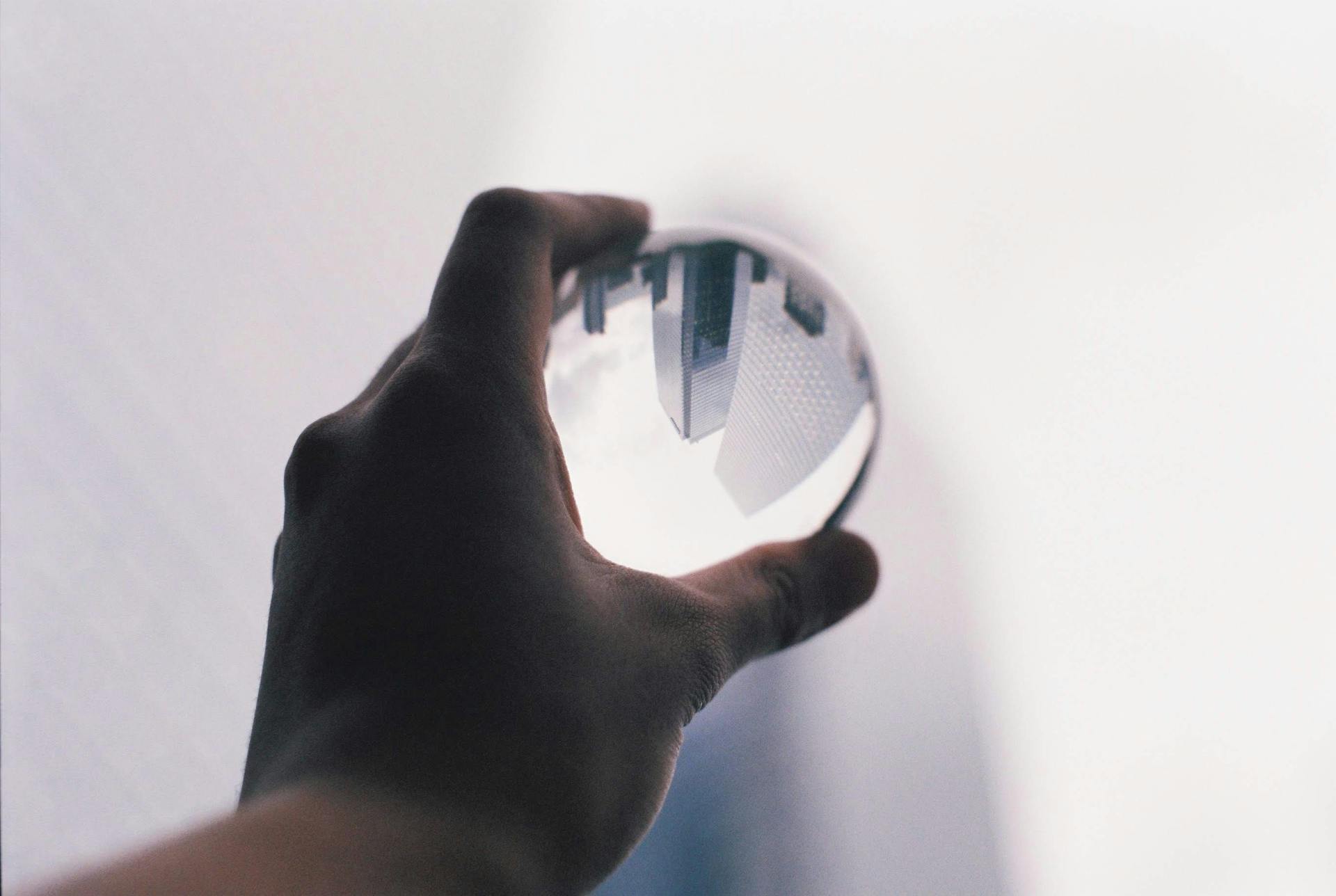
As we explore the ethics of generative AI, it's essential to consider the potential consequences of creating and using these powerful tools. Generative AI can produce highly realistic and convincing content, but this also raises concerns about the potential for misrepresentation and manipulation.
One key consideration is transparency. As we discussed earlier, transparency is crucial in generative AI to prevent the spread of misinformation. This means being open and honest about the source of the content and the methods used to create it.
To achieve transparency, it's essential to provide clear and concise information about the AI model's capabilities and limitations. For instance, if a generative AI is used to create a piece of art, it's essential to disclose the AI's role in the creative process.
