Intelligence, the OECD AI Policy Observatory, and UNESCO's Recommendation on the Ethics of Artificial Intelligence.
Research institutes such as the Future of Humanity Institute, the Institute for Ethics in AI, and the AI Now Institute are also playing a crucial role in studying the social implications of AI.
Here are some key players in the governance and policy space:
- The Partnership on AI to Benefit People and Society
- The IEEE Global Initiative on Ethics of Autonomous and Intelligent Systems
- The European Commission's High-Level Expert Group on Artificial Intelligence
- The OECD AI Policy Observatory
- UNESCO's Recommendation on the Ethics of Artificial Intelligence
Sources
- https://www.captechu.edu/blog/ethical-considerations-of-artificial-intelligence
- https://en.wikipedia.org/wiki/Ethics_of_artificial_intelligence
- https://www.larksuite.com/en_us/topics/ai-glossary/ethical-implications-of-artificial-intelligence
- https://www.isaca.org/resources/isaca-journal/issues/2022/volume-4/bias-and-ethical-concerns-in-machine-learning
- https://www.w3.org/TR/webmachinelearning-ethics/
Featured Images: pexels.com