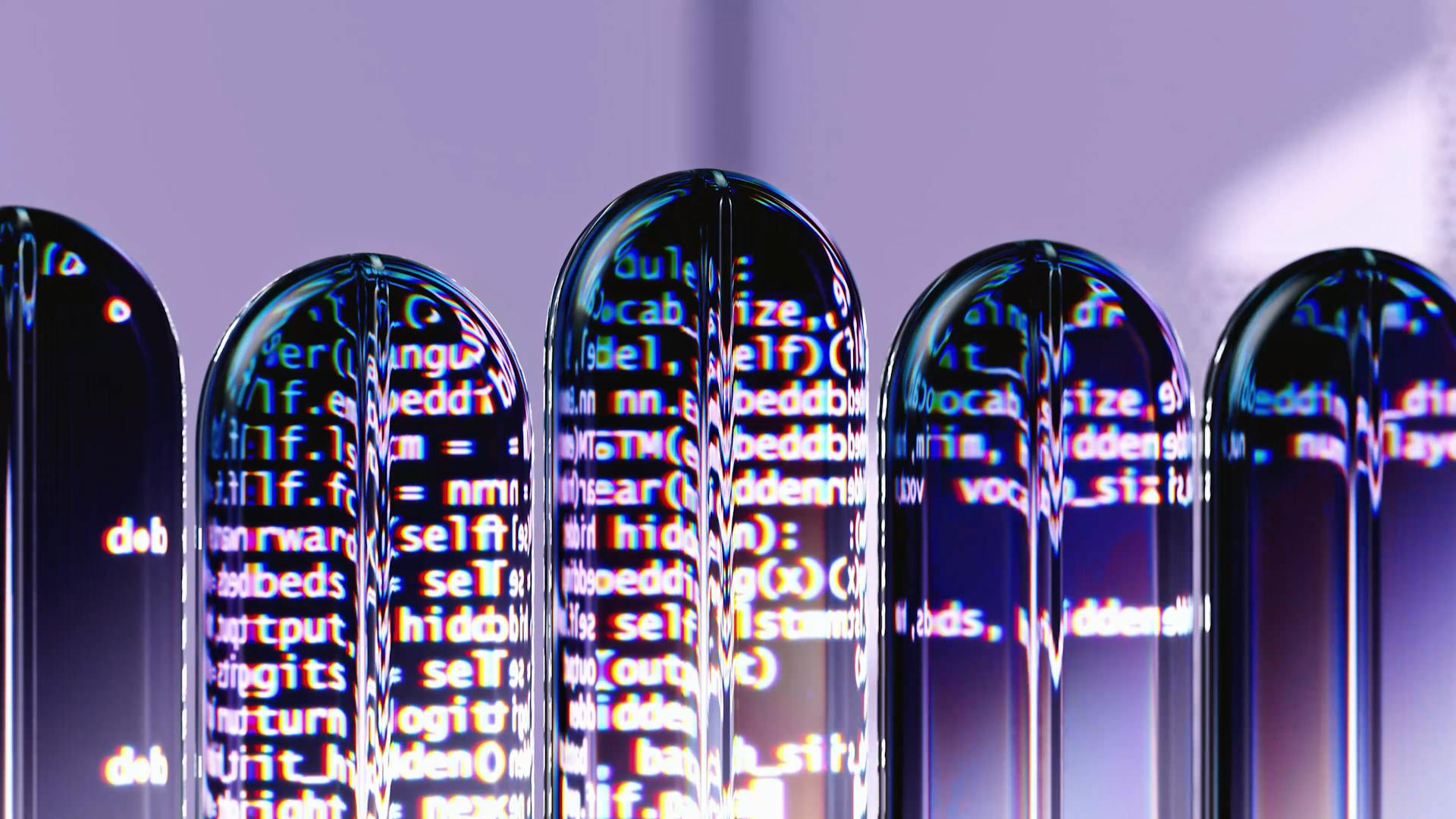
Generative AI has revolutionized the way we approach creative tasks, but it's essential to understand the pros and cons of this technology.
One significant advantage of generative AI is its ability to produce high-quality, unique content at an unprecedented scale and speed. This is because generative AI models can learn from vast amounts of data and generate new content based on patterns and relationships within that data.
