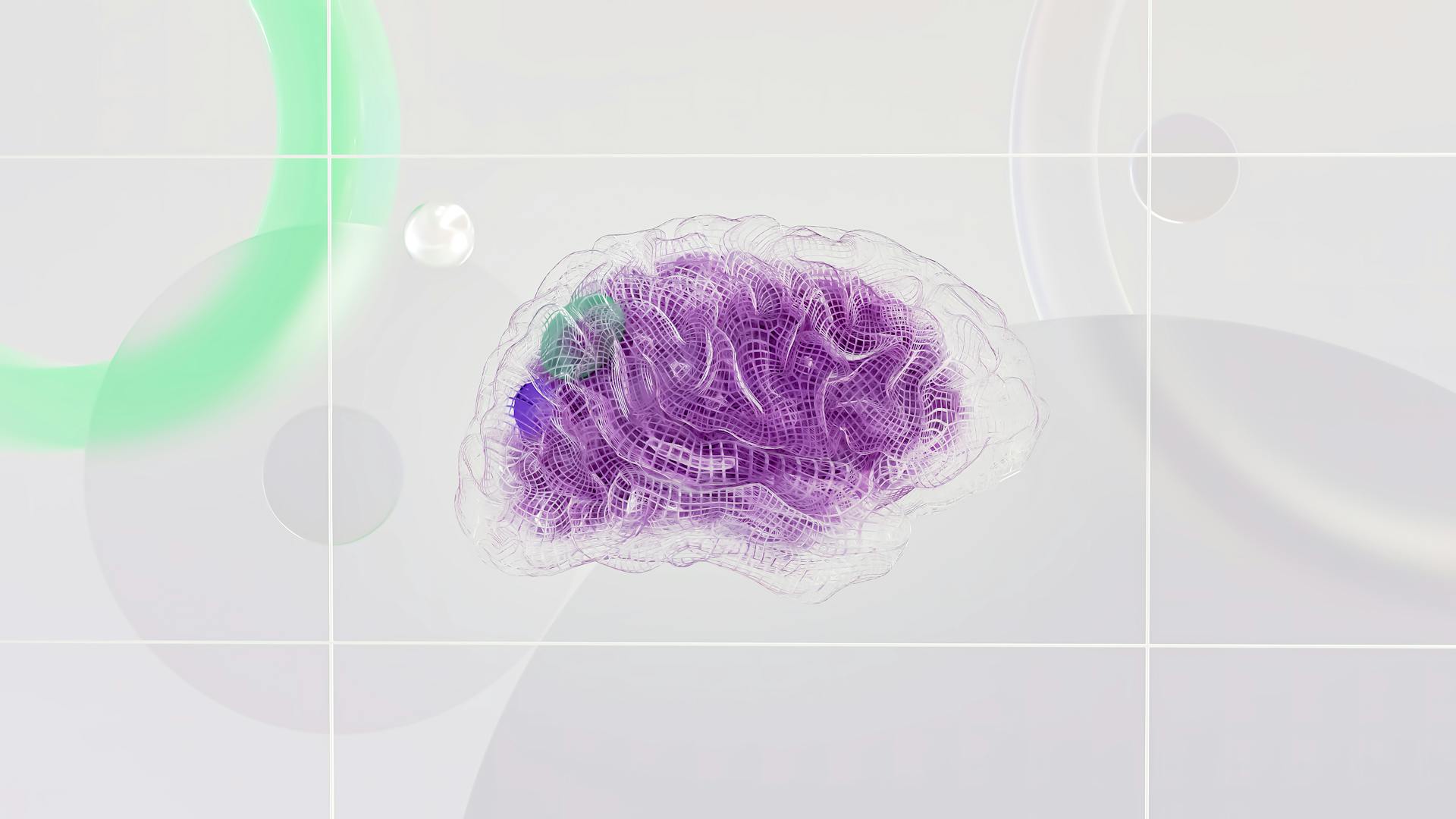
Machine learning is revolutionizing the manufacturing industry, enabling companies to boost efficiency, reduce costs, and improve product quality. By leveraging machine learning algorithms, manufacturers can analyze vast amounts of data from various sources, including sensors, machines, and IoT devices.
This data-driven approach allows for predictive maintenance, which can help prevent equipment failures and reduce downtime. For instance, a study cited in our article found that predictive maintenance can reduce equipment failures by up to 80%.
Manufacturers can also use machine learning to optimize production processes, leading to increased productivity and reduced waste. By analyzing production data, companies can identify bottlenecks and optimize their workflows to improve overall efficiency.
By adopting machine learning in manufacturing, companies can gain a competitive edge in the industry, driving growth and innovation.
