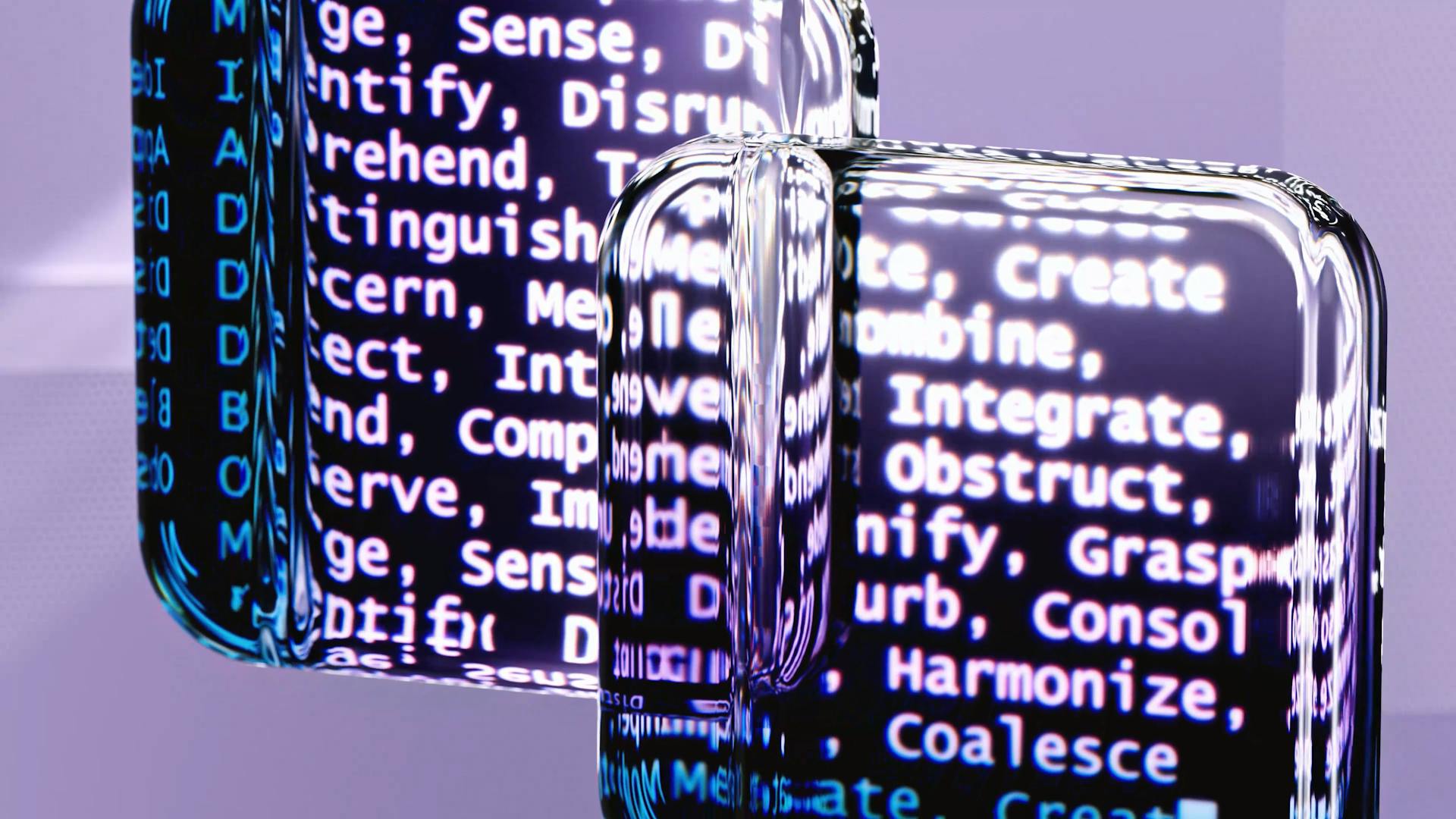
Generative AI in financial services is revolutionizing the way institutions operate. By leveraging this technology, banks and financial organizations can unlock new use cases that improve customer experience and drive business growth.
One key area where generative AI is making a significant impact is in customer service. A study found that 80% of customers prefer to interact with chatbots rather than human customer support agents, especially for routine inquiries.
This shift towards digital customer support is not only cost-effective but also allows financial institutions to provide 24/7 support to their customers. By automating routine tasks, customer support agents can focus on more complex and high-value tasks that require human expertise.
