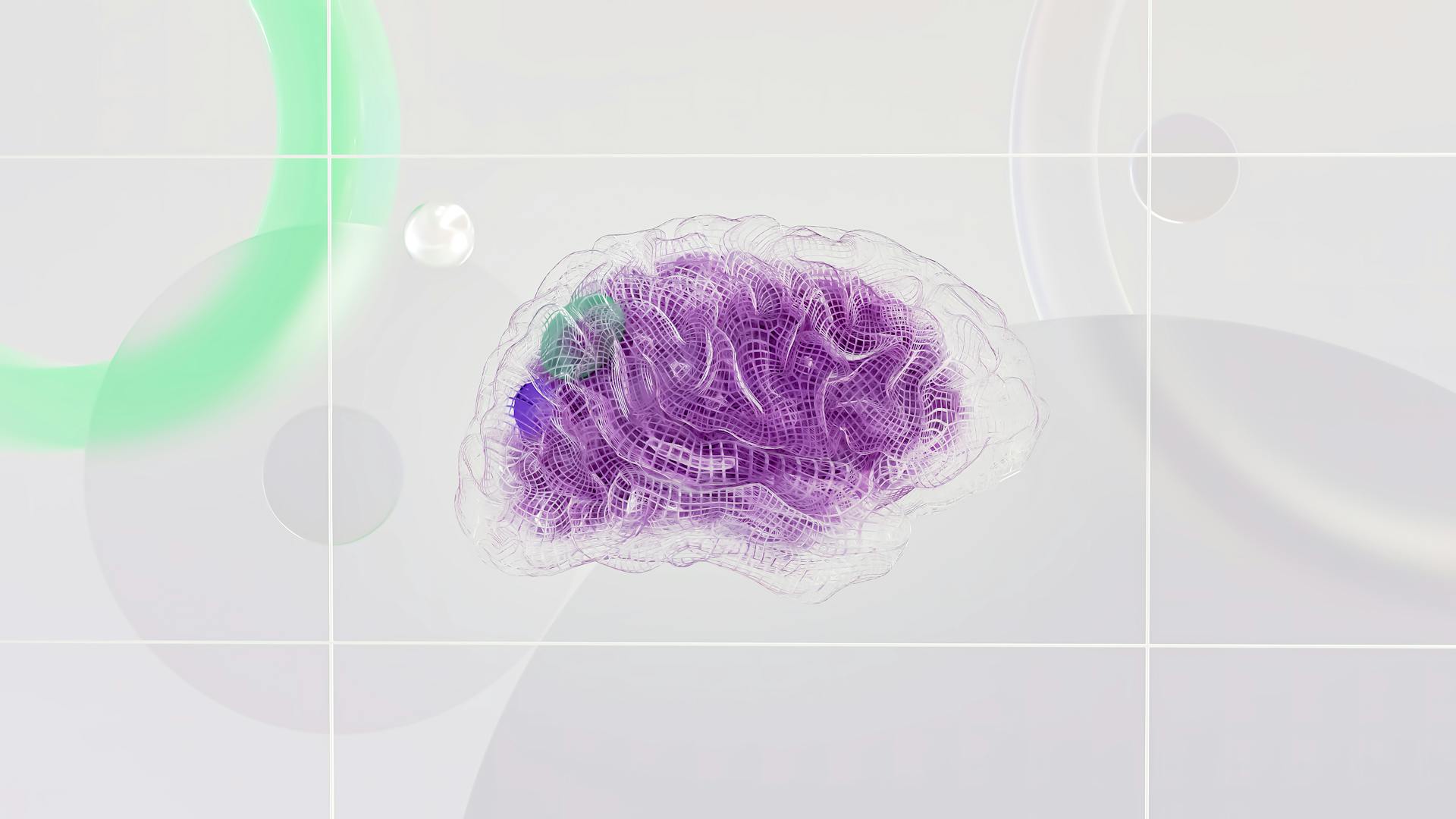
Machine learning is revolutionizing the financial industry, and Fin Tech companies are at the forefront of this transformation. Many Fin Tech companies are using machine learning to improve customer experience and reduce costs.