A different take: Top Machine Learning Applications at Fin Tech Companies
Applications of Machine Learning in Manufacturing
Machine Learning can help manufacturers optimize their use of resources, such as energy and oil. By using predictive analytics, process engineers can test and identify processes that reduce waste and increase efficiency.
Manufacturers like Cargill have already seen success with Machine Learning, using it to run their oil boiler more efficiently. They were able to find faster solutions to their problems by putting Braincube's insights in front of their analysts.
Predictive analytics can also help identify optimum operating set points to optimize throughput. This allows process engineers to isolate areas of improvement without negatively impacting the process downstream.
Machine Learning will continue to play a key role in driving sustainability efforts forward in manufacturing. Companies are looking for ways to reduce their environmental impact, and Machine Learning provides a valuable opportunity to do so.
Examples of Successful Implementation
In the manufacturing industry, machine learning has been successfully implemented in various ways. A predictive maintenance solution for a hydraulic press in an automotive plant increased overall equipment efficiency from 65% to 85%.
Engineers at this plant were able to predict equipment failure with an accuracy of 92%, allowing them to plan maintenance more effectively and offer greater asset reliability and product quality. This resulted in significant time savings.
The application of machine learning also helped a beverage industry manufacturer optimize their equipment maintenance schedules, which had previously been based on defined time intervals rather than real needs. This led to increased business scalability and a more optimized cost structure.
For another approach, see: Impact of Generative Ai on Tax Industry
General Motors
General Motors is a great example of a company that's successfully implemented machine learning in its manufacturing process. The effective price growth of an average vehicle has remained flat since 1998, making efficiency crucial.
GM and Autodesk engineers used generative design technology to reconceive a small vehicle component, the seat bracket. They set parameters like required connection points, strength, and mass, and the software produced over 150 valid design options.
These design options included an organic structure that no human could have imagined. The new seat bracket is 40 percent lighter and 20 percent stronger than the original.
The new design consolidates eight different components into one 3D-printed part, which is a significant benefit of generative design. This innovative approach has given GM a competitive edge in product development.
For another approach, see: Google Announces New Generative Ai Search Capabilities for Doctors
Rolls-Royce
Rolls-Royce has taken a significant step forward in their aircraft design partnership with Altair, incorporating machine learning into their manufacturing processes. This move is expected to reduce the number of sensors required for testing, decreasing development time and costs.
By integrating machine learning, Rolls-Royce aims to reduce development time and costs, allowing their products to reach the market faster. This is a crucial aspect of their IntelligentEngine vision.
The IntelligentEngine technology enables a jet engine to communicate with and be aware of other engines, the support ecosystem, and the customer airline. This level of awareness allows the engine to learn from its experiences and optimize its performance.
Rolls-Royce's use of machine learning is a prime example of how this technology can be applied in a real-world setting. By leveraging machine learning, they are able to improve their products and reduce costs.
Leveraging AI During Covid-19
Leveraging AI During Covid-19 can help manage production uncertainty by analyzing data quickly and effectively.
The power to analyze data quickly and effectively requires AI, which can make a big difference in uncertain times like a global pandemic.
With a global pandemic still ongoing, the uncertainty surrounding supply, demand, staffing, and more continues to impact industrials.
No one could have predicted the path 2020 would take.
The answer to managing production during Covid-19 lives within your data, and AI can help you make sense of it.
Undefined cookies are those that are being analyzed and have not been classified into a category as yet.
Discover more: Data Labeling for Machine Learning
Types of Applications
Machine learning in manufacturing has a wide range of applications, from data gathering to predictive analytics.
Machine learning can be used for sector-specific use cases, such as predictive maintenance, product quality control, and inventory management.
Predictive analytics can help uncover hidden improvement opportunities by detecting patterns, preventing downtime, and reducing costs.
Technologies Used
In the world of application development, several technologies are used to bring ideas to life.
Mobile apps often rely on native technologies like Java and Swift, which are used to create Android and iOS apps, respectively.
Cross-platform development tools like React Native and Flutter are also popular, allowing developers to create apps for multiple platforms using a single codebase.
Database management systems like MySQL and MongoDB are used to store and manage data in web applications.
Cloud computing services like Amazon Web Services (AWS) and Microsoft Azure are used to host and manage web applications, providing scalability and reliability.
If this caught your attention, see: Software Design and Development
Use Cases Across Sectors
Machine learning is being used across various manufacturing sectors, each with its own unique use cases. Predictive maintenance is a key application in multiple sectors.
