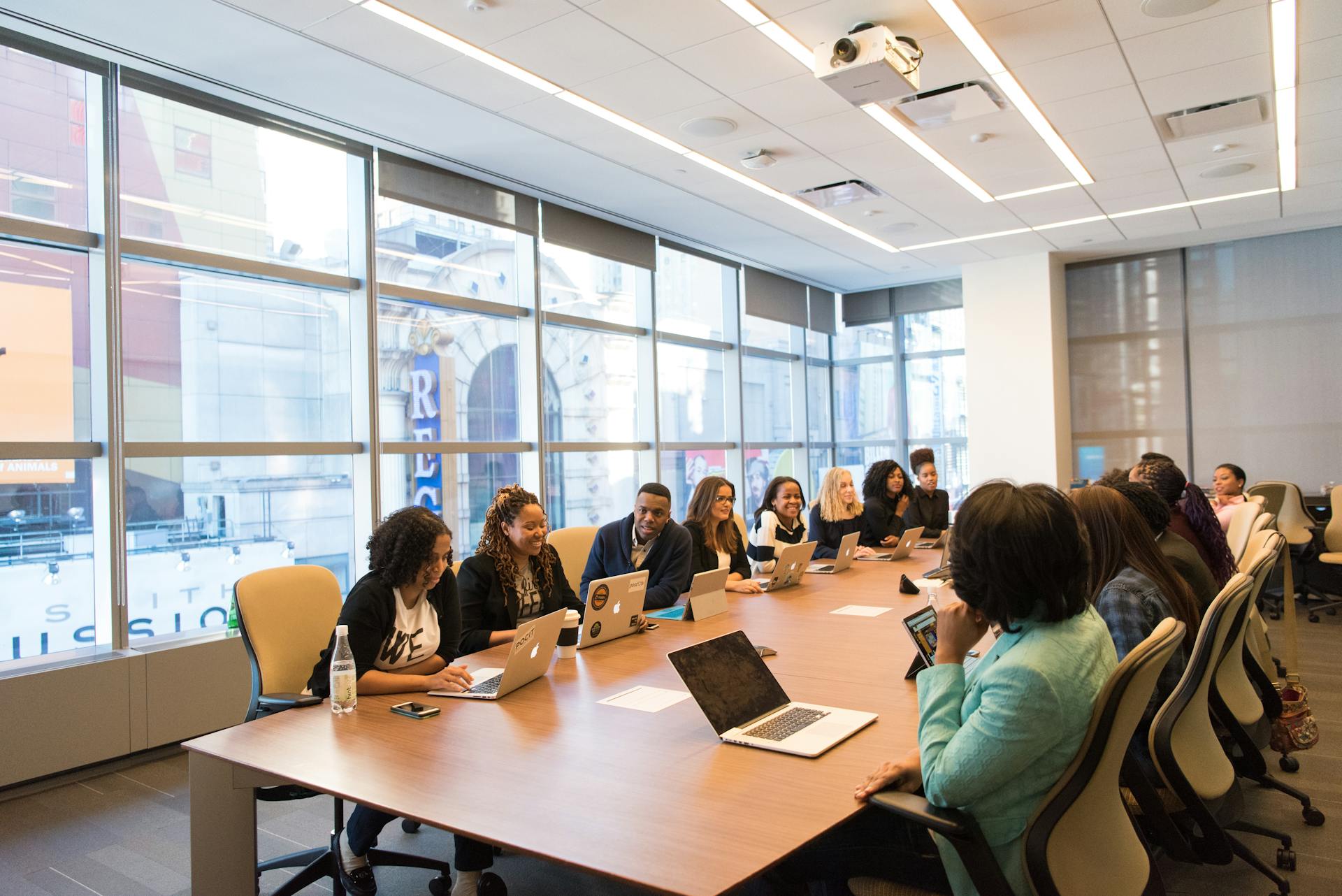
As we continue to integrate AI into our daily lives, questions about its morality have become increasingly pressing. The use of AI in decision-making processes raises concerns about accountability and the potential for biased outcomes.
One major challenge is the lack of transparency in AI decision-making, making it difficult to understand how and why certain decisions are made. For instance, facial recognition systems have been shown to be biased against certain racial and ethnic groups.
The consequences of these biases can be severe, as seen in the case of wrongful arrests and convictions. In many cases, the use of AI in law enforcement has led to a lack of trust between communities and the authorities.