Ultimately, the goal of ethics in generative AI is to ensure that these tools are used responsibly and for the greater good. By following best practices and being mindful of the potential consequences, we can harness the power of generative AI to create positive change.
Readers also liked: Create with Confidence Using Generative Ai
Bias and Discrimination
Bias and Discrimination is a serious issue with Generative AI. Generative models can continue biases present in the datasets they are trained on, leading to unfair discrimination.
For example, biased facial recognition software may wrongly identify individuals, causing legal issues and reputational damage. This is exactly what happened with Google's Gemini, which created historically inaccurate images, including depictions of Black Vikings and an Asian woman wearing a German World War II-era military uniform.
Bias in Generative AI is not a new issue, but rather a continuation of problems within machine learning and algorithmic system development. If datasets used for training generative AI models misrepresent, underrepresent, exclude, or marginalize certain social identities, communities, and practices, the models will reflect and often amplify these biases.
To mitigate bias, it's essential to prioritize diversity in training datasets. Conducting regular audits to identify and rectify unintended biases is also crucial. Here are some strategies to promote fairness in Generative AI:
- Monitor and test AI outcomes for group disparities.
- Use diverse, relevant training data to populate an LLM.
- Including a variety of team input during model development.
- Embedding data context in graphs, which can ensure more fair treatment.
Ethics and Challenges
Generative AI raises serious ethical concerns, including bias, misrepresentation, and marginalization, as well as labor exploitation and worker harms.
Bias is a significant issue, with AI discourse and development dominated by large corporate interests, often prioritizing hypothetical benefits and risks over real-world impacts.
The training data for Large Language Models (LLMs) comes from the open internet, inheriting all the ethical concerns about bias, misinformation, disinformation, fraud, privacy, and copyright infringement that exist about the internet.
Some of the most pressing ethical concerns include the creation of deepfakes, doctored videos and audio clips that can be used for identity theft and election manipulation, and the empowerment of scammers through the creation of deepfakes and various applications.
The European Union has recently passed the Artificial Intelligence Act, the first comprehensive global regulatory framework for AI, which defines AI as a machine-based system that operates with varying levels of autonomy and can influence physical or virtual environments.
Worth a look: Ethical Implications of Ai
Here are some of the key ethical concerns with generative AI:
- Bias, misrepresentation, and marginalization
- Labor exploitation and worker harms
- Misinformation and disinformation
- Privacy violations and data extraction
- Copyright and authorship issues
- Environmental costs
These concerns highlight the need for transparency and accountability in generative AI applications, and the importance of establishing regulations to mitigate the risks associated with this technology.
Ethics and Challenges
Generative AI is implicated in a host of ethical issues and social costs, including bias, misrepresentation, and marginalization. These issues are not just hypothetical, but real-world problems that need to be addressed.
Scholars have pointed out that AI discourse and development has been dominated by large corporate interests, with a focus on hypothetical benefits and risks rather than current, real-world impacts. This has led to a lack of transparency and accountability in generative AI applications.
One of the major concerns with generative AI is labor exploitation and worker harms. This is a critical issue that needs to be addressed, as AI technologies continue to develop and become more integrated into our daily lives.
Readers also liked: Generative Ai Legal Issues
Generative AI's use of copyrighted material in training data sets and generated content may lead to copyright infringement issues. This is a major challenge that businesses and governments need to consider when implementing generative AI technologies.
The lack of AI-specific legislation and regulatory standards highlights the need for transparency and accountability in generative AI applications. This is an area that requires urgent attention, to ensure that the benefits of generative AI are shared by all stakeholders.
Some of the key ethical concerns surrounding generative AI include:
- Bias, misrepresentation, and marginalization
- Labor exploitation and worker harms
- Misinformation and disinformation
- Privacy violations and data extraction
- Copyright and authorship issues
- Environmental costs
These issues are complex and multifaceted, and require a comprehensive approach to address them. By understanding the challenges and risks associated with generative AI, we can work towards creating a safer and more equitable AI ecosystem.
Environmental Impact
Generative AI systems consume huge amounts of energy, much more than conventional internet technologies. They require large quantities of fresh water to cool their processors.
Training a single AI model can emit as much carbon as five cars in their lifetimes, according to a 2019 study. This is a staggering amount of emissions, and it's only getting worse.
Data center emissions are probably 662% higher than big tech claims, according to a 2024 Guardian article. This is a disturbing revelation, and it highlights the need for more transparency in the tech industry.