In the automotive industry, a hydraulic press used in vehicle panel production was equipped with a predictive maintenance solution, which increased overall equipment efficiency from 65% to 85%. This solution also enabled engineers to predict equipment failure with an accuracy of 92%.
In the beverage industry, a manufacturer of industrial equipment implemented a monitoring and prediction system to optimize equipment maintenance schedules. This led to increased business scalability and a more optimized cost structure.
Predictive maintenance is also expected to play a crucial role in the development of autonomous vehicles, where cars will need to monitor their own condition and perform maintenance tasks without human intervention.
Siemens
Siemens has introduced its Anomaly Assistant app, which combines machine learning (ML) and AI development into a single efficient solution.
The app uses ML algorithms trained on process data to identify anomalies affecting a facility's economic efficiency.
A fresh viewpoint: Ai and Ml in Logistics
Plant operators can refocus the AI to identify the most relevant anomalies through the app dashboard, and analyze them repeatedly to develop a fully-trained AI.
The app can be installed as a cloud or within a customer's existing infrastructure, and is available on Amazon Web Services (AWS) and for on-premises deployments on SIMATIC Box PC or virtual machines (ESX, Hyper V).
The cloud-based strategy with AWS allows Siemens and its customers to communicate more successfully.
Working on the cloud can be especially beneficial during the review phase, enabling data analysts and plant operators to cooperate more effectively.
This app is a great example of how machine learning can be applied in manufacturing to improve efficiency and reduce costs.
Expand your knowledge: Building Publishing Iphone App Guide
Cost Reduction
Cost Reduction is a crucial aspect of manufacturing, and machine learning is helping companies like Vistra achieve significant savings. Efficiency increased by 1% at Vistra, translating into millions of dollars in savings and reduced greenhouse gas emissions.
Predictive maintenance is another area where machine learning is making a big impact. By employing algorithms to forecast the next failure of a component, piece of equipment, or system, companies can avoid costly downtime and reduce waste. According to a PwC report, predictive maintenance will be one of the fastest-growing manufacturing machine learning assets, with a projected increase in its market value of 38% from 2020 to 2025.
Machine learning is also being used to identify hidden bottlenecks in manufacturing processes, opening up opportunities to create further efficiencies of resources and automation. This can help companies like General Motors, which has been using generative design technology to reconceive vehicle components, resulting in a 40% lighter and 20% stronger seat bracket.
Some of the key benefits of machine learning in cost reduction include:
- Reducing waste and minimizing losses through predictive maintenance and quality control
- Increasing efficiency and productivity by identifying and addressing bottlenecks
- Optimizing maintenance schedules and reducing the need for reactive maintenance
- Improving product quality and reducing the cost of testing and failures
By leveraging machine learning, companies can achieve significant cost savings and improve their overall competitiveness in the market.
Supply Chain
Supply chains are an essential part of manufacturing, and machine learning technologies are revolutionizing them. Cognitive supply chain management is enhancing areas such as demand forecasting.
Demand forecasting analyzes clients' behaviors and preferences using time series analysis, feature engineering, and natural language processing (NLP). This helps businesses make informed decisions about production and inventory.
Warehouse control enables rapid replenishment of goods by facilitating stock control with deep learning-based computer vision systems. This reduces the risk of stockouts and overstocking.
Logistics and transport optimization machine learning algorithms assess and allocate the most optimal routes for shipping and transportation solutions. This can lead to significant cost savings and reduced carbon emissions.
Industry Growth and Future
Manufacturing companies are now actively engaging with data scientists to tackle specific problems with machine learning. This collaborative approach is yielding promising results.
Bosch, a leading manufacturing company, recently hosted a competition on Kaggle to predict which manufactured parts would fail quality control. This competition aimed to identify causes of manufacturing defects and reduce waste by rejecting faulty components early on.
The results of this competition are expected to improve product quality and lower costs for Bosch.
Industry Growth
Manufacturing companies are now taking a more proactive approach to innovation, sponsoring competitions for data scientists to tackle specific problems with machine learning.
Bosch, a leading manufacturing company, recently hosted a competition on Kaggle, the global platform for data science contests, to predict which manufactured parts would fail quality control.
Companies are seeking to improve product quality and lower costs by leveraging machine learning to identify and prevent defects early on in the production process.
The competition required participants to analyze thousands of measurements and tests done on each component along the assembly line to make accurate predictions.
This approach is expected to support waste reduction by rejecting faulty components at early stages, reducing unnecessary production costs and improving overall efficiency.
Manufacturing companies are recognizing the potential of machine learning to drive growth and competitiveness, and are investing in initiatives like this competition to harness its power.
