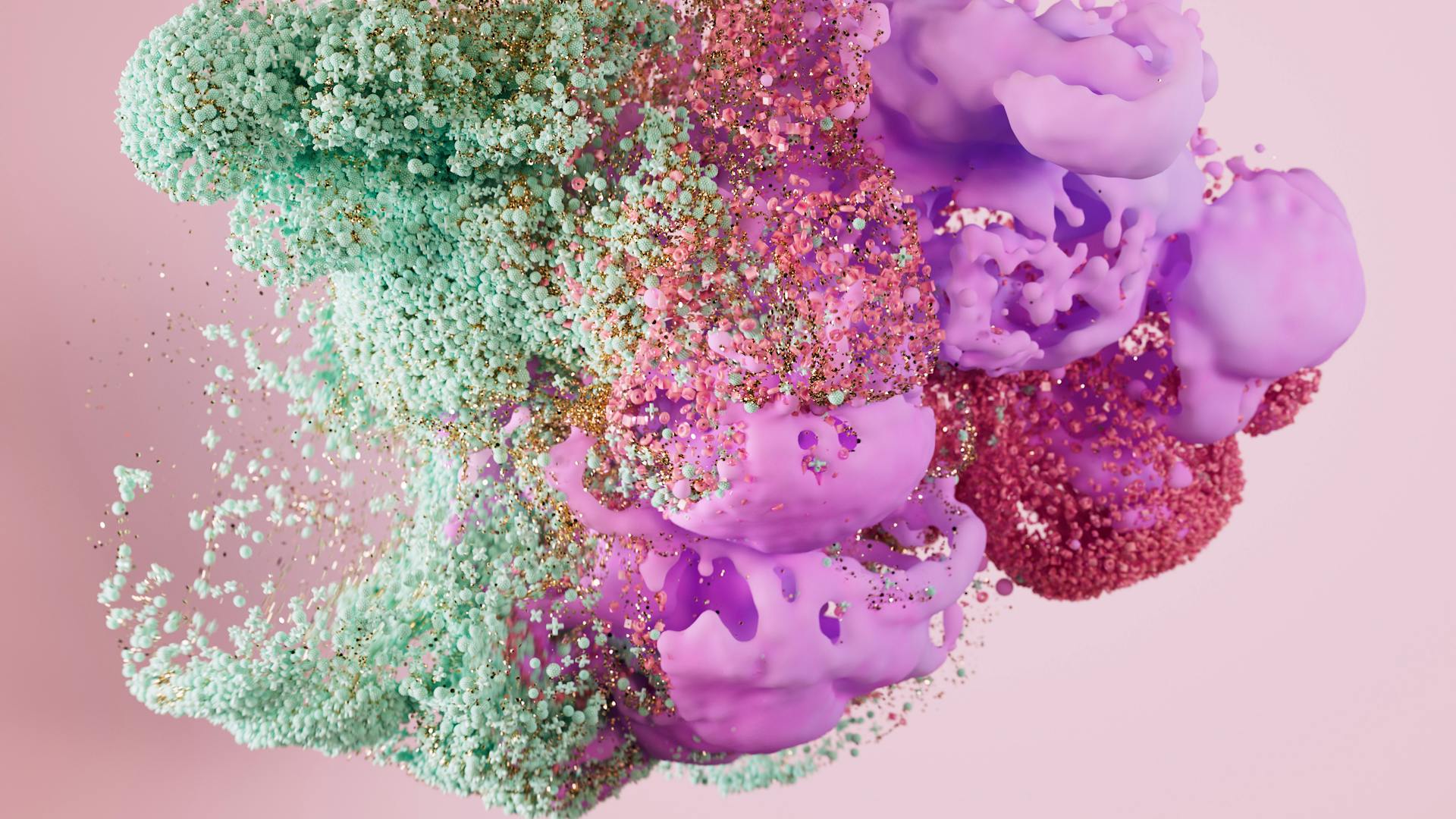
Generative AI agents have revolutionized the way we approach creativity and efficiency. They can generate high-quality content, such as images, music, and text, with unprecedented speed and accuracy.
