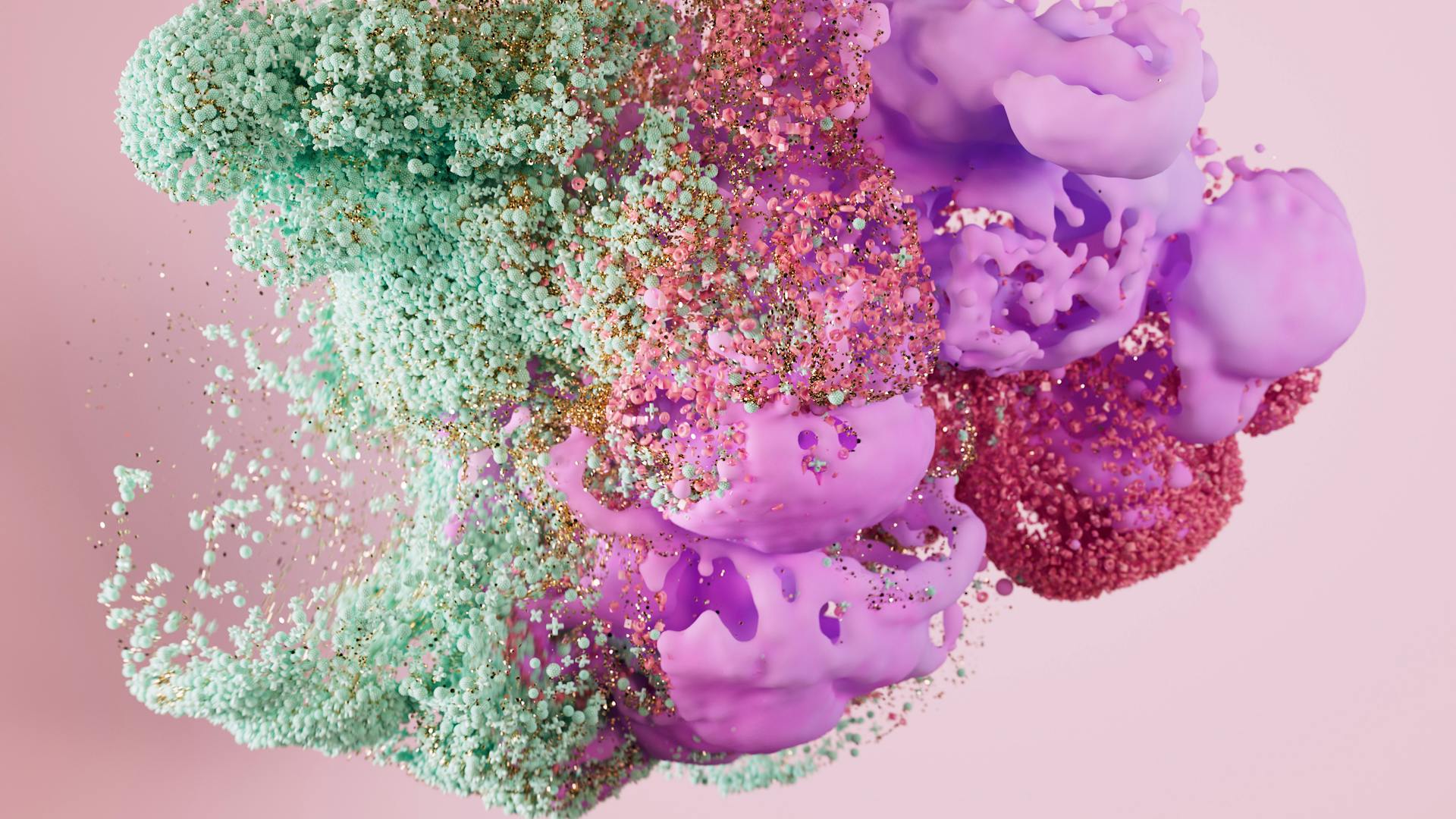
Generative AI is revolutionizing life sciences by streamlining research and development processes. By leveraging large datasets and machine learning algorithms, generative AI can quickly generate new molecules, predict protein structures, and identify potential drug targets.
This technology has the potential to accelerate the discovery of new treatments for complex diseases, such as cancer and Alzheimer's. With generative AI, researchers can explore vast chemical spaces to identify novel compounds that may not have been discovered through traditional methods.
One example of generative AI in action is in the design of new molecules. Researchers can use generative models to generate thousands of new molecular structures in a matter of seconds, allowing them to quickly identify promising candidates for further study.
Recommended read: Google Announces New Generative Ai Search Capabilities for Doctors
10 Use Cases
Generative AI has found numerous applications in life sciences, helping to drive research and development, optimize processes, and generate new insights. Some of the most notable use cases include:
Generative AI can unlock $5–7 billion in value for the life sciences sector, with 90% of this sum coming from three functions: clinical research, manufacturing and supply chain, and commercial.
Generative AI helps to forecast market, regulatory, and patient population changes, enabling strategic choices through scenario modeling and risk analysis.