However, the reliance on data quality and diversity can lead to biased or inaccurate results, as seen in the article section where a generative AI model generated a biased image. This highlights the need for careful data curation and model training to ensure the quality of the output.
The impact of generative AI on various industries is significant, with applications in art, music, and even healthcare, as mentioned in the article section. However, the potential for job displacement and the need for new skills in the workforce are also concerns that need to be addressed.
Check this out: New Generative Ai
Advantages and Applications
Generative AI fundamentally works by using existing content to generate new content, driven by unsupervised and semi-supervised machine learning algorithms. This technology has marked a new era in data generation and content creation that is set to disrupt several processes in different sectors, industries, and markets.
One of the main advantages of Generative AI is its ability to reduce dependence on human involvement in data and content creation. It can automate tasks such as creating lesson plans, developing differentiated learning tasks, and generating curriculum unit summaries, student assessment rubrics, and class discussion topics.
Teachers can use generative AI tools to quickly create lesson plans and develop customized learning materials to cater to students' individual learning styles. In fact, an Association of Heads of Independent Schools of Australia (AHISA) survey showed that the most commonly reported benefit of generative AI tools for teachers was time saved.
Generative AI can also be used in conjunction with a school management system to streamline tasks, such as creating and sharing resources more effectively, and personalizing student learning experiences. This can significantly reduce teacher planning workloads and increase the efficiency of marking and feedback.
You might like: What Are the Generative Ai Tools
Here are some examples of how generative AI can be used in different industries:
- Healthcare: Generative AI is being explored to help accelerate drug discovery, while tools such as AWS HealthScribe allow clinicians to transcribe patient consultations and upload important information into their electronic health record.
- Digital marketing: Advertisers, salespeople, and commerce teams can use generative AI to craft personalized campaigns and adapt content to consumers' preferences, especially when combined with customer relationship management data.
- Education: Some educational tools are beginning to incorporate generative AI to develop customized learning materials to cater to students' individual learning styles.
- Finance: Generative AI is one of the many tools within complex financial systems to analyze market patterns and anticipate stock market trends.
These are just a few examples of the many applications of generative AI. As the technology continues to evolve, we can expect to see even more innovative uses of this powerful tool.
Disadvantages and Issues
Generative AI models have the potential to make certain occupations obsolete or reduce the earning potential of affected professionals. Organizations tend to choose more cost-effective alternatives.
The technology has also raised concerns about misuse and abuse, such as academic papers being written using AI-based writing applications, and image generators being accused of copyright infringement and violations of personal data and privacy rights.
A major concern is the potential for spreading misinformation and harmful content, which can have wide-ranging and severe consequences, including perpetuating stereotypes, hate speech, and damaging personal and professional reputation.
Research suggests that around 12 million people may need to switch jobs by 2030, with office support, customer service, and food service roles most at risk.
A unique perspective: The Economic Potential of Generative Ai the Next Productivity Frontier
Cybersecurity Concerns
Security agencies have made moves to ensure AI systems are built with safety and security in mind. In November 2023, 16 agencies, including the U.K.’s National Cyber Security Centre and the U.S. Cybersecurity and Infrastructure Security Agency released the Guidelines for Secure AI System Development, which promote security as a fundamental aspect of AI development and deployment.
AI systems can be vulnerable to cybersecurity threats, and experts are working to address these concerns. A survey released in October 2024 found that AI, including generative AI, expertise has become the most in-demand skill amongst IT managers in the U.K.
Generative AI has also prompted workforce concerns, most notably that the automation of tasks could lead to job losses. Research from McKinsey suggests that around 12 million people may need to switch jobs by 2030, with office support, customer service, and food service roles most at risk.
If an organization implements Generative AI systems, IT and cybersecurity professionals should carefully delineate where the model can and cannot access data.
Explore further: How Are Modern Generative Ai Systems Improving User Interaction
Environmental Concerns
Generative AI can have a significant negative impact on the environment. This is largely due to the energy consumption of the data centers needed to run these systems, which can rapidly deplete water sources and increase emissions.