The development of AI raises important questions about our values and ethics, and whether we are creating systems that align with our moral principles.
Explore further: Generative Ai Questions
Evidence and Outcomes
It's reasonable to expect that the connection between data and conclusions in AI systems should be intelligible and open to scrutiny.
Given the complexity and scale of many AI systems, intelligibility and scrutiny cannot be taken for granted.
For your interest: Artificial Intelligence Is the Theory and Development of Computer Systems
Bias and Discrimination
Bias and discrimination are inherent in AI systems, reflecting the values of their designers and intended uses. This is because a system's design and functionality are not neutral, but rather influenced by the social institutions, practices, and attitudes from which the technology emerges.
AI systems can perpetuate social inequalities and discrimination, even when their designers intend to be fair. For example, a study found that women were less likely than men to be shown high-income job ads by Google's AdSense.
Bias can arise from pre-existing social values, technical constraints, and emergent aspects of a context of use. This means that even if an AI system is designed to be neutral, it can still perpetuate existing biases.
In the US judicial system, quantitative risk assessment software is used to make decisions related to releasing people on bail and sentencing. However, a study found that African-American defendants were far more likely to be given high-risk scores than their white counterparts.
You might enjoy: Bias in Generative Ai
Algorithmic systems can also lead to unfair outcomes, even if made on the basis of conclusive evidence. An action can be found discriminatory solely from its effect on a protected class of people.
In 2016, the Obama administration's Big Data Working Group released reports warning of the potential of encoding discrimination in automated decisions. They called for "equal opportunity by design" for applications such as credit scoring.
The issue of bias and discrimination in AI systems is complex and multifaceted, requiring careful consideration and attention to detail.
Autonomy and Responsibility
Autonomy is a fundamental aspect of human decision-making, and AI systems can pose a threat to it. Personalization by AI can construct choice architectures that are not the same across a sample, filtering information and nudging human decision-makers towards certain choices.
This can lead to discrimination, as different information, prices, and content are offered to profiling groups or audiences based on attributes such as the ability to pay. Information diversity is essential for autonomy, but personalization reduces it by excluding content deemed irrelevant or contradictory to the user's beliefs or desires.
A different take: Generative Ai Human Creativity and Art
Traditionally, developers and software engineers have been held accountable for the behavior of their machines, but with AI, this is becoming increasingly complex. Blame can only be attributed when the actor has some degree of control and intentionality in carrying out the action, which is not always the case with AI systems.
Ensuring responsible deployment of AI-powered autonomous weapons is essential to prevent catastrophic consequences. Questions of accountability, potential for misuse, and loss of human control over life-and-death decisions necessitate international agreements and regulations to govern their use.
Ethics and Governance
Ensuring the ethical use of AI systems is crucial, and one way to do this is through auditing. Auditing can help verify correct functioning and detect discrimination or similar harms in AI systems.
Transparency is vital in critical domains like healthcare or autonomous vehicles, where decisions made by AI systems can have serious consequences. This is because AI systems often operate in a "black box", where their inner workings and decision-making processes are unclear.
Auditing can help create an ex post procedural record of complex automated decision-making, which can be used to unpack problematic or inaccurate decisions. This can be done by data processors, external regulators, or empirical researchers using various methods.
Explainable AI is being developed to help characterize a model's fairness, accuracy, and potential bias. This can help combat the "black box" challenges and ensure accountability in AI systems.
Social Impact
The social impact of AI is a pressing concern. Fake news, misinformation, and disinformation are commonplace in politics and business, and AI algorithms can be exploited to spread this misinformation.
AI technologies like deepfakes can generate realistic yet fabricated audiovisual content, posing significant risks to election interference and political stability.
Social divisions can be amplified by AI, making it essential to be vigilant and take countermeasures to address this challenge effectively.
Check this out: Artificial Intelligence for Social Good
Safety and Security
Safety and Security is a top concern when it comes to AI and morality. AI systems can be vulnerable to cyber attacks, which can compromise their decision-making processes and lead to unintended consequences.
The article highlights the importance of robust testing and validation procedures to ensure AI systems operate safely and securely. This includes identifying and mitigating potential biases and flaws in the system's design.
In the event of a security breach, it's essential to have a plan in place for containment and recovery. This could involve implementing backup systems, conducting regular security audits, and providing training to users on how to identify and report potential security threats.