The environmental impacts of generative AI often fall disproportionately on socioeconomically disadvantaged regions and localities. This is a serious issue that needs to be addressed.
Generative AI companies including Microsoft, Google, and Amazon have recently signed deals with nuclear power plants to secure emissions-free energy generation for their AI data centers. This is a positive step towards reducing the environmental impact of these companies.
Here are some key statistics on the environmental impact of generative AI:
- Data center emissions: 662% higher than big tech claims
- Training a single AI model: emits as much carbon as five cars in their lifetimes
- Generative AI energy consumption: much more than conventional internet technologies
Honor Human Autonomy
Generative AI technologies threaten human autonomy by "over-optimizing the workflow, hyper-personalization, or by not giving users sufficient choice, control, or decision-making opportunities." This can be seen in the way AI systems make choices for us, such as in healthcare, where the buck should stop with medical professionals.
Respecting autonomy alludes to maintaining the natural order of what humans do, such as making choices. Companies can strive to respect human autonomy by being ethically minded when nurturing talent for developing and using GenAI.
In certain contexts, AI technologies can be seen as a threat to human autonomy. For example, AI systems can "over-optimizing the workflow" or "hyper-personalization" without giving users sufficient choice, control, or decision-making opportunities.
To honor human autonomy, companies should prioritize transparency and accountability in GenAI applications. This includes being open about the data used to train AI systems and providing users with clear information about how AI decisions are made.
Here are some ways to prioritize human autonomy in GenAI:
- Be ethically minded when nurturing talent for developing and using GenAI
- Provide users with clear information about how AI decisions are made
- Be transparent about the data used to train AI systems
Data and Security
Data and Security is a top concern when it comes to generative AI. Generative models trained on personal data can pose significant privacy risks, as they may generate synthetic profiles that closely resemble real individuals.
According to a study, 15% of employees put company data in ChatGPT, where it becomes public. This highlights the need for stronger data security measures, such as encryption and robust data storage.
To safeguard user data, anonymizing data during training and implementing robust data security measures is essential. Adhering to principles like GDPR’s data minimization can also help minimize the risk of privacy breaches.
Here are some key steps to protect sensitive data:
- Setting up strong enterprise defenses
- Using robust encryption for data storage
- Using only zero- or first-party data for GenAI tasks
- Denying LLMs access to sensitive information
- Processing only necessary data (a GDPR principle)
- Anonymizing user data
- Fine-tuning models for particular tasks
Remember, individuals should use caution and avoid sharing personal information with generative AI tools, as this information may be used as training data and show up later in prompt responses given to other users.
Environmental Costs
Training and using generative AI models can require a lot of energy, increasing emissions and consuming drinking water.
Data center emissions are probably 662% higher than big tech claims, according to a report by The Guardian in September 2024.
This is a significant concern, as AI systems consume huge amounts of energy - much more than conventional internet technologies.
Broaden your view: Generative Ai Energy Consumption
Training a single AI model can emit as much carbon as five cars in their lifetimes, as reported by MIT Technology Review in June 2019.
Generative AI companies are starting to take steps to reduce their environmental impact, such as signing deals with nuclear power plants to secure emissions-free energy generation for their AI data centers.
Here are some examples of companies taking action:
- Amazon, Google, and Microsoft have signed deals with nuclear power plants in Pennsylvania and Washington.
- Google and Microsoft have reported their environmental impacts, but some companies do not disclose this information in detail.
The U.S. Congress proposed the Artificial Intelligence Environmental Impacts Bill of 2024 to encourage voluntary reporting of environmental data from generative AI companies.
Curious to learn more? Check out: Generative Ai Environmental Impact
Data Privacy
Data Privacy is a major concern with Generative AI models. They can scrape large datasets from the web that contain personal information, and some tools may use user input to train models or provide future outputs.
Researchers have discovered ways to extract training data directly from AI models, including ChatGPT. This raises huge security implications for users.
AI chatbots can be tricked into misbehaving, and scientists are still figuring out how to stop it. This is a serious issue, as it can lead to breaches of user privacy and legal consequences.
Here's an interesting read: How Are Modern Generative Ai Systems Improving User Interaction
To safeguard user data, anonymizing data during training and implementing robust data security measures, such as encryption, is essential. Adhering to principles like GDPR’s data minimization can also help minimize the risk of privacy breaches.