In 2019, Alphabet Inc. (GOOGL) set a goal to cut its total greenhouse gas emissions in half by 2030. However, its emissions actually grew 48% between 2019 and 2024.
The increase in emissions was primarily due to the growth in data center energy consumption and supply chain emissions associated with generative AI. This is a stark contrast to the company's promises of using AI to lower emissions.
Google's 2024 report noted that any mitigation or decrease in AI's climate effects may be challenging. This suggests that the company is facing significant difficulties in reducing the environmental impact of its AI systems.
Readers also liked: Impact of Generative Ai
Quality Control
Quality Control is a crucial aspect of Generative AI, as it's not perfect and can produce low-quality outputs.
Intriguing read: Generative Ai Photoshop Increase Quality
Generative AI models like ChatGPT have been known to provide responses to prompts about more recent events, which are often inaccurate.
The quality of outputs produced by a generative model is determined by the quality of its datasets or training sets.
A specific model can reflect the biases present in the training data, resulting in biased results if the training set is biased.
These biased results affect both the quality and reliability of outputs, making it essential to inspect and audit the data produced by Generative AI models.
For instance, Google Bard was criticized for an advertisement with a wrong claim that the James Webb Space Telescope was used to take the very first pictures of a planet outside the Solar System.
This highlights the importance of quality control in Generative AI, where outputs need to be inspected and audited to ensure accuracy and relevance.
Worth a look: How to Learn Generative Ai
Ethics and Regulations
Generative AI models are trained using large datasets scraped from the internet, making them vulnerable to intellectual property infringement.
Using a Generative AI service can expose an individual or organization to potential legal responsibilities, including copyright and trademark infringement.
Besides intellectual property infringement, a specific service may generate new data that could potentially violate privacy rights, such as generating personal or sensitive information.
Generative AI companies may clash with media companies over the use of published work, as these models are trained on internet-sourced information.
IT and cybersecurity professionals should carefully delineate where the model can and cannot access data to prevent potential issues.
The technology has also raised concerns about misuse and abuse, such as using chatbots to write academic papers.
Image generators have been accused of copyright infringement and violations of personal data and privacy rights, highlighting the need for responsible use of Generative AI.
If this caught your attention, see: Generative Ai in Customer Service
Technical Aspects
Generative AI uses a computing process called deep learning to analyze patterns in large sets of data and replicate those patterns to create new data that mimics human-generated data.
It employs neural networks, a type of machine learning process inspired by the human brain, to learn from the information it's fed. Neural networks don't require human supervision or intervention to distinguish differences or patterns in the training data.
Generative AI models can be run on various models, including generative adversarial networks, transformers, and variational autoencoders. These models use different mechanisms to train the AI and create outputs.
The more data a generative AI model is trained on and generates, the more convincing and human-like its outputs become. This is because the machine learning algorithms powering generative AI models learn from the information they're fed.
A fresh viewpoint: Generative Ai Human Creativity and Art Google Scholar
Networks
Generative AI models use a type of machine learning called deep learning to analyze patterns in large sets of data and replicate those patterns to create new data.
This process involves neural networks, a type of machine learning process loosely inspired by the way the human brain processes, interprets, and learns from information over time.
For your interest: Which Term Describes the Process of Using Generative Ai
Generative AI models can be run on various models, which use different mechanisms to train the AI and create outputs, including generative adversarial networks, transformers, and variational autoencoders.
These models get more sophisticated over time, the more data a model is trained on and generates, the more convincing and human-like its outputs become.
Generative adversarial networks comprise two neural networks known as a generator and a discriminator, which essentially work against each other to create authentic-looking data.
Transformer-based models are trained on large sets of data to understand the relationships between sequential information such as words and sentences, making them well suited for text-generation tasks.
Variational autoencoders leverage two networks to interpret and generate data, an encoder and a decoder, which can be used to increase the diversity and accuracy of facial recognition systems.
Google's Gemini is a text-to-text generative AI interface based on their large language model, similar to ChatGPT, and can answer questions or generate text based on user-given prompts.
DALL-E and Midjourney are examples of GAN-based generative AI models, which use generative adversarial networks to create authentic-looking data.