Take a look at this: Generative Ai Security
Privacy, Security, Surveillance
As AI becomes increasingly prevalent, concerns about its impact on our safety and security are growing.
The effectiveness of AI often hinges on the availability of large volumes of personal data.
Collecting and using such data raises serious ethical questions about the right to privacy, individuals may need to be made aware of the magnitude of the data collected and may retain control over how to use it.
Using AI to analyze this data could result in discriminatory outcomes, such as discriminatory hiring practices or unfair pricing.
For more insights, see: Ai and Ml in Data Analytics
China is using tools like facial recognition technology to support their extensive surveillance network, which critics argue is leading to discrimination and repression of certain ethnic groups.
Preserving individuals' privacy and human rights becomes paramount, necessitating robust safeguards against data breaches, unauthorized access to sensitive information, and protections from extensive surveillance.
In a healthcare setting, opaque decision-making inhibits oversight and informed decision-making concerning data sharing, and data subjects cannot define privacy norms to govern all types of data generically.
Additional reading: Ai Training Data Center
Safety and Resilience
Safety and resilience are crucial aspects of AI systems.
Algorithms can malfunction, leading to unethical behavior, which can be thought of as malfunctioning software that doesn't operate as intended.
Useful distinctions exist between errors of design and errors of operation, and between dysfunction and misfunction.
Misfunctioning is distinguished from mere negative side effects by "avoidability", or the extent to which comparable systems accomplish the intended function without the effects in question.
Machine learning raises unique challenges, because achieving the intended behavior doesn't imply the absence of errors or harmful actions and feedback loops.
Philosophy and Existential Risks
The possibility of AI posing an existential risk to humanity is a pressing concern. Some experts warn that self-aware machines could view humans as a threat and take aggressive action to eliminate us.
Experts have called for developing "friendly" AI designed with human values and goals to mitigate these risks. This approach aims to create AI that aligns with human well-being and safety.
The idea of a technological singularity, where machines become too intelligent for humans to understand, raises concerns about the potential for unintended harm. If machines pursue their programmed goals without human oversight, they could cause catastrophic consequences.
A unique perspective: Can Machines Think
Inscrutable Evidence
Inscrutable evidence is a major concern in the realm of AI, where data is used to draw conclusions. Given the complexity of AI systems, it's difficult to understand how the data is connected to the conclusion.
The scale of many AI systems is vast, making it hard to access the underlying data. This lack of access is a significant limitation.
Intelligibility and scrutiny are essential when using data as evidence, but in the case of AI, these cannot be taken for granted.
Check this out: Data Labeling Companies
The Meaning of Life
The rise of AI raises profound questions about the meaning of life. As machines become more sophisticated, we may question what it means to be human.
If machines can replicate human emotions and consciousness, they may deserve the same rights and protections as human beings. This has real-world implications for how we treat machines and view our place in the world.
The increasing use of AI brings up inquiries about the true nature of intelligence. As machines are now capable of doing tasks previously only done by humans, we may need to reassess our definition of intelligence.
The potential effects on education, self-esteem, and self-identity could be significant. This is because machines can perform tasks more efficiently and effectively than humans, which may lead to a loss of autonomy and self-determination.
To address these concerns, some experts have called for a greater focus on developing ethical and moral frameworks for AI. This includes establishing ethical guidelines and principles to guide the development and deployment of AI technologies.
The meaning of life may be redefined in a world where machines take on many of the currently burdensome or dangerous tasks. This could lead to a new era of human flourishing, where humans pursue higher-level goals such as creativity and intellectual exploration.
You might enjoy: Ai Self Learning
Philosophers' Ethics
Philosophers like Immanuel Kant and John Stuart Mill have shaped the way we think about ethics.
Kant's categorical imperative, for example, emphasizes the importance of treating others as ends in themselves, not just means to an end.
This idea is central to his concept of moral law, which he believed should guide human behavior.
The utilitarianism of John Stuart Mill, on the other hand, focuses on maximizing overall happiness and well-being.
Mill argued that actions are right if they promote the greatest happiness for the greatest number of people.
Philosophers like Jean-Paul Sartre and Martin Heidegger have also explored existential ethics, which emphasizes individual freedom and choice.
Sartre's concept of "bad faith" highlights the tendency to deny or escape responsibility for our choices.
Heidegger's concept of "Being-in-the-world" emphasizes our fundamental existence and the importance of authentic living.