Generative AI tools assist in finding biomarkers for how well drugs work and uncovering possible risk factors, leading to advances in customized medicine and drug creation.
Generative AI can automate clinical summaries, enhance efficiency, and assist regulatory compliance in medical writing, potentially saving billions in R&D.
Here are some of the key areas where generative AI is making its mark in life sciences:
Data Augmentation
Generative models can generate synthetic data to augment existing datasets, helping to improve the performance of AI models in tasks like the analysis of medical images.
This can be particularly useful in medical imaging, where data augmentation can help AI models detect diseases more accurately. For example, in the analysis of medical images, generative models can generate synthetic data to help improve the performance of AI models.
Generative models can also help improve the performance of AI models in drug discovery. By generating synthetic data, researchers can test and refine their models more efficiently, leading to faster and more accurate discoveries.
Data augmentation can also be used to improve the accuracy of downstream analysis, such as cell-type identification and gene expression quantification, in single-cell RNA sequencing (scRNA-seq) data.
A different take: Synthetic Data Generative Ai
Image and Text Generation
Generative AI in life sciences is opening up new possibilities for data visualization and hypothesis testing.
Generative models can be used to generate images of biological structures or processes based on textual descriptions, which can be helpful in visualizing complex phenomena.
This can be particularly useful for researchers who want to explore and understand complex data, but may not have the resources or expertise to create visualizations from scratch.
Generative models can also be used to generate data for hypothesis testing, which can help scientists to better understand the underlying mechanisms of biological systems.
You might enjoy: Generative Ai Testing
Novel Molecule Generation
Generative models like VAEs and GANs can be used to design novel drug-like molecules with desired properties.
These models can generate molecules with high binding affinity to a target protein, making them potentially effective treatments.
VAEs and GANs can also design molecules with low toxicity, reducing the risk of adverse side effects.
This approach has the potential to revolutionize the field of drug discovery by providing a more efficient and targeted way to develop new medications.
By leveraging generative models, researchers can create novel molecules that may not have been possible to design through traditional methods.
These molecules can then be tested and refined to create effective treatments for a wide range of diseases and conditions.
Worth a look: Generative Ai Drug Discovery
Text-to-Image Generation
Text-to-image generation is a powerful tool for visualizing complex biological phenomena. Generative models can be used to generate images of biological structures or processes based on textual descriptions.
This can be especially helpful for researchers who need to understand complex data or generate data for hypothesis testing. By providing a visual representation of biological processes, text-to-image generation can facilitate a deeper understanding of the underlying mechanisms.
For instance, scientists can use text-to-image generation to create images of molecular structures or cellular processes based on detailed descriptions in scientific papers. This can aid in the development of new treatments or therapies by providing a clearer understanding of the biological systems involved.
For more insights, see: Generative Ai Text Analysis
Simulating Biological Processes
Simulating biological processes is a game-changer for researchers, allowing them to create realistic simulations of cellular signaling and metabolic pathways.
These simulations can help predict behavior under different conditions, giving scientists a better understanding of complex biological systems. This can be particularly useful for studying rare diseases or developing new treatments.
Generative AI models can create these simulations, making it easier to study and analyze biological processes without the need for extensive experimentation.
Readers also liked: Create with Confidence Using Generative Ai
Biomatter
Biomatter is a company that's pushing the boundaries of synthetic biology. They specialize in designing novel enzymes using a powerful algorithm called ProteinGAN.
This algorithm is run on Biomatter's Intelligent Architecture platform, which combines generative AI with physical modeling. The result is entirely new and functional enzymes that didn't exist before.
Biomatter's approach has the potential to revolutionize various industries, from biomanufacturing to medicine. By harnessing the power of generative AI, they're making it possible to create novel enzymes with specific properties.
The Intelligent Architecture platform is a testament to the capabilities of generative AI in synthetic biology. It's an exciting development that's sure to have a significant impact on the field.
A unique perspective: Generative Ai Architecture Diagram
Simulating Biological Processes
Generative AI can be used to create realistic simulations of biological processes, such as cellular signaling or metabolic pathways, helping researchers understand and predict their behavior.
These simulations can be incredibly detailed, allowing researchers to model complex interactions and test hypotheses in a controlled environment. This can save time and resources compared to traditional experimental methods.
Google Deepmind's AlphaProteo is an example of how generative models can be used to design novel proteins that bind to target molecules, which can be useful in various fields such as medicine and biotechnology. This technology has the potential to revolutionize the way we approach protein engineering and design.
By simulating biological processes, researchers can gain a deeper understanding of how living systems work, which can lead to breakthroughs in fields like medicine and biotechnology. This knowledge can also be used to develop new treatments and therapies for various diseases.
Generative models can create novel protein sequences with specific functionalities or properties, which can be used to design new enzymes or therapeutics. This can be a game-changer for fields like biotechnology and medicine.
These simulations can also be used to generate synthetic patient and healthcare data, which can be used to train AI models and simulate clinical trials. This can help researchers study rare diseases and develop new treatments without access to large real-world datasets.
By using generative AI to simulate biological processes, researchers can gain a better understanding of complex systems and develop new treatments and therapies. This technology has the potential to transform various fields and improve human health.
