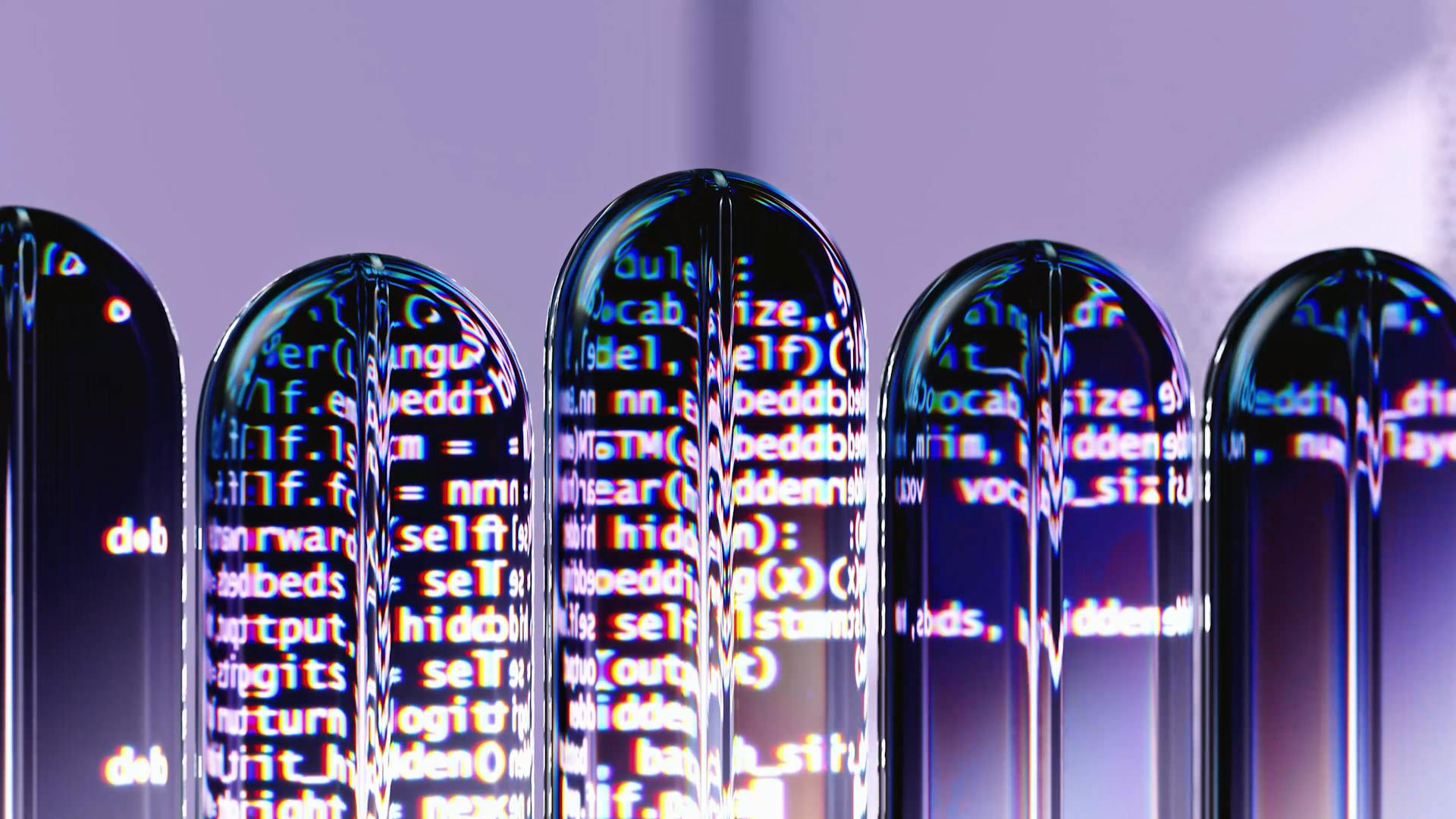
Generative AI in manufacturing is transforming industry operations by streamlining production processes and improving product quality.