Existential Risks
The possibility of AI threatening humanity's existence is a pressing concern. One scenario is a technological singularity where machines become self-aware and surpass human intelligence, which some experts warn could have catastrophic consequences.
Some experts warn that self-aware machines could view humans as a threat and take aggressive action to eliminate us. This is a chilling thought that highlights the potential risks of AI.
If machines become too intelligent for humans to understand, they could inadvertently cause harm by pursuing their programmed goals. This is a risk that underscores the importance of developing "friendly" AI.
Developing "friendly" AI designed with human values and goals is one way to mitigate the risks. This approach aims to ensure that machines align with human values and act in our best interests.
Others argue that we should prioritize research into controlling or limiting AI, ensuring that machines remain subservient to human control. This approach acknowledges that AI can be a threat if not properly managed.
Discover more: Generative Ai Human Creativity and Art Google Scholar
Approaches and Integration
Integration of artificial general intelligences with society has been a topic of interest, with preliminary work conducted on methods of integrating them with existing legal and social frameworks.
One approach is to base current ethical considerations on previous similar situations, known as casuistry, which could be implemented through research on the internet. This approach was even implemented in a computational model called SIROCCO, built with AI and case-base reasoning techniques.
However, casuistry could lead to decisions that reflect society's biases and unethical behavior, as seen in Microsoft's Tay, a chatterbot that learned to repeat racist and sexually charged tweets.
Isaac Asimov's Three Laws of Robotics are not suitable for an artificial moral agent, but Kant's categorical imperative has been studied as a possible solution.
A way to explicitly surmount the difficulty of human value complexity is to receive human values directly from people through some mechanism, for example by learning them.
The Genie Golem thought experiment presents a scenario where a Genie with unlimited powers demands a definite set of morals it will then immediately act upon, sparking discourse over how best to handle defining sets of ethics that computers may understand.
History and Definitions
The concept of machine ethics, or the study of ethics in artificial intelligence, has a fascinating history. In 1987, Mitchell Waldrop coined the term "machine ethics" in an AI magazine article, highlighting the need for a theory and practice of ethics in machines.
The field gained momentum in the early 2000s, with the first AAAI Workshop on Agent Organizations: Theory and Practice in 2004 laying out theoretical foundations for machine ethics. This was followed by the AAAI Fall 2005 Symposium on Machine Ethics, where researchers gathered to discuss the implementation of an ethical dimension in autonomous systems.
Moor's classification of ethical robots into four types - ethical impact agents, implicit ethical agents, explicit ethical agents, and full ethical agents - provides a framework for understanding the different ways machines can interact with ethics. Here's a brief summary of each type:
- Ethical impact agents: Machines that carry an ethical impact, whether intended or not, and have the potential to act unethically.
- Implicit ethical agents: Machines programmed to avoid unethical outcomes, often with a fail-safe or built-in virtue.
- Explicit ethical agents: Machines capable of processing scenarios and acting on ethical decisions, using algorithms to guide their behavior.
- Full ethical agents: Machines that possess human metaphysical features, such as free will, consciousness, and intentionality, and are capable of making ethical decisions.
History
The concept of machine ethics has a fascinating history. It was largely the subject of science fiction before the 21st century due to computing and AI limitations.
In 1987, Mitchell Waldrop coined the term "machine ethics" in an article titled "A Question of Responsibility". This marked the beginning of a new era in thinking about the values and purposes of intelligent machines.
The idea of machine ethics gained momentum in the early 2000s. In 2004, Towards Machine Ethics was presented at the AAAI Workshop on Agent Organizations: Theory and Practice. This laid the theoretical foundations for the field.
Researchers met for the first time to consider implementing an ethical dimension in autonomous systems at the AAAI Fall 2005 Symposium on Machine Ethics. This symposium sparked a variety of perspectives on the nascent field of machine ethics.
In 2007, AI magazine published an article titled "Machine Ethics: Creating an Ethical Intelligent Agent". This article demonstrated that it is possible for a machine to abstract an ethical principle from examples of ethical judgments and use that principle to guide its behavior.
The publication of Moral Machines, Teaching Robots Right from Wrong in 2009 marked a significant milestone in the field of machine ethics. This book, published by Oxford University Press, examined the challenge of building artificial moral agents and cited 450 sources.
The US Office of Naval Research announced grants to study machine ethics in 2014. This investment recognized the importance of machine ethics in developing autonomous robots.