Consumers are becoming increasingly aware of corporate data breaches and are advocating for stronger cybersecurity. GenAI models are in the spotlight because they often collect personal information, and consumers’ sensitive data isn’t the only type at risk.
Most company leaders understand that they must do more to reassure their customers that data is used for legitimate purposes. In response, 63% of organizations have placed limits on which data can be entered digitally, while 61% are limiting the GenAI tools employees are allowed to use.
Here are some ways executives and developers can protect sensitive data:
- Setting up strong enterprise defenses
- Using robust encryption for data storage
- Using only zero- or first-party data for GenAI tasks
- Denying LLMs access to sensitive information
- Processing only necessary data (a GDPR principle)
- Anonymizing user data
- Fine-tuning models for particular tasks
Individuals should use caution and avoid sharing personal information with generative AI tools. Chatting with AI bots in human-like "conversations" can lead to unintentional oversharing of such personal information.
Misinformation and Disinformation
Generative AI is being used to create manipulated and entirely faked text, video, images, and audio, sometimes featuring prominent politicians and celebrities. These tools make it easier for bad actors to create persuasive, customized disinformation at scale.
Digital watermarking and automated detection systems are insufficient on their own, as these can be bypassed in various ways. Generative AI may also provide factually inaccurate outputs, generate "fake citations", or misrepresent information in other sources.
As AI models improve, it is increasingly difficult to tell the difference between images of real people and AI-generated images. AI-powered image manipulation tools are also being built into the latest generations of smartphones, with broad implications for fact-checking and navigating social media.
Some examples of AI-generated misinformation include AI-generated audio of politicians and celebrities, and AI-generated images that are nearly indistinguishable from real people. These tools can be used to spread false information and propaganda, and can be particularly effective on social media platforms.
Consider reading: Can Generative Ai Improve Social Science
Here are some notable examples of AI-generated misinformation:
- AI-generated audio of politicians and celebrities on TikTok
- AI-generated images that are nearly indistinguishable from real people
- Chatbots that "hallucinate" and provide factually inaccurate information
- AI-generated text that includes "fake citations" and misrepresents information
These tools can have serious consequences, including the spread of misinformation and propaganda, and the undermining of trust in institutions and individuals. It's essential to be aware of these risks and to take steps to verify the accuracy of information, especially when it comes from AI-generated sources.
Copyright and IP
Generative AI models are trained on large datasets, including copyrighted works, without the creators' knowledge or consent. This raises concerns about copyright infringement and intellectual property.
Some lawsuits have already been filed against companies like OpenAI and Meta, alleging copyright infringement. The U.S. Copyright Office has stated that works created by generative AI cannot be copyrighted, as they are not founded in the creative powers of the human mind.
To prevent unintentional infringements, companies should ensure that training content is properly licensed and transparently document how generated content is produced. Implementing metadata tagging in training data can help trace the origins of generated content, reducing the risk of copyright violations.
On a similar theme: How to Learn Generative Ai
Here are some potential copyright issues with generative AI:
- Generative AI models use copyrighted material from websites, social networks, and other sources without attribution or reimbursements.
- Content creators are concerned about the use of their material without permission.
- Lawsuits have been filed by artists and Getty Images, claiming copyright violations based on the training of AI image programs.
Scholarly Publishing
Scholarly publishing is a rapidly evolving field, and AI is playing a significant role in it. Oxford University Press is actively working with AI companies to explore new opportunities.
Some major academic publishers have signed deals with AI companies, sparking controversy among professors. Christa Dutton reported that two major academic publishers have signed such deals.
These deals have raised concerns about the use of academic content for AI training. The Author's Guild recommended including a clause in publishing and distribution agreements to prohibit AI training uses. This recommendation was made in March 2023.
You might enjoy: Generative Ai Companies
Copyright and IP
Generative AI models are trained on large datasets, including copyrighted materials, without the creators' knowledge or consent.
The U.S. Copyright Office has stated that works created by generative AI cannot be copyrighted, as they are not founded in the creative powers of the human mind. Instead, they pass immediately into the public domain.
Lawsuits have been filed by The New York Times and other entities because their copyrighted material has been taken from the internet and used as training data for LLMs. This copyrighted material has appeared verbatim in text generated by the tools.
To prevent unintentional infringements, companies should ensure that training content is properly licensed and transparently document how generated content is produced. Implementing metadata tagging in training data can help trace the origins of generated content, reducing the risk of copyright violations.