With the ability to generate complex designs and simulations, generative AI can reduce the need for physical prototypes and minimize waste. Generative AI can also optimize production workflows, reducing production times and increasing efficiency.
Manufacturers are leveraging generative AI to create customized products and improve supply chain management. For instance, generative AI can help predict demand and optimize inventory levels.
By automating routine tasks and enabling data-driven decision-making, generative AI is freeing up manufacturers to focus on innovation and growth.
Benefits and Applications
Generative AI in manufacturing offers numerous benefits and applications that can revolutionize the industry. One of the main benefits is the automation of factory operations, enabling fully automated factories and streamlining processes.
Generative AI can identify inefficiencies and offer valuable insights across all aspects of manufacturing, from production to the supply chain and client interactions. This technology represents the future of smart manufacturing or industry 4.0 by maintaining high productivity and efficiency levels.
Readers also liked: Generative Ai in Chemical Industry
Generative AI enables manufacturers to automate the optimization of product designs, reducing the weight of aircraft partition walls by 45% while maintaining structural integrity, as seen in Airbus' example. This is achieved by inputting constraints and parameters, and AI algorithms generate a multitude of design variations.
AI-driven simulations predict the behavior of materials under diverse conditions, facilitating identifying weaknesses, optimizing product performance, and reducing material waste. General Electric (GE) employs AI-based simulations to enhance turbine blade designs, resulting in more efficient and reliable engines.
AI algorithms generate synthetic data that mimics real-world manufacturing scenarios, aiding in training AI models and enhancing their performance. Ford utilizes synthetic data to train autonomous vehicle systems, enabling safe and efficient testing in virtual environments.
Pre-trained AI models, known as foundation models, automate and streamline various manufacturing tasks, providing actionable insights for informed decision-making. Siemens leverages foundation models to optimize production processes, improve product quality, and enhance supply chain management.
Curious to learn more? Check out: Generative Ai in Performance Testing
Use Cases
Generative AI has the potential to revolutionize manufacturing by reducing time to market and leveraging digital twin technology. It can also drive innovation, enable predictive maintenance, and enhance decision-making.
In the manufacturing sector, generative AI offers a wide range of use cases that can improve efficiency and innovation. From accelerating time to market to harnessing digital twin technology, generative AI has diverse applications and transformative capabilities.
The adoption of generative AI in manufacturing becomes increasingly compelling due to the pressure to enhance production processes, optimize supply chains, and deliver high-quality products to customers. To overcome these challenges, manufacturers can integrate generative AI capabilities into their operations.
One example of generative AI in manufacturing involves using generative models to generate fake data for training machine learning algorithms. This approach can significantly reduce costs and time needed for data collection and labeling processes.
Generative AI can also be used to reimagine product design and development, leading to superior and more innovative outcomes. It can explore uncharted design territories and introduce novel solutions that might have remained hidden or overlooked.
A fresh viewpoint: Roundhill Generative Ai & Technology Etf
Time to Market and Innovation
Generative AI in manufacturing is revolutionizing the way products are designed, developed, and brought to market. By automating and optimizing various stages of product development, manufacturers can significantly reduce the time it takes to launch a new product.
With generative AI, manufacturers can analyze data from sensors and equipment to predict maintenance needs, extending the equipment's lifespan and reducing unplanned maintenance costs.
The innovation process is also being transformed by generative AI, which can analyze vast amounts of data to identify patterns and generate new ideas. This can help manufacturers uncover innovative product designs, optimize manufacturing processes, and even discover new business models.
Manufacturers can use generative AI to innovate in product design, optimize manufacturing processes, and explore new business opportunities, maintaining a competitive edge in the market.
Readers also liked: New Generative Ai
Digital Transformation and Efficiency
Digital transformation and efficiency are key benefits of generative AI in manufacturing. By using digital twins, manufacturers can create accurate virtual replicas of their products, production lines, or entire factories, allowing for simulations and predictive analysis to optimize operations and identify potential issues.