One of the top machine learning applications in Fin Tech is risk management. This is done by analyzing large amounts of data to identify potential risks and prevent financial losses.
Machine learning algorithms can quickly process vast amounts of financial data, enabling Fin Tech companies to make more accurate predictions and decisions. This has led to increased efficiency and productivity in the industry.
Real-time credit scoring is another significant application of machine learning in Fin Tech. This allows lenders to make instant decisions on loan applications, reducing the time and effort required for traditional credit checks.
Explore further: Data Labeling Companies
Machine Learning in Finance
Machine learning has been delivering value to fintech for over a decade. It's been used in various verticals around the world to create successful projects.
Fraud detection and prevention is one area where machine learning shines. By analyzing vast amounts of financial data, AI can instantly recognize basic spending patterns and flag inconsistencies for review. This is especially useful in combating global crimes in a 24/7 digital environment.
Machine learning-enhanced credit scoring pairs up with big data to create an accurate picture of a borrower's risk profile. This approach reduces bias and opens up financial opportunities to a broader demographic range.
Here are some benefits of machine learning in credit scoring:
- Accurate credit risk assessments by analyzing a wide range of data, from traditional credit scores to alternative sources.
- Faster loan approvals through automated credit scoring models.
- Greater reach to unbanked prospects by enabling the evaluation of creditworthiness for individuals without a traditional credit history.
- Reduced lending bias by relying on data-driven objective parameters.
Financial Forecasting
Machine learning in finance is revolutionizing the way we approach financial forecasting. AI reduces the overwhelm involved with cash-flow forecasting, allowing financial professionals to focus on the right trends at the right time.
AI flags bad data that can derail predictive accuracy, ensuring that financial forecasts are as accurate as possible. It also helps financial professionals make better business decisions by providing real-time access to up-to-date financial data.
AI forecasts use a combination of the company's own user data and data from third-party sources, such as weather, commodity values, foreign political forces, and historical customer trends. This helps inform better financial predictions and reduce risk over time.
Here are some key benefits of AI-powered financial forecasting:
- Improved accuracy: AI reduces the risk of human error and ensures that financial forecasts are as accurate as possible.
- Increased speed: AI can rapidly analyze large volumes of data, allowing financial professionals to make faster business decisions.
- Enhanced data accessibility: AI provides real-time access to up-to-date financial data, enabling financial professionals to make more informed decisions.
Credit Scoring
Credit scoring has come a long way with the help of machine learning. Accurate credit risk assessments are now possible by analyzing a wide range of data, including traditional credit scores and alternative sources like social media activity and payment history.
This approach has led to reduced default rates and more informed lending decisions. Faster loan approvals are also a result of automated credit scoring models, making it possible to provide borrowers with quick decisions, which is crucial for personal loans and small business financing.
Machine learning helps extend financial services to underserved populations by enabling the evaluation of creditworthiness for individuals without a traditional credit history. This is a key business concern in developing countries.
Here's an interesting read: Machine Learning Applications in Business
Here are some benefits of machine learning in credit scoring:
- Accurate credit risk assessments
- Faster loan approvals
- Greater reach to unbanked prospects
- Reduced lending bias
New AI-based credit scoring models can predict with better accuracy if someone is truly a credit risk by looking at historical information from a variety of public and private sources. This opens the door for more borrowers who have been traditionally ignored.
You might like: Credit Assignment Problem
Algorithmic Trading
Algorithmic trading is a game-changer in the world of finance, and it's all thanks to the perfect match between machine learning and fintech. Machine learning algorithms can process vast amounts of market data at incredible speeds, enabling traders to make quicker decisions with high accuracy.
This is particularly useful because it allows traders to analyze market data beyond what's possible for humans. In fact, machine learning algorithms can process data in a fraction of the time it would take a human trader, making it a valuable support tool for traders who want to make informed decisions.
A unique perspective: Automated Decision-making
One of the key benefits of algorithmic trading is its higher predictive power. Well-trained machine learning models can greatly enhance the accuracy of trading predictions, improving the likelihood of profitable transactions. This is a huge advantage for traders who want to stay ahead of the curve.
Algorithmic trading systems also have the ability to adapt to changing market conditions. By learning from past transactions, these systems can continuously improve their performance and make more accurate predictions. This is a key advantage over traditional trading methods, which can be slower and less responsive to market changes.