A Congressional Research Services report examined copyright issues on both sides of the equation. On one hand, artists have filed lawsuits claiming that their copyrighted works were infringed when they were used as part of the training of AI image programs. On the other hand, the report discussed whether content produced by generative AI, such as DALL-E 2, can be copyrighted as an original work.
Here are some key concerns about copyright and IP:
- Generative AI's ability to replicate copyrighted materials raises concerns about intellectual property infringement.
- Companies should ensure that training content is properly licensed to prevent unintentional infringements.
- Implementing metadata tagging in training data can help trace the origins of generated content, reducing the risk of copyright violations.
Transparency and Accountability
Transparency and accountability are crucial aspects of generative AI. Establishing clear policies on the responsible use of generative AI can help clarify boundaries and ensure accountability, similar to platforms like X (formerly known as Twitter).
To address the lack of transparency in AI systems, researchers and developers need to work on enhancing transparency, including understanding emergent capabilities and factors influencing decision-making. This can help improve trust in generative AI and ensure accountability for its outcomes.
Transparency and accountability can be achieved through various means, such as:
- Providing context and peripheral information to facilitate understanding of the pathways of logic processing
- Using graph databases like Neo4j to enhance transparency
- Building in accountability by acknowledging issues, determining whether changes are needed, and making the necessary changes
- Ensuring explainability through the ability to verify, trace, and explain how responses are derived
By prioritizing transparency and accountability, companies can build trust with their users and ensure that their generative AI systems operate in an ethical and responsible manner.
Lack of Transparency
The lack of transparency in AI systems is a major concern. It's difficult to understand their decision-making processes, leading to uncertainty and unpredictability.
Researchers and developers are working on enhancing transparency in AI systems, including understanding emergent capabilities and factors influencing decision-making. This is crucial for improving trust in generative AI and ensuring accountability for its outcomes.
You might enjoy: Why Is Controlling the Output of Generative Ai Important
Currently, generative AI models are not Explainable AI (XAI)-based, which means they can't explain their actions and decisions in a way that's comprehensible to humans. This is a significant limitation.
The complexity of generative AI models makes it challenging to offer clear explanations about how they make decisions. Simplifying these models could reduce their effectiveness, which is a trade-off that developers are struggling with.
In critical sectors like finance and healthcare, transparency is vital for enhancing trust and accountability. Jurkiewicz highlights that hyperspecific use cases can create transparency and traceability, which may improve the ability to achieve a higher level of responsibility and regulatory compliance.
Be Transparent
Transparency is key to building trust in generative AI systems. Establishing clear policies on the responsible use of generative AI, similar to platforms like X (formerly known as Twitter), can help clarify boundaries and ensure accountability.
To achieve transparency, researchers and developers need to work on enhancing transparency in AI systems, including understanding emergent capabilities and factors influencing decision-making. This can help improve trust in generative AI and ensure accountability for its outcomes.
For your interest: Ai Accountability Essential Training
A system can provide context, which facilitates understanding of the pathways of logic processing. Explicitly incorporating context ensures that the technology doesn’t violate ethical principles. One way for a company to enhance transparency is to incorporate context by using a graph database such as Neo4j.
Transparency is not just about providing information, but also about being able to explain how decisions are made. If a company can’t explain how a decision was made, it can lead to public distrust of AI. To address this, companies can use knowledge graphs and metadata tagging to allow for backward tracing to show how generated content was created.
Here are four components of explainability:
- Being able to cite sources and provide links in a response to a user prompt
- Understanding the reasoning for using certain information
- Understanding patterns in the “grounding” source data
- Explaining the retrieval logic: how the system selected its source information
By storing connections between data points, linking data directly to sources, and including traceable evidence, knowledge graphs facilitate LLM data governance. For instance, if a company board member were to ask a GenAI chatbot for a summary of an HR policy for a specific geographic region, a model based on a knowledge graph could provide not just a response but the source content consulted.
Worth a look: Generative Ai Knowledge Management
Frequently Asked Questions
What are the 5 ethics of AI?
The 5 key ethics of AI are Transparency, Impartiality, Accountability, Reliability, and Security & Privacy, ensuring AI systems operate safely and responsibly. Understanding these ethics is crucial for developing trustworthy AI that benefits society.
What ethical considerations arise from using generative AI for job interview preparation?
Using generative AI for job interview preparation raises concerns about unfair biases and lack of transparency in the hiring process, potentially leading to unequal opportunities and unfair treatment of candidates
Featured Images: pexels.com