As a result, customers can get their queries resolved faster and with more accuracy, leading to increased customer satisfaction and loyalty.
For your interest: Generative Ai Customer Service
Automated Accounting Processes
Automated accounting processes are a game-changer in the financial services industry. Generative AI can automate routine accounting tasks, such as data entry, reconciliation, and categorization of financial transactions. This reduces manual effort and minimizes errors, increasing efficiency and accuracy in financial record-keeping.
Explore further: Generative Ai in Accounting
According to a study, generative AI can achieve high rates of automation in most accounting tasks, thanks to its deep learning functions. This means that finance units can rely on AI to automate functions such as auditing, accounts payable, and invoice capture and processing.
Generative AI can also help with dynamic data validation and cleanup, proactively correcting errors and ensuring higher data quality and reliability for financial operations. This is particularly useful for tasks that were previously labor-intensive, such as document management workflows.
Here are some examples of how generative AI can automate accounting processes:
- Automated document processing: Generative AI can understand context, extract relevant information, and summarize content, streamlining document management workflows.
- Intelligent customer interaction: Generative AI can generate personalized customer responses, including drafting tailored emails or chat responses.
- Data validation and cleanup: Generative AI can analyze patterns and learn from data inconsistencies, ensuring higher data quality and reliability for financial operations.
Conversational
Conversational finance is revolutionizing the way financial institutions interact with their customers. Generative AI models can produce more natural and contextually relevant responses, enhancing the performance and user experience of financial conversational AI systems and chatbots.
By providing customers with improved customer support, personalized financial advice, payment notifications, and document generations, conversational finance is making a significant impact. For instance, Morgan Stanley employs OpenAI-powered chatbots to support financial advisors.
Discover more: Generative Ai in Finance
Conversational finance can also provide customers with a range of benefits, including:
- Improved customer support
- Personalized financial advice
- Payment notifications
- Document generations like investment summaries or loan applications.
Generative AI-powered chatbots can cater to a broad spectrum of customer needs, from simple account inquiries to providing complex financial advice. These chatbots can analyze and understand individual customer preferences and history, tailoring their assistance and advice in real-time.
Financial institutions have a variety of tools at their disposal to develop a custom AI chatbot, including Chatbase, Botsonic, and CustomGPT.ai.
Broaden your view: Generative Ai Customer Experience
Credit and Risk Management
Credit and Risk Management is a critical area where generative AI is making a significant impact. AI models can generate synthetic borrower profiles to test the robustness of credit risk models, improving the accuracy of credit scoring and default predictions.
Generative AI in finance enables sophisticated portfolio optimization and risk management by analyzing historical data, market trends, and risk factors. This helps financial institutions make data-driven decisions to maximize returns while minimizing risk exposure.
Credit risk assessment is a prominent use of generative AI in finance. Generative AI algorithms can analyze diverse data sources, including credit history, financial statements, and economic indicators, to assess credit risk for individual borrowers or businesses.
For more insights, see: Generative Ai for Product Managers
AI credit scoring transforms traditional credit evaluation methods by leveraging machine learning algorithms to digest a vast array of data points, including unconventional ones, to forecast an applicant's loan repayment capabilities. This approach results in an AI-generated score that offers a richer, more precise insight into an individual's financial conduct.
Generative AI can also identify potential credit risks by analyzing credit history, statements of every relatable financial document, and economic indicators. This enables creditors to make more informed decisions regarding loan approvals, interest rates, and credit limits.
Here are some key benefits of using generative AI in credit and risk management:
- Improved credit risk modeling: Generative AI refines the accuracy of key credit risk metrics such as probability of default (PD), loss given default (LGD), and exposure at default (EAD).
- Enhanced stress testing: GenAI enables the creation of diverse, challenging scenarios for stress testing, helping financial institutions better prepare for adverse conditions.
- Advanced model validation and bias reduction: By utilizing synthetic data, Gen AI improves the process of model validation, reduces biases, and enhances the transparency of credit scoring models.
In practice, generative AI has been used by financial institutions to analyze vast amounts of data and make more accurate predictions about credit risk. For example, a UK high street bank leveraged Kortical's platform to build a machine learning model that beat traditional credit scoring, predicting credit default better and catching 83% of bad debt not caught by credit score.
Automated Reporting
Automated reporting is a game-changer in the financial services industry. Generative AI can automatically create well-structured, coherent, and informative financial reports based on available data, including balance sheets, income statements, and cash flow statements.
