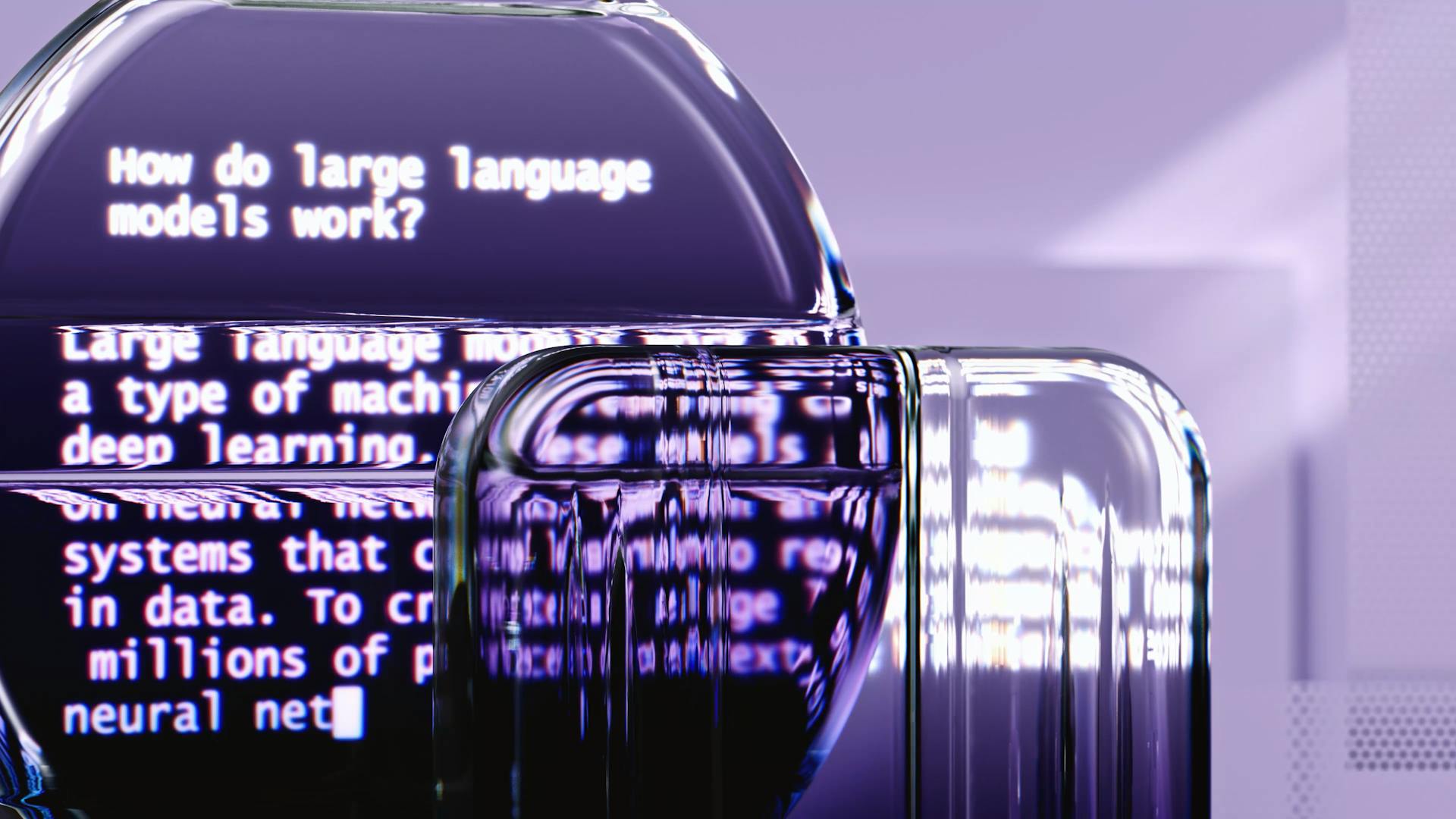
Generative AI DLP can be a double-edged sword, offering both benefits and risks.
The benefits of Generative AI DLP include improved data discovery and classification, which can lead to more accurate risk assessments and compliance.
One of the main risks of Generative AI DLP is the potential for data bias and inaccuracies.
Curious to learn more? Check out: Advantages of Generative Ai
Understanding Generative AI DLP Risks
Generative AI DLP risks are real and can be categorized into three main areas: unintended data generation, data exposure through prompts, and model poisoning and data extraction. These risks can lead to the creation of inaccurate or sensitive information that appears authoritative.
Generative AI tools can inadvertently expose sensitive information through data inputs, such as fictional customer data or financial projections that sound plausible. This can be damaging if shared internally or externally. Strac's solutions help mitigate these risks by controlling usage, blocking sensitive data, and monitoring and reporting AI usage and data interactions.
Here are some key statistics on the risks of generative AI:
To manage these risks proactively, it's essential to assess your current AI usage, identify the types of data being processed, and map data flows. This will help you understand how information moves between AI tools and other applications, and review your organization's existing DLP controls and their effectiveness in dealing with the risks posed by generative AI.