Expand your knowledge: Pre Trained Multi Task Generative Ai
Traditional vs. Discriminative vs Non-Discriminative
Traditional machine learning models, such as logistic regression, rely on a probability distribution to make predictions.
These models can be prone to overfitting, especially when dealing with complex datasets.
Discriminative models, like support vector machines, focus on finding the optimal hyperplane to separate classes.
They are often more accurate than traditional models but can be computationally expensive.
Non-discriminative models, including k-means clustering, group similar data points into clusters without considering class labels.
They are useful for exploratory data analysis and can help identify underlying patterns in the data.
Explore further: Difference between Generative Ai and Discriminative Ai
Interfaces
Generative AI interfaces have become increasingly accessible through user-friendly software interfaces.
These interfaces allow users to interact with generative AI using natural language, making it easier for non-technical users to adopt.
Voice-activated AI assistants, now ubiquitous in smartphones and smart speakers, illustrate the shift towards more intuitive interfaces.
Intuitive user gateways have significantly expanded the user base and potential applications of generative AI.
Modern generative AI interfaces have become a fundamental change driving the widespread adoption of generative AI.
You might enjoy: Which Is an Example Limitation of Generative Ai Interfaces
Multimodal Models
Multimodal models can understand and process multiple types of data simultaneously, such as text, images, and audio.
They allow AI models to create more sophisticated outputs, like generating an image based on a text prompt, as well as a text description of an image prompt.
DALL-E 3 and OpenAI's GPT-4 are examples of multimodal models that can do this.
These models can process multiple types of data at once, making them more powerful and versatile than single-modal models.
Multimodal models can be used for a wide range of applications, from generating artwork to creating more natural-sounding language.
Expand your knowledge: Generative Ai Prompt Examples
How to Make Work for School
Generative AI can be a game-changer for schools, but it requires a thoughtful and responsible approach.
Schools need an AI policy that covers responsible use, potential risks, and bias and errors. This policy should also outline the school's commitment to using AI tools fairly and safely.
Teachers should know what's expected of them when it comes to using AI in the classroom. They should be aware of how to effectively use AI to support learning.
A different take: Generative Ai Policy Template
You can treat ChatGPT like a calculator, allowing it for some assignments but not others. This helps students learn how to interact with AI and ask the right questions.
By allowing students to use generative AI for part of a task, you can teach them critical skills they'll need in a world full of AI programs. For example, students can use ChatGPT to create outlines for essays and then write them independently.
Specific Models and Tools
DALL-E is an example of a text-to-image generative AI that can generate photo-realistic imagery based on a user's input. It can also edit images by making changes within an image or extending an image beyond its original proportions or boundaries.
Some popular generative AI models and products include GPT-4, ChatGPT, DALL-E 3, Google Gemini, Claude 3.5, Midjourney, GitHub Copilot, Llama 3, and Grok. These models can perform various tasks, such as generating text, images, or code completions.
Readers also liked: Telltale Words Identify Generative Ai Text
Here's a brief overview of some of these models:
Google and Meta have also demonstrated photorealistic image generators, although these are not publicly available as of October 2024.
Examples of
ChatGPT is an example of text-to-text generative AI, trained to interact with users via natural language dialogue. It can compose text in different styles or genres, such as poems, essays, stories, or recipes.
OpenAI sells the application programming interface (API) for ChatGPT, among other enterprise subscription and embedding options. Many people use the free version of ChatGPT online.
Google's Gemini is a text-to-text generative AI interface based on Google's large language model, similar to ChatGPT. It can answer questions or generate text based on user-given prompts.
Gemini was launched in March 2023 in response to OpenAI's ChatGPT and Microsoft's Copilot AI tool. It was later launched in Europe and Brazil in 2023.
Google integrates generative AI into Search with AI Overviews. Microsoft incorporates the Copilot AI into PCs. Apple released Apple Intelligence, a mix of proprietary AI models and OpenAI technology, in iOS 18, iPadOS 18, and macOS Sequoia.
Discover more: Generative Ai with Large Language Models
Here are some examples of generative AI models and products:
DALL-E
DALL-E is a text-to-image generative AI released by OpenAI in January 2021. It uses a neural network trained on images with accompanying text descriptions.