This automation streamlines the reporting process, reduces manual effort, and ensures consistency, accuracy, and timely delivery of reports. It's a win-win for financial institutions and stakeholders alike.
Here are some benefits of automated reporting:
- Balance sheets
- Income statements
- Cash flow statements
The SEC in the U.S. has been ratcheting up its use of machine learning since the global financial crisis, using decisions by machine learning models to guide regulators to entities whose behavior required more detailed analysis. This approach, known as 'keeping the human in the loop', takes advantage of the power of machine learning while maintaining a level of human accountability.
Check this out: Generative Ai Human Creativity and Art Google Scholar
Financial Services
Generative AI is transforming the financial services industry in exciting ways. Banks are leveraging its power to provide personalized banking services that cater to individual customers' needs.
If this caught your attention, see: Generative Ai Consulting Services
According to a Salesforce Research survey, 84% of customers want to be treated as individuals, not just numbers, in winning their business. This is where generative AI comes in, enabling banks to offer tailored financial advice, investment strategies, and savings plans that align with individual goals and risk tolerances.
Dynamic financial planning, personalized product recommendations, and customized customer profiles are just a few examples of how generative AI is being used to provide a more personalized banking experience. For instance, the Cleo App connects to bank accounts and gives clients proactive advice and information on their finances.
Here are some benefits of generative AI in the finance industry:
Banking Basics
Generative AI in banking and finance can find transaction anomalies, which is a crucial aspect of fraud detection. This technology helps identify suspicious activities and prevents financial losses.
In the context of banking, generative AI can assist in conversational finance through chatbots. These chatbots can help customers with their queries and provide them with personalized financial advice.
Check this out: Are Chatbots Generative Ai
Financial forecasting is another area where generative AI can be of great help. It can analyze large datasets and make predictions about future market trends. This can enable businesses and individuals to make informed investment decisions.
Synthetic data can be used to ensure data privacy in banking and finance. This is particularly useful when dealing with sensitive customer information.
Risk management is also an area where generative AI can be applied. It can help identify potential risks and provide recommendations on how to mitigate them.
Banking Overview
The banking industry is undergoing a significant transformation with the help of generative AI. This technology has the potential to redefine traditional methods and bring more informative and realistic scenarios, making impressive portfolios, better-optimizing strategies, and enabling superior risk handling and fraud detection.
Generative AI is being used in various areas of banking, including personalized banking services. According to a recent Salesforce Research survey, 84% of customers emphasize the importance of being treated as individuals, not just numbers, in winning their business. This is where generative AI comes in, providing tailored financial advice, investment strategies, and savings plans that align with individual goals and risk tolerances.
For more insights, see: Generative Ai in Financial Services
Dynamic financial planning is one of the personalized services that generative AI offers. This involves analyzing a customer's financial situation to suggest the most suitable banking products, such as savings accounts, investment funds, or loans. This targeted approach simplifies the product selection process, significantly enhancing the likelihood of product adoption.
Customized customer profiles are also being created using generative AI. This enables banks to provide customized advice and services, fostering a stronger, more personalized customer-bank relationship. The Cleo App is a great example of this, connecting to bank accounts and giving clients proactive advice and information on their finances.
Several banks are already using generative AI, including Morgan Stanley, Bank of America, and Wells Fargo. Morgan Stanley uses OpenAI-powered chatbots, while Bank of America and Wells Fargo have integrated conversational AI into their systems. Deutsche Bank collaborates with Google Cloud's generative AI.
The benefits of generative AI in the finance industry are numerous. It improves financial conditions by providing efficient research, business partnering, and risk mitigation. For example, EY claims that investment banking experiences more efficient research and financial modeling, while corporate and SMB banking gains from improved risk management and business lending.
Here are some key benefits of generative AI in the finance industry:
- Improved financial condition
- Efficient research
- Business partnering
- Mitigating risks
Wells Fargo's Predictive Banking Feature is a great example of how generative AI can be used to provide personalized banking services. This feature allows access to more than 50 prompts related to past and future account activity. By analyzing large volumes of data, generative AI can also improve the accuracy of financial forecasts, including stock prices, interest rates, and economic indicators.
You might enjoy: Key Features of Generative Ai
Portfolio Management Solutions
Portfolio management solutions are becoming increasingly sophisticated thanks to generative AI. Generative AI models can analyze historical financial data and generate various investment scenarios to help asset managers and investors identify optimal asset and wealth management.