Related reading: Generative Ai Risks
Model Poisoning and Extraction
Generative AI models can be manipulated by attackers to produce incorrect or biased results, which can compromise the integrity of an AI-based smart security system and lead to data breaches.
This type of attack is called model poisoning, and it's a serious concern that can have devastating consequences.
Attackers might attempt to manipulate the training data of an AI model to produce incorrect or biased results, which can be used to gain unauthorized access to sensitive information.
For example, an attacker might manipulate the training data of a generative AI model to produce fictional customer data or financial projections that sound plausible and would be damaging if shared internally or externally.
Here are some ways model poisoning can occur:
- Manipulating the training data to produce biased results
- Introducing backdoors into the AI model
- Using AI-based attacks to evade security measures
- Model poisoning can be used to compromise the integrity of an AI-based smart security system and lead to data breaches.
To mitigate the risks of model poisoning, it's essential to implement robust security measures, such as:
- Regularly monitoring and updating AI models
- Implementing data validation and sanitization
- Using secure data storage and transmission protocols
- Conducting regular security audits and penetration testing
Assess Current
Assessing your current generative AI usage is a crucial step in understanding the risks associated with it. You should conduct a thorough inventory of all generative AI tools in use across your organization, identifying the types of data being processed by these tools and categorizing them according to their level of sensitivity.
A fresh viewpoint: Top Generative Ai Tools
To do this, you'll want to map data flows to understand how information moves between the AI tools and other applications. This will help you identify potential vulnerabilities and areas where sensitive data may be exposed. Reviewing your organization's existing DLP controls and their effectiveness in dealing with the risks posed by generative AI is also essential.
Here's a step-by-step guide to help you assess your current AI usage:
- Conduct a thorough inventory of all generative AI tools in use across your organization.
- Identify the types of data being processed by these tools, categorizing them according to their level of sensitivity.
- Map data flows to understand how information moves between the AI tools and other applications.
- Review your organization’s existing DLP controls and their effectiveness in dealing with the risks posed by generative AI.
By following these steps, you'll be able to gain a better understanding of your organization's current generative AI usage and identify areas where you can improve your DLP controls to mitigate potential risks.
Set Up Continuous Monitoring and Auditing
Setting up continuous monitoring and auditing is crucial to ensure the security and integrity of your generative AI systems. This involves configuring real-time alerts for potential data leaks or policy violations in AI interactions.
To detect anomalous patterns in AI usage, make use of user behavior analytics (UBA). This can help identify insider threats before they cause harm.
Regular audits of AI-generated content are also necessary to ensure compliance with data protection policies. This can be done using tools that automate the task of classifying and labeling data.
Establish a process for investigating and remedying incidents, and make sure to regularly review and update your data classification scheme.
Here are the key steps to set up continuous monitoring and auditing:
- Configure real-time alerts for potential data leaks or policy violations in AI interactions.
- Make use of user behavior analytics (UBA) to detect anomalous patterns in AI usage.
- Conduct regular audits of AI-generated content to ensure compliance with data protection policies.
- Establish a process for investigating and remedying incidents.
Having a detailed log of all interactions with generative AI systems is vital for real-time monitoring and post-incident analysis. This log should record user identities, input prompts, output summaries, and any data accessed or generated during each interaction.
Technical Implementation
Implementing technical controls is a crucial step in managing generative AI data loss prevention (DLP). This involves using content inspection tools to scan generative AI inputs and outputs in real-time.
To restrict the flow of sensitive data, configure DLP software to recognize your organization's sensitive data and block it from generative AI tools. This can be done at various levels, including API.
Curious to learn more? Check out: What Are Generative Ai Tools
To track all interactions with generative AI platforms, set up logging and monitoring systems. This will help you monitor and manage the flow of data between AI tools and other applications.
Here are some key technical controls to implement:
- Content inspection tools to scan generative AI inputs and outputs in real-time.
- DLP software to recognize and block sensitive data from generative AI tools.
- Controls at API level to restrict data flow between AI tools and other applications.
- Logging and monitoring systems to track all interactions with generative AI platforms.
Step 3: Technical
Implementing technical controls is a crucial step in ensuring the secure use of generative AI in your organization. This involves using content inspection tools to scan inputs and outputs in real-time.
To block sensitive data from being accessed by generative AI tools, configure your DLP software to recognize and flag your organization's sensitive data. This can be done by setting up specific rules and filters to identify and block sensitive data.
Setting up controls at the API level is also essential to restrict the flow of data between AI tools and other applications. This can be achieved by implementing API keys, tokens, or other authentication mechanisms.
Logging and monitoring systems should be set up to track all interactions with generative AI platforms. This can be done by configuring logging tools to record all API calls, data transfers, and other relevant events.
Here are some key technical controls to implement:
- Use content inspection tools to scan inputs and outputs in real-time.
- Configure DLP software to recognize and block sensitive data.
- Set up controls at the API level to restrict data flow.
- Set up logging and monitoring systems to track interactions.