With generative AI, manufacturers can automate repetitive tasks such as inventory management, supply chain operations, and order processing, freeing up resources for strategic and value-adding activities. This automation enables manufacturers to complete tasks in no time, with more consistency and error-free.
Generative AI also helps manufacturers streamline their production planning and scheduling, reducing inaccuracies and costs associated with inefficient inventory management. By analyzing historical sales data and market trends, generative AI can provide valuable insights and recommendations for optimized production planning and resource allocation.
Broaden your view: Generative Ai and Knowledge Management
Productivity and Efficiency
Generative AI has emerged as the conductor of modern manufacturing, ensuring that the production process runs smoothly by analyzing data from diverse sources and identifying patterns and bottlenecks. This results in a manufacturing process fine-tuned to perfection, minimizing waste and maximizing output.
With Generative AI, manufacturers can automate repetitive tasks such as inventory management, supply chain operations, order processing, quality inspection, and customer interactions, freeing up resources for strategic and value-adding activities. This automation enables tasks to be completed quickly, consistently, and with fewer errors.
Check this out: Which Term Describes the Process of Using Generative Ai
Generative AI can analyze vast amounts of product quality and visual inspection data, allowing manufacturers to identify defects in real-time and make immediate adjustments to prevent waste. This capability ensures an immediate jump in the rectifying process, significantly reducing waste and improving product consistency.
By leveraging machine learning algorithms, Generative AI can analyze historical data from various sources, including inventory management, market trends, and customer feedback, providing valuable insights and recommendations for strategic decision-making. This data-driven approach enables manufacturers to optimize production planning, identify cost-saving opportunities, and improve overall business performance.
Generative AI can also optimize production data analysis, process optimization, and relevant measure approvals, allowing for continuous monitoring and adaptation to changing circumstances. This results in minimized resources and improved operational efficiency.
By creating digital twins—virtual replicas of physical assets or processes—Generative AI enables manufacturers to create accurate digital representations of their products, production lines, or entire factories. These digital twins can be used for simulations and predictive analysis, allowing manufacturers to optimize operations, identify potential issues, and test different scenarios without disrupting the production process.
Generative AI can also help manufacturers achieve optimal production schedules, ideal resource allocation, and workflow optimization, making production and inventory management smooth and effective. This results in reduced cost overruns and improved efficiency in the manufacturing industry.
A unique perspective: Telltale Words Identify Generative Ai Text
Workforces Skills
As manufacturing workflows become increasingly digital, the skills gap between the workforce and technology adoption is a significant challenge. This gap is caused by the continuous upgrading of technology, which creates a disconnect between the skills required to implement it and the knowledge of the workforce.
Manufacturers need to invest in comprehensive training programs to bridge this gap and ensure their workforce is proficient in leveraging AI tools. This includes providing reskilling opportunities to mitigate the impact of job displacement and workforce restructuring.
Generative AI models can aid in creating synthetic training data to upskill the manufacturing workforce. This enables frontline workers to make informed decisions with data-driven insights and recommendations.
Here are some ways generative AI models can support workforce skills:
- Creating synthetic training data to upskill the manufacturing workforce
- Automating report generation, providing data-driven insights and recommendations
- Generating interactive learning materials like virtual simulations
By leveraging these capabilities, manufacturers can empower their workforce to make the most of digital transformation and drive efficiency in their operations.
Operational, Reputational, Regulatory Risks
Manufacturers must navigate various risks associated with GenAI adoption, including compliance with industry regulations, operational challenges, reputational risks, and evolving regulatory frameworks. Ensuring AI systems comply with existing regulations and industry standards is essential to avoid legal consequences and maintain operational integrity.
A different take: Impact of Generative Ai on Tax Industry
Operational risks such as AI system malfunctions leading to production delays or quality issues must be mitigated through rigorous testing and monitoring.
Manufacturers must ensure that AI systems do not compromise the reliability of their conclusions due to inherent biases in the models.