These agents use complex algorithms to analyze vast amounts of data and learn from it, allowing them to create unique and innovative outputs. For instance, a generative AI agent can learn from a dataset of paintings and generate new artwork that is similar yet distinct from the originals.
Generative AI agents can also automate repetitive tasks, freeing up human time and resources for more creative and high-value work. This can be seen in industries such as marketing, where AI-generated content can help personalize customer experiences and improve engagement.
By harnessing the power of generative AI agents, businesses and individuals can unlock new levels of creativity and efficiency, leading to increased productivity and innovation.
A fresh viewpoint: Can I Generate Code Using Generative Ai Models
What is?
Generative AI agents are capable of producing new content that's indistinguishable from human-created data. This is made possible by algorithms like Generative Adversarial Networks (GANs) and Transformer architectures.
They can generate a wide range of content, including text, images, and even music. With AI agents, you can automate tasks that used to require human input, freeing up experts to focus on high-value processes.
The adoption of AI agents represents a significant turning point in business tech, enabling faster decision-making and increased productivity. This is because AI agents can learn and improve with minimal intervention.
AI agents can be built using tools like Agentforce, which allows you to describe what you need it to do using natural language, and it does the rest. No IT expertise is required to build AI agents with Agentforce.
How It Works
Generative AI agents operate as sophisticated systems capable of managing complex workflows autonomously. They integrate Generative models with a multi-agent framework, allowing them to execute tasks from start to finish, adapt to new information, and refine their outputs based on user feedback.
The process begins with the user input, which is then directed to the Generative AI agent. This agent uses a large language model (LLM) to understand the query and formulate a plan.
Explore further: How Are Modern Generative Ai Systems Improving User Interaction
AI agents start by gathering data from various sources, including customer interactions, transaction histories, and social media. This data is crucial for understanding the context and nuances of customer queries.
Using sophisticated machine learning models, AI agents analyze the collected data to identify patterns and make decisions. They can determine the most appropriate response to a customer query based on past interactions and the current context.
AI agents can execute tasks autonomously, such as making product recommendations, troubleshooting problems, and engaging in follow-up interactions. This frees up human agents to focus on more complex and value-added activities.
The Generative AI agent system interprets the user's prompt and constructs a comprehensive work plan. A manager agent oversees the entire process and subdivides the task into smaller, manageable components.
Here's a breakdown of the specialist agents involved:
These specialist agents work collaboratively, sharing insights and findings with each other to ensure a cohesive approach towards task completion.
Benefits and Applications
Generative AI agents have numerous benefits and applications that can transform businesses and customer interactions. They offer 6 benefits, including enhancing user engagement and satisfaction through personalization at scale.
Automation of creative tasks is another significant advantage, as generative AI agents can handle repetitive tasks, freeing up human creators to focus on higher-level concepts. This allows for more efficient use of time and resources.
By adopting AI agents, businesses can scale their customer service, improving efficiency and generating revenue from the contact center to the field. This is made possible by the power of generative AI on a unified foundation of trusted data.
McKinsey’s report highlights the promise and reality of gen AI agents in the enterprise, providing valuable insights into their applications and use cases.
Take a look at this: Generative Ai Customer Service
Challenges and Risks
The potential misuse of Generative AI agents is a significant challenge that needs to be addressed. Misinformation can be generated in the form of fake news or misleading content, which can have serious consequences.
Developing tools to identify and prevent misuse is crucial. Regulation and detection methods are necessary to mitigate the risks associated with Generative AI agents.
Improving the explainability and transparency of AI systems is also essential. AI agents often operate as "black boxes", making it difficult to understand their decision-making processes.
Challenges and Risks
Generative AI agents are complex systems that can be prone to misuse. One potential issue is the creation of misinformation through fake news or misleading content.
Misinformation can have serious consequences, including erosion of trust in institutions and the spread of false information. In fact, fake news or misleading content can be generated with relative ease.