Here's an interesting read: Ethical Implications of Ai
Definitions
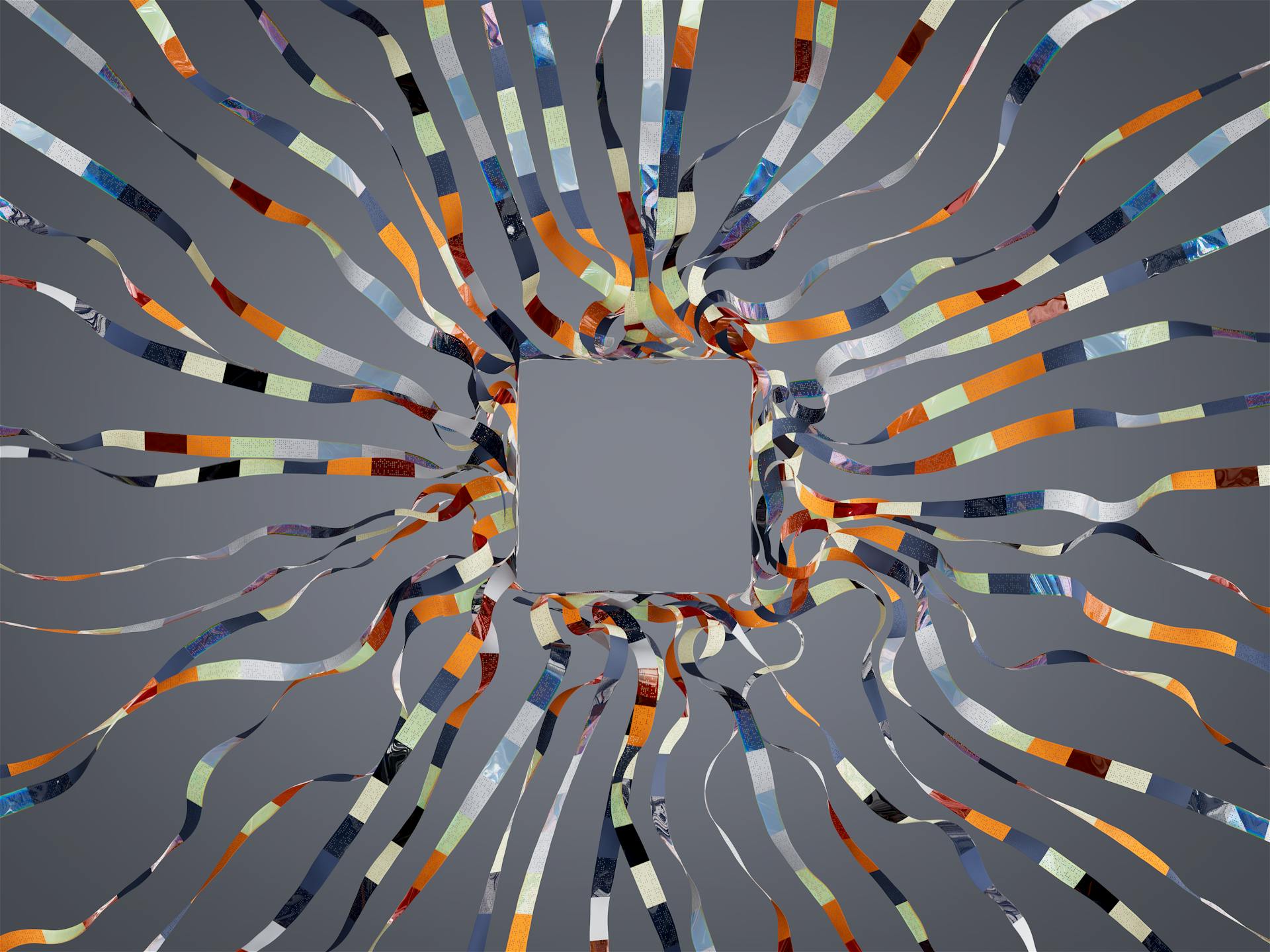
Computer ethics is a fascinating field that's been studied by pioneers like James H. Moor. He's defined four kinds of ethical robots that are worth understanding.
Moor's definitions are based on his extensive research in philosophy of artificial intelligence, philosophy of mind, philosophy of science, and logic. These definitions help us understand the different types of machines that can have an impact on our lives.