Compliance with industry regulations is crucial to avoid legal consequences and maintain operational integrity.
Manufacturers must proactively manage operational risks to maximize the benefits of AI adoption while mitigating risks.
Expand your knowledge: Risks of Generative Ai
Supply Chain and Logistics
Supply Chain and Logistics is a critical spoke in the wheel of manufacturing success. The Global Generative AI In Manufacturing Market size is expected to be worth around USD 6,397.4 Million by 2033, growing at a CAGR of 41.10% during the forecast period from 2024 to 2033.
Generative AI is transforming supply chain logistics and demand forecasting, resulting in reduced lead times, lower inventory costs, and impressive success stories for companies embracing AI-driven optimization. It uses advanced algorithms to predict demand patterns, enabling companies to adjust their production schedules and inventory levels accordingly.
By leveraging the power of ML, analyzing historical data to forecast demand and enabling manufacturers to coordinate distribution channels is a breeze. This proactive approach minimizes the risk of overstocking or understocking, optimizing inventory costs.
Expand your knowledge: How Are Companies Using Generative Ai
Predictive Maintenance
Predictive maintenance is a game-changer for supply chain and logistics, allowing manufacturers to detect potential equipment failures before they occur.
By analyzing real-time data from sensors and equipment, generative AI algorithms can detect patterns and anomalies that indicate maintenance needs, enabling proactive scheduling and minimizing downtime.
Manufacturers can optimize maintenance schedules, leading to increased operational efficiency and cost savings, thanks to predictive maintenance.
Generative AI can automate data analysis and generate synthetic datasets, addressing the challenges of large volumes of data and lack of data science expertise.
Predictive maintenance powered by generative AI helps the manufacturing industry minimize downtime and optimize maintenance processes through well-planned scheduling.
This approach not only improves equipment reliability but also enables manufacturers to achieve effective cost-cutting.
With predictive maintenance, manufacturers can schedule maintenance at precisely the right moment, preventing unplanned downtime that can dent both productivity and profitability.
Supply Chain Management
Supply Chain Management is a complex process that requires careful planning and execution. Generative AI is revolutionizing supply chain management by providing real-time insights into ongoing supply chain performance.
Additional reading: Generative Ai for Data Management
With GenAI, manufacturers can gain visibility across interconnected parts of the supply chain. This includes analyzing historical interactions with suppliers to strengthen relationships and recommending suppliers based on factors like raw material availability, pricing, and potential risks.
Supply chain disruptions can have significant consequences, but GenAI helps mitigate these risks by identifying potential issues before they become major problems.
GenAI's ability to analyze data and generate content in multiple formats makes it an invaluable tool for supply chain management. This includes recommending and selecting suppliers, as well as providing real-time insights into supply chain performance.
By leveraging GenAI, manufacturers can ensure smooth transactions and make more informed decisions. This is critical for business success, especially in today's fast-paced and competitive market.
The Global Generative AI In Manufacturing Market is expected to be worth around USD 6,397.4 Million by 2033, growing at a CAGR of 41.10% during the forecast period from 2024 to 2033. This growth is driven in part by the increasing adoption of GenAI in supply chain management.
Streamlining supplier evaluation and selection based on data evaluation is just a click away with GenAI. This is a significant advantage for manufacturers looking to optimize their supply chain operations.
With GenAI, manufacturers can analyze historical data to forecast demand and enable coordination of distribution channels. This is a major step forward in supply chain management, allowing companies to respond quickly to changing market conditions.
Consider reading: Generative Ai in Supply Chain
Future and Evolution
The future of generative AI in manufacturing is highly promising, with numerous benefits and use cases being explored. It has the potential to revolutionize processes and drive innovation.
With its ability to provide instant insights, genAI can smoothen out end-to-end production flows, foresee equipment failures before time, and monitor quality by detecting anomalies. GenAI can also supply custom experiences for buyers, giving businesses a competitive edge.
As genAI evolves, it will be increasingly integrated into different parts of manufacturing, driving efficiency and productivity.