On a similar theme: New Generative Ai
Companies and Solutions
Evozyne is a company that integrates engineering and deep learning techniques to develop highly functional synthetic proteins. They use the NVIDIA BioNeMo framework to create ProT-VAE, a transformer-based model for protein engineering.
Peptilogics' Nautilus generative AI platform facilitates peptide drug design and lead optimization across various therapeutic fields and biological targets. Powered by the company's custom-built N4 supercomputer, Nautilus incorporates proprietary peptide representation and generative algorithms.
Evozyne works on various bioengineering use cases, including gene optimization, gene editing, and generating therapeutic antibodies. These use cases demonstrate the potential of generative AI in life sciences.
Here are some specific examples of the types of bioengineering use cases that Evozyne works on:
- Gene optimization
- Gene editing
- Generating therapeutic antibodies
Evozyne
Evozyne is a company that's making waves in the field of bioengineering. They're using a combination of engineering and deep learning techniques to develop synthetic proteins that are highly functional.
Their approach involves utilizing the NVIDIA BioNeMo framework to create a transformer-based model called ProT-VAE, specifically designed for protein engineering. This allows them to expedite the creation of new proteins.
Evozyne is working on a range of bioengineering use cases, including gene optimization and gene editing. They're also using their technology to generate therapeutic antibodies.
Here are some specific areas where Evozyne is applying their expertise:
- Gene optimization
- Gene editing
- Generating therapeutic antibodies
Peptilogics
Peptilogics is a company that has developed a powerful AI platform for designing peptide drugs. The Nautilus platform is a key part of their technology.
This platform is built on a custom supercomputer called N4, which uses NVIDIA GPUs to speed up the design process.
The Nautilus platform can help reduce the costs, time, and risks associated with traditional drug design methods.
Quantiphi: Leading Integration
Quantiphi is a leading company that integrates generative AI into the life sciences sector. They offer customized AI solutions that adhere to the highest standards of ethical AI practices and data security.
Quantiphi's unique approach is grounded in a deep understanding of each client's specific needs, enabling them to craft AI solutions that are both cutting-edge and aligned with industry standards. This commitment to excellence is further bolstered by their strategic partnerships with technology leaders like Amazon Web Services (AWS), Google Cloud Platform (GCP), and NVIDIA.
Quantiphi's partnerships with AWS, GCP, and NVIDIA underscore their capability to deliver transformative AI solutions in life sciences.
For your interest: Generative Ai Solutions
Commercialization
Commercialization is a crucial step in bringing a new drug to market, and generative AI can play a significant role in this process. It can help life science firms acquire new clients and close deals more efficiently. Gen AI-powered sales assistants can craft customized pitches and support human sales forces by providing the right information at the right time.
Gen AI models can even help prepare for deal negotiation, model negotiation scenarios, and draft contracts. This can be a game-changer for life science companies looking to expand their customer base. By automating some of the sales process, companies can focus on high-value tasks and build stronger relationships with their clients.
Marketing content generation is another area where generative AI excels. It can help life science companies create customized marketing material that is both informative and compliant with pharma regulations. AI-driven marketing assistants can generate campaign ideas and assemble marketing materials, freeing up human resources for more strategic tasks.
Explore further: Generative Ai Company
Here are some specific examples of how Gen AI can be used in marketing content generation:
- Generating campaign ideas and assembling customized marketing material
- Creating medical articles that are easy to read
- Fact-checking and editing existing copies
- Ensuring marketing material abides by industry regulations
Market surveillance is also an important aspect of commercialization, and generative AI can be used to monitor social media and pharma websites for customer feedback on new drugs. This can help companies spot any side effects and flag them for investigation. Additionally, Gen AI can be used to analyze competitor intelligence and assess the performance of similar medicines.
If this caught your attention, see: Chatgpt Openai's Generative Ai Chatbot Can Be Used for
Frequently Asked Questions
How can generative AI be used in healthcare?
Generative AI helps healthcare professionals analyze complex genetic data, identifying specific markers for personalized care. It enables tailored treatment plans, improving patient outcomes and quality of life
What is generative AI in biology?
Generative AI in biology uses artificial intelligence to create new insights, data, and solutions from existing biological information. This innovative approach is revolutionizing the field of life sciences by uncovering new knowledge and possibilities
What is the estimated value potential for Gen AI in healthcare and life sciences?
Gen AI is estimated to unlock $5-7 billion in value for life sciences companies, primarily through research, manufacturing, and commercial applications
Sources
- https://www2.deloitte.com/us/en/pages/life-sciences-and-health-care/articles/gen-ai-life-sciences.html
- https://research.aimultiple.com/generative-ai-in-life-sciences/
- https://quantiphi.com/the-next-leap-in-healthcare-how-generative-ai-is-reshaping-life-sciences/
- https://www.sikich.com/insight/navigating-the-potential-of-generative-ai-in-life-sciences/
- https://itrexgroup.com/blog/generative-ai-in-life-sciences-use-cases/
Featured Images: pexels.com