For another approach, see: Quantum Machine Learning Companies
Robotics-Powered Future
The robotics-powered future is looking bright. Modern manufacturing is still heavily reliant on human workers, but robots are changing that.
Machines can perform tasks with precision, but they struggle in changing environments. In the near future, robots will be able to adapt to these environments and work alongside humans.
Robotics provides a great opportunity for reinforcement learning, a machine learning technique that allows machines to adapt to changing environments and build complex strategies. This technique is currently the only way for machines to achieve goals that are unachievable with explicit programming.
Robots will be able to perform tasks in a more human way, making them a game-changer for manufacturing. They will be able to cooperate with humans and adapt to the object being produced.
Getting Started and Benefits
To start leveraging machine learning in your factories, identify clear learning and business objectives, connect your data to a powerful IIoT platform, and drive the shift to a more data-centric decision-making process.
Machine learning use cases have to bring tangible financial impact, so it's essential to address real business needs. Successful use case definition boils down to selecting the right use case, which can be done by starting small, deploying the machine learning tool on a single factory floor in a single production line before expanding to other facilities.
See what others are reading: Data Labeling in Machine Learning with Python
The impact of machine learning in manufacturing can be felt across departments, from improving the bottom line to solving some of the biggest challenges faced by manufacturers today. Machine learning is a useful tool for process engineers, data scientists, and analysts focused on continuous improvement.
Machine learning allows companies to automate a wide variety of tasks, making them more efficient and cost-effective. This is just one of the benefits of machine learning, which also includes less reliance on human interaction, a scope of improvement, efficient data handling, and a wide range of applications.
Here are some key benefits of machine learning in manufacturing:
- Automation: Machine learning allows companies to automate tasks, making them more efficient and cost-effective.
- Less reliance on human interaction: Machine learning relies almost exclusively on algorithms to get its work done.
- Scope of improvement: Machine learning algorithms are able to constantly build on their own bases of knowledge and functionality.
- Efficient data handling: Machine learning can analyze any type of data, making it incredibly useful for data analysis and data science.
- Wide range of applications: Machine learning is used in nearly every industry today, from healthcare to e-commerce.
Common Challenges and Solutions
One of the biggest challenges in implementing machine learning in manufacturing is data quality and availability. This can be due to the lack of historical data, inconsistent data formats, or even data being locked away in proprietary systems.
Manufacturers often struggle with integrating machine learning models with existing production systems, which can be a significant barrier to adoption.
Discover more: Data Labeling in Machine Learning with Python Pdf
However, there are solutions to these challenges. For example, using simulation-based approaches can help manufacturers generate synthetic data to train machine learning models, even when historical data is scarce.
Another common challenge is the high cost of implementing and maintaining machine learning systems, which can be a deterrent for small and medium-sized manufacturers.
Fortunately, cloud-based machine learning platforms can provide access to scalable and cost-effective solutions, making it more feasible for smaller manufacturers to adopt machine learning technologies.
In addition, manufacturers can also leverage open-source machine learning frameworks and libraries to reduce development costs and accelerate time-to-market for new applications.
For another approach, see: Pixel Based Websites
Implementation and Logistics
Implementing machine learning in manufacturing requires careful planning and consideration of various factors.
Data collection is a crucial step in the process, as it provides the necessary information for training and testing machine learning models.
The type of data collected can vary depending on the specific application, but it often includes sensor readings, production metrics, and quality control data.
In the case of predictive maintenance, for example, sensor data from machines can be used to identify potential issues before they occur.
Implementing machine learning models in manufacturing can be done through various platforms, including cloud-based services and on-premise software.
Cloud-based services like Amazon Web Services and Google Cloud Platform offer scalable and secure infrastructure for machine learning applications.
On-premise software, on the other hand, provides more control over data and security, but requires significant upfront investment in hardware and infrastructure.
The choice between cloud-based and on-premise solutions depends on the specific needs and resources of the manufacturing organization.
In the case of a small to medium-sized manufacturer, a cloud-based service might be a more cost-effective option.
Frequently Asked Questions
What is generative AI use case in manufacturing?
Generative AI in manufacturing enables real-time data analysis to create accurate digital representations of products, production lines, and factories. This allows manufacturers to improve design, test new processes, and create new products more efficiently.
Sources
- https://www.itransition.com/machine-learning/manufacturing
- https://pixelplex.io/blog/machine-learning-in-manufacturing/
- https://braincube.com/resource/use-machine-learning-in-manufacturing/
- https://deepsense.ai/machine-learning-for-applications-in-manufacturing/
- https://bootcamp.cvn.columbia.edu/blog/machine-learning-applications/
Featured Images: pexels.com