Preparing for Regulatory Evolution
Regulatory landscapes are evolving to address the complexities of AI technologies. Manufacturers must stay informed about regulatory developments and adapt their AI strategies accordingly.
The EU's proposed AI Act highlights the need for comprehensive regulations specific to generative AI to address emerging risks and ensure responsible AI deployment. This emphasizes the importance of understanding regulatory requirements for GenAI.
Initiatives like the EU's proposed AI Act require manufacturers to adopt a proactive approach to risk management and compliance. This involves addressing the benefits and risks of GenAI adoption.
Manufacturers can harness the transformative power of GenAI while navigating challenges effectively by implementing robust strategies for risk management and compliance. Downloading the infosheet "4 Key Considerations for Generative AI in Manufacturing" can help ensure AI initiatives align with industry best practices and regulatory requirements.
OAuth 2.0 is the industry-standard protocol for authorization, allowing users to grant third-party application access to protected resources without revealing long-term credentials. This is essential for secure GenAI deployment.
Discover more: Generative Ai in Tourism
The Future of
GenAI is poised to transform the manufacturing industry profoundly, with its ability to analyze vast amounts of data and generate valuable insights. This technology has the potential to revolutionize processes and drive innovation.
One significant benefit of GenAI is its ability to provide instant insights, optimize processes, and drive innovation. This positions it as a catalyst for competitive advantage.
However, like any emerging technology, GenAI adoption in manufacturing presents inherent risks and challenges. One significant concern is the potential for inaccurate or biased outcomes if the underlying algorithms and machine learning models are not meticulously trained and validated.
Here's an interesting read: The Economic Potential of Generative Ai
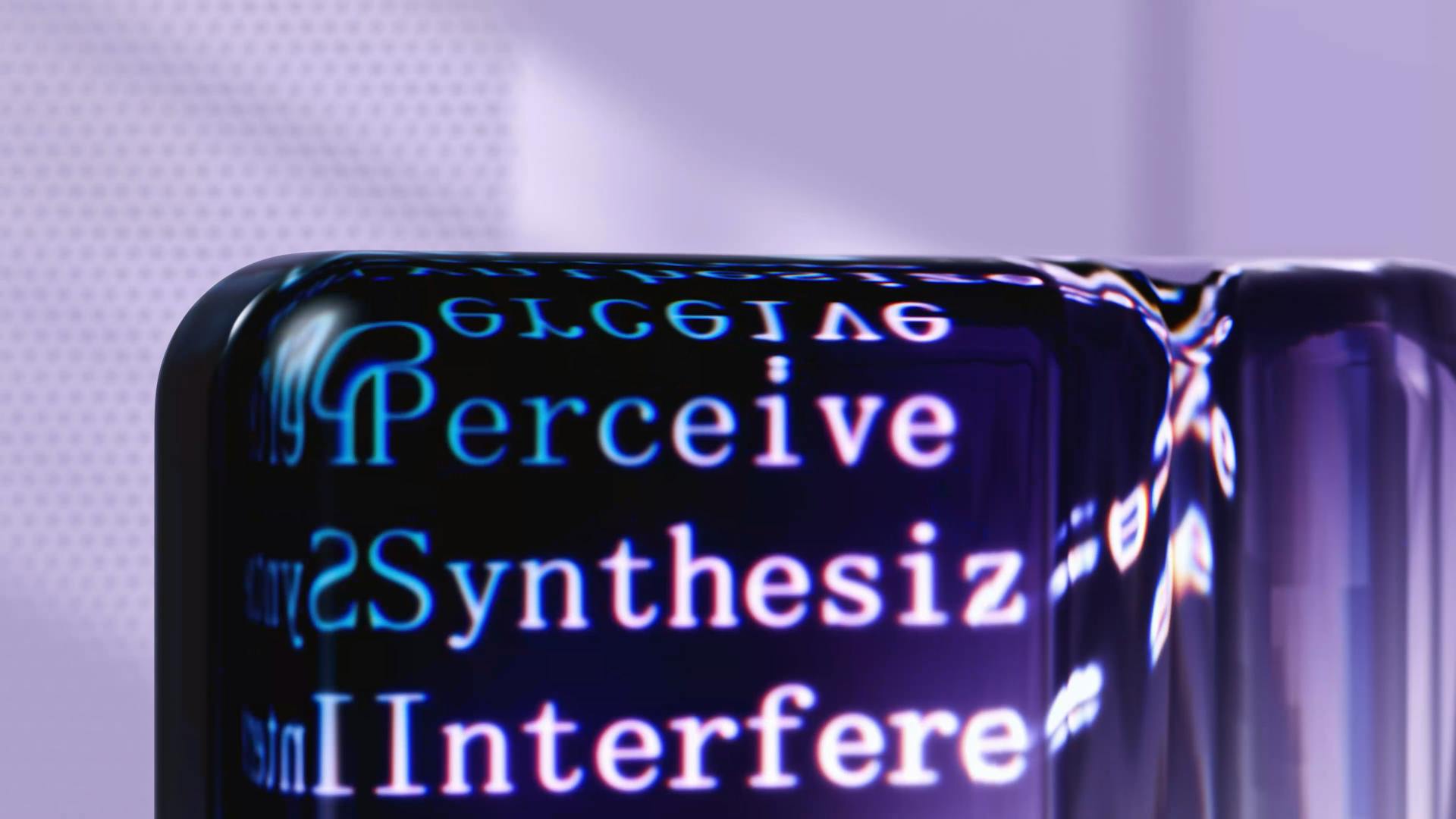
Through rigorous training and validation of AI models, manufacturers can mitigate risks associated with data accuracy and bias. This can be achieved by properly training and validating algorithms and machine learning models.