Users can input descriptive text, and DALL-E will generate photo-realistic imagery based on the prompt. This can be a game-changer for creative professionals and hobbyists alike.
DALL-E can also edit images, whether by making changes within an image or extending an image beyond its original proportions or boundaries.
Take a look at this: Generative Ai Text Analysis
The History of
The concept of generative AI has been around since the 1950s, when Alan Turing's research on machine thinking laid the foundation for modern AI.
In 1957, Frank Rosenblatt invented the first neural networks that could be trained, a crucial technology underlying generative AI.
The journey to the AI powerhouses we see today was marked by waves of innovation and periods of stagnation, with neural networks gaining traction in the 1980s.
For another approach, see: Neural Network vs Generative Ai
The introduction of generative adversarial networks (GANs) in 2014 by Ian Goodfellow and his colleagues revolutionized the field, opening new frontiers in generating images, music, and text.
The 2010s saw an explosion in deep learning capabilities, fueled by advances in computing power and the availability of massive data sets.
The release of GPT-3 in the 2020s showcased AI's potential to produce coherent, contextually relevant content across various domains.
The true economic impact of generative AI began to crystallize in 2022 with the public release of ChatGPT, which reached an estimated 100 million users within just two months of launch.
For your interest: Generative Ai Release Date
Challenges and Limitations
Generative AI is not a perfect solution. It can be prone to errors and inaccuracies, especially when dealing with complex or nuanced topics.
One of the main limitations of generative AI is its lack of human judgment and expertise. As we saw in the article, a study found that AI-generated text can be 10-20% less accurate than human-written text.
This can be particularly problematic in high-stakes situations, such as medical diagnosis or financial analysis. In these cases, the consequences of AI-generated errors can be severe.
Take a look at this: Is Speech to Text Generative Ai
Environmental Impact
Google's own data shows that its emissions grew 48% since 2019, with a 13% increase in 2023 alone. This is largely due to increased energy consumption and supply chain emissions from generative AI.
Generative AI can increase emissions and rapidly deplete water sources. The data centers needed to run generative AI have become a key conversation in the debates over the Earth's future energy needs.