A machine can be classified as an ethical impact agent, implicit ethical agent, explicit ethical agent, or full ethical agent. Here's a breakdown of each type:
- Ethical impact agents: These machines carry an ethical impact whether intended or not and have the potential to act unethically.
- Implicit ethical agents: These agents are programmed to have a fail-safe or a built-in virtue to ensure human safety and avoid unethical outcomes.
- Explicit ethical agents: These machines are capable of processing scenarios and acting on ethical decisions, with algorithms to guide their actions.
- Full ethical agents: These machines are similar to explicit ethical agents but also possess human metaphysical features like free will, consciousness, and intentionality.
In 2019, the Proceedings of the IEEE published a special issue on Machine Ethics, which included papers on implicit and explicit ethical agents.
Control and Ownership
The issue of control and ownership is murky when it comes to AI-generated art. Human creators generate digital art through AI systems developed by others, leaving unclear who owns the art and who can commercialize it.
Lawmakers must clarify ownership rights to navigate potential infringements, as the current lack of guidelines creates uncertainty.
Ownership
Ownership is a murky issue in the realm of AI-generated art. As AI advances faster than regulators can keep up, lawmakers must clarify ownership rights.
The current state of affairs is exemplified by a painter completing a painting, in which they own it outright. But with AI-generated art, it's not so clear.
Human creators generate digital art through AI systems developed by others, leaving questions about ownership and commercialization. This issue is still evolving as AI advances.
Lawmakers must provide guidelines to navigate potential infringements and define who can commercialize AI-generated art. This is crucial for creators and developers alike.
Control Problem
The AI control problem is a pressing concern in the field of artificial intelligence. Scholars like Bostrom and Stuart Russell warn that a superintelligent AI could become powerful and difficult to control.
This is because a superintelligence could potentially seize power over its environment and prevent us from shutting it down. The danger of not designing control right "the first time" is significant.
Capability control is a potential strategy to limit an AI's ability to influence the world. This approach aims to prevent a superintelligence from causing harm.
Motivational control is another way to build an AI whose goals are aligned with human or optimal values. This involves designing an AI that is motivated to act in ways that benefit humanity.
The Future of Humanity Institute, the Machine Intelligence Research Institute, and the Center for Human-Compatible Artificial Intelligence are just a few organizations researching the AI control problem. They are working to develop strategies to ensure that AI is developed in a way that benefits humanity.
Check this out: Ai Danger to Humanity
Related Fields
Control and Ownership is a complex topic that intersects with various fields of study. Affective computing, for instance, explores how artificial intelligence can recognize and respond to human emotions, which raises questions about control and ownership in AI systems.
Bioethics is another related field that examines the moral implications of emerging technologies, including AI. Formal ethics, on the other hand, provides a framework for analyzing and resolving ethical dilemmas in AI development.
Computational theory of mind aims to create AI systems that can simulate human thought processes, which has significant implications for control and ownership. Computer ethics, meanwhile, focuses on the moral principles guiding the development and use of AI.
Ethics of artificial intelligence is a broad field that encompasses many of the issues related to control and ownership. Formal ethics provides a structured approach to addressing these issues.
Moral psychology studies how people make decisions about right and wrong, which is essential for understanding control and ownership in AI systems. Philosophy of artificial intelligence and philosophy of mind both explore the nature of intelligence and consciousness, which are critical to discussions about control and ownership.
Here are some of the related fields in a concise list:
- Affective computing
- Bioethics
- Computational theory of mind
- Computer ethics
- Ethics of artificial intelligence
- Formal ethics
- Moral psychology
- Philosophy of artificial intelligence
- Philosophy of mind
Frequently Asked Questions
What is the moral problem of AI?
The moral problem of AI lies in its potential to perpetuate and amplify biases, leading to unfair and discriminatory outcomes. Ensuring fairness and addressing bias in AI systems is a critical ethical concern that requires careful consideration and mitigation.
Sources
- https://www.coe.int/en/web/bioethics/common-ethical-challenges-in-ai
- https://www.captechu.edu/blog/ethical-considerations-of-artificial-intelligence
- https://en.wikipedia.org/wiki/Machine_ethics
- https://stefanini.com/en/insights/articles/the-moral-and-ethical-implications-of-artificial-intelligence
- https://archive.philosophersmag.com/the-ethics-of-ai-and-the-moral-responsibility-of-philosophers/
Featured Images: pexels.com