Developing tools to identify and prevent misuse is crucial. This includes regulation and detection methods to prevent the spread of misinformation. Regulation and detection tools can help mitigate the risks associated with Generative AI agents.
Improving the explainability and transparency of AI systems is also essential. This is because AI agents often operate as "black boxes", making it difficult to understand their decision-making processes. By improving explainability and transparency, we can build trust and ensure accountability in AI systems.
Here are some examples of the challenges and risks associated with Generative AI agents:
- Misinformation: Generating fake news or misleading content.
- Regulation and Detection: Developing tools to identify and prevent misuse.
Human-in-the-Loop Approaches
One way to address the challenges and risks of AI is by using Human-in-the-Loop Approaches, which combine human creativity with AI efficiency. This allows for more accurate and reliable results.
Collaboration is a key aspect of this approach, where humans and AI systems work together to achieve a common goal. By combining the strengths of both, we can create something greater than the sum of its parts.
Validation is another important part of Human-in-the-Loop Approaches, where humans review and refine AI-generated content to ensure it meets the required standards. This step is crucial in preventing errors and inaccuracies.
Here are some key benefits of Human-in-the-Loop Approaches:
- Combining human creativity with AI efficiency.
- Validation of AI-generated content by humans.
Regulatory and Ethical Considerations
As generative AI agents become more prevalent, regulatory and ethical considerations are crucial to ensure their responsible development and deployment. Developing comprehensive frameworks to govern the use of AI is essential to address these concerns.
Transparency is a key principle in AI development, making the workings of AI systems understandable is vital. This means that AI developers should strive to explain how their systems work and make their decision-making processes transparent.
A different take: Generative Ai for Software Development
Bias and fairness are also significant concerns, as AI models can inadvertently perpetuate biases present in their training data. Ensuring fairness and mitigating bias in AI systems is critical to avoid discriminatory outcomes and ensure equitable treatment for all users.
Ensuring accountability for AI actions is also essential, establishing responsibility for AI decisions and outcomes is vital. This can be achieved by implementing mechanisms that hold AI developers and deployers accountable for their AI systems.
Determining ownership of content created by AI is another challenge, with evolving laws to address AI-generated works. This includes determining who owns the rights to AI-generated content, such as art, music, or writing.
Here are some key principles to consider when developing regulatory frameworks for generative AI agents:
- Transparency: Making the workings of AI systems understandable.
- Accountability: Establishing responsibility for AI actions.
Future Outlook
The future of Generative AI agents is looking bright, with companies investing heavily in AI to gain a competitive edge.
Continuous advancements in AI research and technology are driving this growth, and we can expect to see significant progress in the next few years.
Companies like BCG are already highlighting the potential for mainstream adoption of autonomous agents capable of executing complex tasks independently.
This shift will require companies to develop robust transformation roadmaps and invest in the necessary infrastructure and talent to stay ahead.
Frequently Asked Questions
What are the 5 types of agents in AI?
AI agents are categorized into five primary types: Simple Reflex Agents, Model-Based Agents, Goal-Based Agents, Utility-Based Agents, and Learning Agents. Each type of agent uses a distinct approach to make decisions and interact with their environment
What are generative AI examples?
Generative AI examples include creating new text, images, music, audio, and videos. These can range from generating art and music to producing written content, such as articles and stories.
What is the difference between GenAI and AI agent?
Gen-AI chatbots react to user inputs, while Agentic AI systems are proactive, planning ahead and adapting to new challenges
Sources
- https://arunapattam.medium.com/agents-in-generative-ai-a-comprehensive-overview-6458be008b08
- https://www.linkedin.com/pulse/unleashing-creativity-generative-ai-agents-nagesh-deshmukh-lhxlc
- https://www.salesforce.com/agentforce/what-are-ai-agents/
- https://www.oracle.com/artificial-intelligence/generative-ai/agents/
- https://www.cio.com/article/3488553/ai-agents-loom-large-as-organizations-pursue-generative-ai-value.html
Featured Images: pexels.com