Google's 2024 report noted that any mitigation or decrease in AI's climate effects "may be challenging." This is a stark contrast to its earlier promises of AI's potential to lower emissions.
The increase in emissions from Google's data centers is a pressing concern, and it's essential to address this issue to meet the company's 2030 timeline.
Readers also liked: What Is Google's Generative Ai Called
Challenges in Schools
The rise of generative AI in schools is a double-edged sword. Calculators reduced the need for basic arithmetic skills, but generative AI poses a bigger threat to creativity and critical thinking.
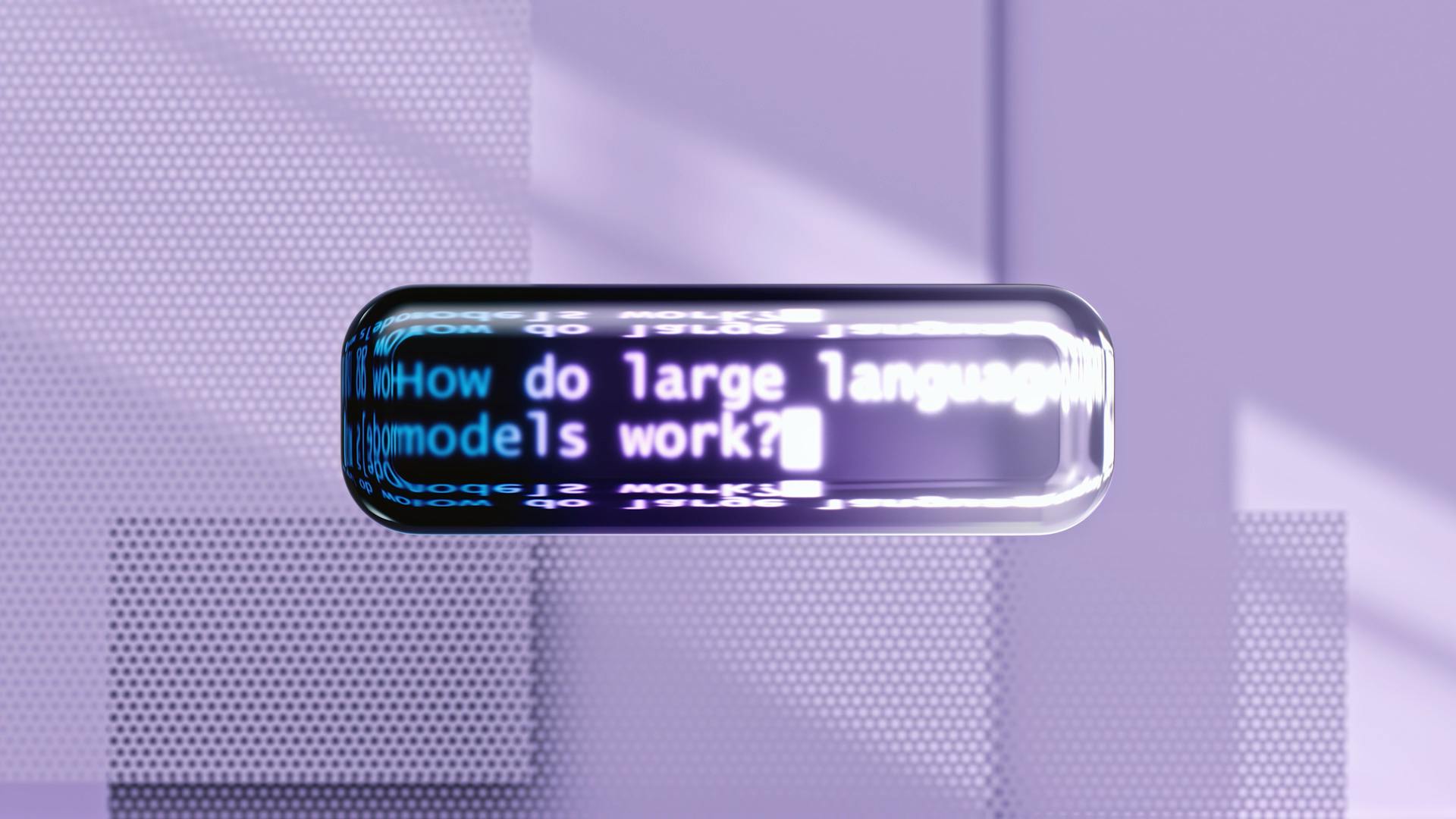
Some educators worry that AI will lead to a permanent downgrading of human skills and knowledge. There's a risk that students may become dependent on AI technology to do the work for them.
Why would a student bother learning when a robot can give the answers? This complacency could be a major issue in schools.
School leaders are concerned about inherent bias in generative AI tools. This could lead to unfair outcomes for certain students.
Teachers have to figure out how to detect and manage output from generative AI tools. This is a challenge they're facing head-on.
Explore further: Top Generative Ai Tools
Key Takeaways
Generative AI is a form of machine learning that can produce text, video, images, and other types of content. This technology is incredibly versatile and has a wide range of applications.
ChatGPT, DALL-E, and Gemini are just a few examples of generative AI applications that can produce text or images based on user-given prompts. These tools are being used in various industries, from automotive to media/entertainment.
For another approach, see: Generative Ai by Getty Images
Generative AI is being used in everything from creative to academic writing and translation, to composing, dubbing, and sound editing. It's also being used in infographics, image editing, and architectural rendering.
The potential applications of generative AI are vast, but so are the concerns surrounding its use. Some of the concerns include its potential legal, ethical, political, ecological, social, and economic effects.
Consider reading: How Can Generative Ai Be Used in Cybersecurity
Sources
- https://www.profolus.com/topics/advantages-and-disadvantages-of-generative-ai/
- https://www.investopedia.com/generative-ai-7497939
- https://www.techrepublic.com/article/what-is-generative-ai/
- https://educationhorizons.com/blog/generative-ai-the-pros-and-cons-for-students-and-schools/
- https://www.procon.org/headlines/artificial-intelligence-ai-top-3-pros-and-cons/
Featured Images: pexels.com