Flow to and from Tools with Policies
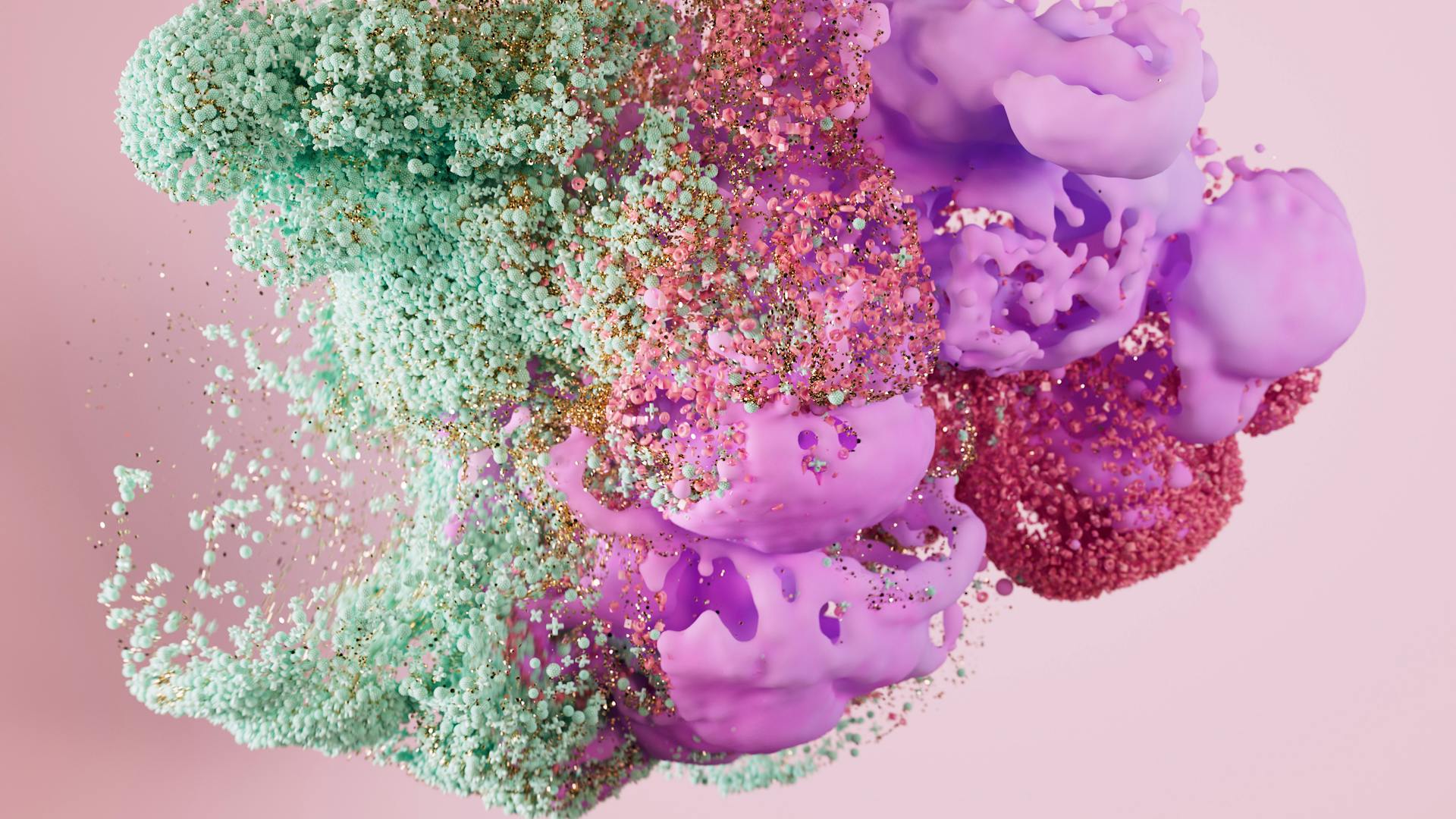
Implementing policies to control the flow of data to and from AI tools is a crucial step in technical implementation.
Cyberhaven records all data flows to the internet without any configuration or policies needed, allowing your team to understand what sensitive data is flowing to and from these tools.
You can define incredibly simple policies with Cyberhaven to prevent sensitive data from flowing to dangerous AI tools.
With Cyberhaven, you can define policies that scale visibility and control up or down, saving analysts' time and reducing false positives.
Here are some key features of dynamic policies for visibility and control:
By implementing these policies, you can ensure that your sensitive data is protected from unauthorized access and misuse.
Sources
- https://www.teramind.co/blog/generative-ai-dlp/
- https://www.strac.io/integrations/generative-ai-dlp
- https://umbrella.cisco.com/trends-threats/generative-ai-cybersecurity-risks-and-rewards
- https://www.cyberhaven.com/technologies/gen-ai
- https://www.proofpoint.com/us/blog/information-protection/whats-best-way-stop-genai-data-loss-take-human-centric-approach
Featured Images: pexels.com