By considering factors such as risk tolerance, expected returns, and investment horizons, generative AI can provide personalized investment portfolios tailored to individual investor profiles, preferences, and financial goals.
Generative AI can simulate different market conditions, economic environments, and events to better understand the potential impacts on portfolio performance. This allows financial professionals to develop and fine-tune their investment strategies, optimize risk-adjusted returns, and make more informed decisions about managing their portfolios.
Generative AI can also help financial institutions make data-driven decisions to maximize returns while minimizing risk exposure. This is achieved by analyzing historical data, market trends, and risk factors.
Here are some key benefits of generative AI in portfolio management:
- Risk tolerance
- Expected returns
- Investment horizons
- Customized investment portfolios
- Improved financial outcomes
By leveraging generative AI, financial professionals can develop more effective investment strategies, optimize risk-adjusted returns, and provide better financial outcomes for their clients or institutions.
Market Predictions
Generative AI can improve the accuracy of financial forecasts, including stock prices, interest rates, and economic indicators. By analyzing large volumes of data, generative AI can help predict market trends and make more informed investment decisions.
The banking industry is expected to spend $84.99 billion on generative AI by 2030, growing at a remarkable compound annual growth rate of 55.55 percent. This investment will likely lead to significant improvements in market predictions and financial forecasting.
J.P. Morgan is investing $17 billion in generative AI this year, a 10% increase from $15.5 billion in 2023. This investment will focus on finding applications for generative AI in various business verticals.
A fresh viewpoint: Generative Ai Market
Regulatory Compliance
Generative AI is being used by banks to respond to simple and less critical queries from regulators, freeing up staff to focus on more complex issues. This is a result of proof-of-concepts (PoCs) being run to see if LLMs can be used for this purpose.
On a similar theme: How Multimodal Used in Generative Ai
Banks are also leveraging generative AI to analyze vast amounts of regulatory data and provide insights to organizations on how to adapt to regulatory code changes efficiently. This helps businesses stay compliant and mitigate regulatory risks effectively.
Citi used AI to analyze 1,089 pages of new capital rules on the U.S. banking sector, assessing the impact of the plans and determining how much capital the lender has to set aside to guard against future losses. This is just one example of how generative AI is being used to streamline compliance processes.
To enhance operational efficiency, ensure regulatory adherence, and proactively mitigate risks, financial institutions are increasingly leveraging generative AI. This technology excels in analyzing vast data sets more efficiently and accurately than human capabilities allow, identifying patterns and anomalies indicative of risk or fraudulent activities, and ensuring compliance with regulatory mandates more effectively.
Generative AI can be used for various compliance and risk management tasks, including AI-Powered chatbots for compliance queries, regulatory document analysis, automated compliance reporting, and risk identification.
Explore further: Risks of Generative Ai
Challenges in Industry & Solutions
In the financial industry, generative AI can be a powerful tool for regulatory compliance, but it also comes with its own set of challenges. One major concern is data accuracy, as the European Central Bank notes that AI can be prone to significant data quality issues.
Data quality and accuracy are crucial factors when feeding foundation models, as biased and inaccurate data can lead to output with more errors. This is a critical issue in finance, where decisions rely on precise data.
Another challenge is bias in models, which can inherit biases from the data they are trained on, leading to unfair or skewed decisions. This is particularly concerning in areas like credit scoring or investment recommendations.
Limited generalization is also a problem, as off-the-shelf large language models may not perform well in specific, highly specialized financial contexts without proper fine-tuning. This can result in inaccurate or irrelevant outputs.
Generative AI can also produce inaccurate or fabricated information, known as hallucinations, which is risky in finance where decisions rely on precise data.
Take a look at this: Generative Ai Photoshop Increase Quality
The financial sector is highly regulated, and AI must comply with strict standards regarding transparency, accountability, and data usage. This poses challenges in ensuring compliance with evolving legal frameworks.
To mitigate these risks, it's essential to ensure data security, as financial data is sensitive and must be handled securely to maintain client trust and avoid regulatory penalties.
Here are some key risks associated with LLMs in finance:
- Data accuracy issues
- Bias in models
- Limited generalization
- Hallucinations
- Regulatory challenges
- Data security risks
By understanding these challenges and taking steps to address them, financial institutions can harness the power of generative AI for regulatory compliance while minimizing the risks associated with its use.
Compliance Management
Compliance management is a crucial aspect of regulatory compliance, and generative AI is revolutionizing the way financial institutions approach it. Banks are using AI to respond to simple and less critical queries from regulators.