The future impact of GenAI on manufacturing is enormous, with the potential to smoothen out end-to-end production flows, foresee equipment failures, monitor quality, and provide custom experiences for buyers. This integration will drive efficiency, productivity, and innovation.
Here are some of the potential benefits of GenAI in manufacturing:
- Instant insights
- Optimized processes
- Driven innovation
- Competitive advantage
- Efficiency
- Productivity
- Innovation
Intelligent Integration
Integrating Generative AI with existing manufacturing systems can be complex and time-consuming, potentially leading to disruptions in operations and impacting productivity.
Many manufacturing processes have been in place for years and may not easily accommodate AI technologies without significant modifications or infrastructure overhauls.
The integration process can be complex due to the need for substantial initial investment, including expenses related to acquiring AI systems, upgrading infrastructure, and implementing necessary hardware and software.
Readers also liked: Generative Ai Infrastructure
To effectively incorporate GenAI within manufacturing workflows, organizations should follow a systematic structure, starting with a thorough analysis to identify inefficiencies, bottlenecks, and quality issues.
Here's a step-by-step process to consider:
- Evaluate existing processes and workflow to identify pain points and determine how GenAI can address them.
- Pre-determine specific use cases by prioritizing manufacturing workflows and tasks that can benefit most from GenAI integration.
- Gather and prepare data by identifying relevant data sources and ensuring data quality, cleanliness, and standardization.
- Select and develop GenAI models by evaluating and choosing the appropriate algorithms and architectures.
- Integrate GenAI into manufacturing workflows by embedding the trained models into relevant processes.
- Monitor and continuously improve by establishing robust feedback mechanisms to track performance and analyze outcomes.
By following this structured approach, manufacturers can unlock significant benefits in the forms of productivity, efficiency, and cost savings.
Sources
- https://www.alpha-sense.com/blog/trends/generative-ai-manufacturing/
- https://www.ideas2it.com/blogs/generative-ai-for-manufacturing
- https://www.rishabhsoft.com/blog/generative-ai-in-manufacturing
- https://censius.ai/blogs/generative-ai-use-cases-in-the-manufacturing-industry
- https://www.softserveinc.com/en-us/generative-ai/manufacturing
Featured Images: pexels.com