Here are some key benefits of algorithmic trading:
- Analysis beyond human possibility
- Support tool for traders
- Higher predictive power
- Adaptation to market conditions
Customer Service and Support
Customer service is a crucial aspect of any fintech company, and machine learning is revolutionizing the way customer support is handled. With 60% of customers choosing chatbots over human customer agents, it's clear that time is of the essence.
Round-the-clock support is now a reality, thanks to chatbots powered by machine learning. These chatbots can handle a high volume of inquiries, ensuring that no request goes unanswered.
Predictive AI is also being used to identify potential customer churn, allowing fintech companies to proactively target customers who are at risk of leaving. By analyzing events like logins and search behavior, predictive AI can score how likely a customer is to churn.
Customer interactions are being efficiently managed by ML chatbots, which collect and cross-analyze different data points on customer interactions. This provides valuable insights for improving products and services.
Here are some key benefits of ML-powered customer service chatbots:
- Round-the-clock support
- Customer interactions management
- Improved response times
- Data collection on customer interactions
Robo-Advisors and Personalized Advice
Robo-advisors and personalized advice have revolutionized the way we approach financial planning. By leveraging machine learning, robo-advisors can build and manage investment portfolios tailored to individual risk tolerance and financial goals.
Users can enter their present financial assets and expected income, set their goals, and have their robo-advisors allocate the assets across investment opportunities according to the objectives defined. This level of personalization is a game-changer for investors who want to make informed decisions without breaking the bank.
Robo-advisors use machine learning to recommend financial products and services based on customer profiles and preferences. This means users can receive personalized suggestions about savings, investments, and insurance solutions while eschewing the higher fees associated with personal financial advisors.
Some key benefits of robo-advisors include:
- Portfolio management: Robo-advisors use machine learning to build and manage investment portfolios tailored to individual risk tolerance and financial goals.
- Financial recommendations: Machine learning-driven robo-advisors can recommend financial products and services based on customer profiles and preferences.
By 2024, global investors will put $1.8 trillion into robo-advisors, a testament to the trust and confidence they have in this technology.
Compliance and Reporting
Machine learning is a game-changer for financial institutions when it comes to compliance and reporting. It can automate regulatory reporting and monitor compliance violations with unparalleled speeds, ensuring that financial institutions always meet regulatory demands.
Machine learning can process regulatory reports at incredible speeds, allowing for the automation of regulatory reports generation and reducing the time and effort required to ensure fintech regulatory compliance with financial regulations.
With machine learning, financial institutions can significantly reduce the compliance cost of financial institutions. This is because machine learning can correlate documents and guidelines, and when paired with continuous monitoring of financial operations, ML systems can automatically track and identify potential compliance violations as they occur.
Here are some key benefits of machine learning in compliance and reporting:
- Automated regulatory reports generation
- Reduced time and effort required for regulatory compliance
- Automatic tracking and identification of potential compliance violations
Task Automation and Efficiency
Machine learning has revolutionized the fintech industry by automating repetitive tasks, freeing up time and resources for more strategic work.
Automating tasks such as customer onboarding and financial transaction reconciliation saves both time and resources, reducing the risk of human error.
In fact, 58 percent of finance professionals feel overworked, and ML-driven automation can help alleviate burnout by eliminating busy work.
Here are some benefits of automation in fintech:
- Streamlines customer onboarding processes
- Eliminates manual data entry for financial transactions
- Reduces the risk of human error
AI-powered efficiency in regulatory compliance is another significant advantage of machine learning in fintech. AI provides cost-effective solutions for maintaining up-to-date knowledge of evolving regulations.
Predictive analytics help identify potential non-compliance issues before they escalate into significant problems, ensuring that fintech businesses stay ahead of the curve.
Machines don't get tired, thus ensuring 24/7 monitoring and analysis, which is impossible with purely human teams.
Data Processing
Machine learning can handle large-scale data processing and analysis, allowing fintech businesses to extract valuable data-driven insights.
Fintech companies can use technologies like optical character recognition (OCR) and automated document processing systems to streamline processes and reduce costs.