Financial institutions face high stakes when it comes to managing security risks and compliance with local and federal regulations. They're leveraging generative AI to analyze vast data sets more efficiently and accurately than human capabilities allow.
Generative AI can analyze vast amounts of regulatory data and provide insights to organizations on how to adapt to regulatory code changes efficiently. This helps businesses stay compliant and mitigate regulatory risks effectively.
Citi used AI to analyze 1,089 pages of new capital rules on the U.S. banking sector, assessing the impact of the plans and determining how much capital the lender has to set aside to guard against future losses.
Here are some potential applications of Gen AI within compliance and risk management:
- AI-Powered chatbots for compliance queries: Deploy AI chatbots and virtual assistants to address routine compliance questions from both employees and customers.
- Regulatory document analysis: Utilize generative AI for rapid regulatory and financial document search, analysis, and summarization.
- Automated compliance reporting: Employ generative AI to draft compliance reports by analyzing financial data from compliance monitoring systems.
- Risk identification: Apply generative AI to scrutinize customer feedback and social media for potential conduct risks and compliance issues.
Scenario-Based Solutions
Scenario-based solutions are a game-changer in financial services, and generative AI is at the forefront of this innovation. By simulating various market scenarios, these models can help traders and portfolio managers understand potential risks and returns under different conditions.
According to Dimension Market Research, the global market for generative AI in trading is projected to grow at a compound annual growth rate (CAGR) of 26.3% in 2024, reaching USD 208.3 million. By 2033, the market is expected to reach USD 1,705.1 million.
Recommended read: Generative Ai Market Research
Generative AI can create different regulatory scenarios and generate reports to help financial institutions ensure they meet all necessary compliance requirements under various conditions. This is particularly useful in anomaly detection, where generative AI models can be trained to understand normal patterns of transactions and identify potential outliers or anomalies.
Here are some key benefits of scenario-based solutions:
- Simulate various market scenarios to understand potential risks and returns
- Help financial institutions ensure compliance with regulatory requirements
- Identify potential outliers or anomalies in transactions
- Streamline reporting and reduce manual effort
By leveraging generative AI for scenario-based solutions, financial institutions can make more informed decisions, reduce risk, and stay ahead of the competition.
Synthetic for Simulations
Generative AI models, like Generative Adversarial Networks (GANs) or Variational Autoencoders (VAEs), can model complex financial relationships and generate synthetic data that mimics real market conditions. This synthetic data can be used to test and optimize trading algorithms, reducing the reliance on historical data alone.
Synthetic data for simulations is particularly valuable in the financial sector, where acquiring substantial amounts of data for AI or analytics purposes can be challenging without breaching privacy concerns.
On a similar theme: Generative Ai Synthetic Data
Generative AI can simulate different economic conditions and their impact on financial markets, helping policymakers and financial institutions plan for various future scenarios.
Financial institutions can use synthetic data to test new products and services in a safe and controlled environment, allowing for the assessment of potential issues and the refinement of products before they are introduced to the market.
Here are some key areas where synthetic data generation can be applied:
- Product testing and development: Financial institutions can use synthetic data to test new products and services.
- Compliance training: Synthetic data can be used to create realistic financial scenarios for compliance training purposes.
- Building in-house machine learning models: Financial services institutions can use synthetic data generated by Generative AI to build and train in-house machine learning (ML) models.
By leveraging synthetic data, financial institutions can develop and test models without risking customer privacy or breaching data regulations.
Training and Development
Synthetic data can be created to train better detection algorithms, helping to combat the scarcity of real fraudulent transactions.
Having a solid dataset is crucial for developing and implementing Generative AI models tailored to finance projects.
Limited data accessibility can hinder model development, but with the right algorithms and methodologies, Generative AI can help address this challenge.
By leveraging Generative AI, financial institutions can improve their detection algorithms and make more informed decisions.
A fresh viewpoint: Generative Ai Fraud Detection
For Training
For Training, it's essential to have a robust dataset to train your Generative AI models. Synthetic data can be created to help train better detection algorithms, especially for rare events like fraudulent transactions.
Real-world data can be limited, but Generative AI can help fill this gap. By generating synthetic examples of fraudulent activity, you can improve your detection algorithms' accuracy and efficiency.
AI models can generate synthetic borrower profiles to test the robustness of credit risk models. This helps improve the accuracy of credit scoring and default predictions.