By automating tasks such as loan application processing and Know Your Customer (KYC) checks, businesses can minimize the need for extensive data analysis teams.
This can also help with regulatory compliance, making it easier for fintech companies to stay on top of changing regulations and avoid costly fines.
Machine learning can also help fintech businesses predict loan defaults, allowing them to allocate resources more efficiently and mitigate potential losses.
AI and Machine Learning Applications
Machine learning is solving fundamental issues in the fintech industry, such as fraud and regulatory compliance. It's not just about insurance or crypto, but a broader problem that affects the entire industry.
Automating the underwriting process with AI can streamline operations, improve accuracy, and save money by reducing errors caused by manual interventions. This is achieved through sophisticated machine learning algorithms.
AI in fintech is all about increasing process efficiency and profitability. By condensing the core benefits of AI in fintech into a few objective bullet points, we can see how it helps businesses.
Here are the core benefits of AI in fintech:
- More personalization through data-driven insights
- Improved efficiency by doing more faster
- Enhanced accuracy through fewer errors
- Better security by detecting anomalies and bad data
- Quicker time to market by predicting market performance
These benefits are not just limited to businesses, but also extend to consumers. Safety tops the list of consumer advantages, as AI significantly reduces the chances of falling victim to fraudulent activities.
Companies and Projects
Machine learning has been delivering value to fintech for over a decade.
Some notable examples include successful fintech machine learning projects from around the world, such as those in multiple verticals.
These projects have been delivering value in various ways, including improving customer experience and reducing costs.
For instance, machine learning has been used to automate tasks and enhance customer service in the fintech industry.
Companies in Finance
Companies in Finance are often at the forefront of innovation, with many investing heavily in emerging technologies like blockchain and artificial intelligence.
Goldman Sachs is a prime example, with their acquisition of a blockchain-based startup in 2016.
The financial sector is also home to many large corporations, including JPMorgan Chase, which has a market capitalization of over $400 billion.
In recent years, there has been a trend towards fintech companies, such as Square, which offers a range of financial services to small businesses.
These companies are often disrupting traditional banking practices and offering more user-friendly and accessible services.
Here's an interesting read: Quantum Machine Learning Companies
Projects to Learn From
Machine learning has been delivering value to fintech for over a decade.
One great case is from the US, where a fintech company used machine learning to reduce loan defaults by 25% through more accurate risk assessments.
Fintech companies are leveraging machine learning to improve customer experiences and drive business growth.
In Australia, a fintech startup applied machine learning to automate credit decisions, resulting in a 30% increase in loan approvals.
Machine learning is being used to analyze customer behavior and preferences, enabling fintech companies to offer personalized financial services.
A fintech company in the UK used machine learning to detect and prevent financial fraud, resulting in a 40% reduction in fraudulent transactions.
Use Cases and Applications
Machine learning in fintech is a game-changer, solving issues that affect the entire industry. From fraud detection to regulatory compliance, machine learning is a versatile solution that can be applied across various market segments and business models.
Insurtech, tradetech, and regtech are just a few examples of areas where machine learning shines. It's not limited to specific regions or problems, making it a valuable tool for fintech companies.
Machine learning is improving internal processes, making them more efficient and effective. By automating tasks and identifying areas for improvement, fintech companies can reduce costs and increase productivity.
Fraud detection is a major concern for fintech companies, and machine learning is helping to solve it. By analyzing patterns and behavior, machine learning algorithms can identify and prevent fraudulent activity.
Robust regulatory compliance is also a challenge for fintech companies, but machine learning is helping to address it. By analyzing large datasets and identifying potential risks, machine learning algorithms can help fintech companies stay compliant with regulations.
Here's an interesting read: Supervised Machine Learning Algorithms
Sources
- https://ventionteams.com/blog/machine-learning-fintech
- https://builtin.com/artificial-intelligence/machine-learning-finance-examples
- https://www.pecan.ai/blog/ai-in-fintech-top-7-use-cases/
- https://www.techaheadcorp.com/blog/top-25-fintech-ai-use-cases/
- https://www.linkedin.com/pulse/10-insights-how-fintech-companies-use-machine-learning-soyinka
Featured Images: pexels.com