Synthetic data can also be used to train models for other finance projects, such as detecting anomalies or predicting market trends. With a solid dataset, you can develop and implement Generative AI models tailored to your specific needs.
Expand your knowledge: Can Generative Ai Improve Social Science
Model Development and Implementation
To develop a Generative AI model tailored to finance projects, you'll need a solid dataset in hand. This dataset will serve as the foundation for your model's development and implementation.
With a strong dataset, you can deploy the right algorithms and methodologies to address the identified challenges and meet the defined objectives. This is a crucial stage in the development process.
Generative AI models require careful consideration of model generalizability and adaptability to ensure they can handle various financial tasks and scenarios. Limited data accessibility can be a significant challenge in this regard.
The development and implementation of Generative AI models involve meticulous project planning, in-depth research analysis, and cutting-edge technology solutions. This comprehensive approach enables businesses to leverage generative AI in business applications, streamlining complex processes and generating innovative content automatically.
You might like: Generative Ai in Software Development
User Experience and Support
Generative AI is revolutionizing the way financial institutions interact with their customers, providing personalized and efficient support through chatbots and virtual assistants. These AI-powered tools can analyze customer preferences and history to offer tailored assistance and advice in real-time.
With the ability to process customer queries instantly, GenAI-driven chatbots can significantly accelerate the speed of customer support, vastly improving upon traditional customer service channels. This is especially beneficial for financial institutions that need to handle a high volume of customer inquiries.
Financial institutions have a range of tools at their disposal to develop custom AI chatbots, including Chatbase, Botsonic, and CustomGPT.ai. These platforms offer advanced features for analyzing chatbot interactions, improving responses, and enhancing user experiences.
Here are some key benefits of using GenAI for customer support:
- Instant responses to customer queries
- Personalized assistance and advice in real-time
- Adaptive learning capabilities to enhance comprehension and problem-solving skills
Customer Support
Customer support is a crucial aspect of any business, and financial institutions are no exception. AI-powered chatbots and virtual assistants are revolutionizing the way businesses interact with their customers, providing personalized and efficient assistance.
Generative AI plays a pivotal role in transforming customer support services, delivering instant responses and vastly improving upon traditional customer service channels. These Gen AI-powered chatbots can analyze and understand individual customer preferences and history, tailoring their assistance and advice in real-time.
With the ability to analyze customer feedback from various sources, such as social media, surveys, and customer support interactions, financial institutions can gauge sentiment toward their products and services. This helps them tailor their offerings and marketing strategies to better meet customer needs and preferences.
See what others are reading: Generative Ai Assistance
Financial institutions have a variety of tools at their disposal to develop a custom AI chatbot, including Chatbase, Botsonic, and CustomGPT.ai. Each of these platforms offers advanced features for analyzing chatbot interactions, improving responses, and enhancing user experiences.
Here are some key benefits of using Gen AI-powered chatbots for customer support:
- Accelerate the speed at which customer queries are addressed
- Provide instant responses that improve upon traditional customer service channels
- Offer support that is tailored to each customer's unique financial situation
- Enhance comprehension and problem-solving skills within the finance function
Applicant-Friendly Loan Denials
A recent Forbes Advisor survey revealed that 42.4% of business owners used their loan funds for business expansion. This highlights the pivotal role loans play in shaping business trajectories. The banking sector's reliance on AI in loan decision-making becomes evident in this context.
AI aids in meticulously evaluating creditworthiness, setting apt credit limits, and tailoring loan pricing. Generative AI offers a deeper dive, identifying potential red flags by analyzing vast data points. This includes credit scores and subtle shifts in financial behaviors.
However, the crux lies in transparency. AI-driven loan decisions, especially denials, need to be clear and understandable. A conditional generative adversarial network (GAN) is a specialized form of Generative AI that crafts user-friendly explanations for loan denials.
This hierarchical approach ensures that feedback is insightful and easy to grasp. Two-level conditioning is employed to generate more understandable explanations for applicants. The goal is to foster trust and improve customer awareness for future applications.
Sources
- https://research.aimultiple.com/generative-ai-finance/
- https://appinventiv.com/blog/generative-ai-in-finance/
- https://www.appventurez.com/blog/use-cases-of-generative-ai-in-finance
- https://www.softkraft.co/generative-ai-in-finance-services/
- https://medium.com/@kanerika/top-10-use-cases-of-generative-ai-in-financial-services-and-banking-b560657cb0b1
Featured Images: pexels.com