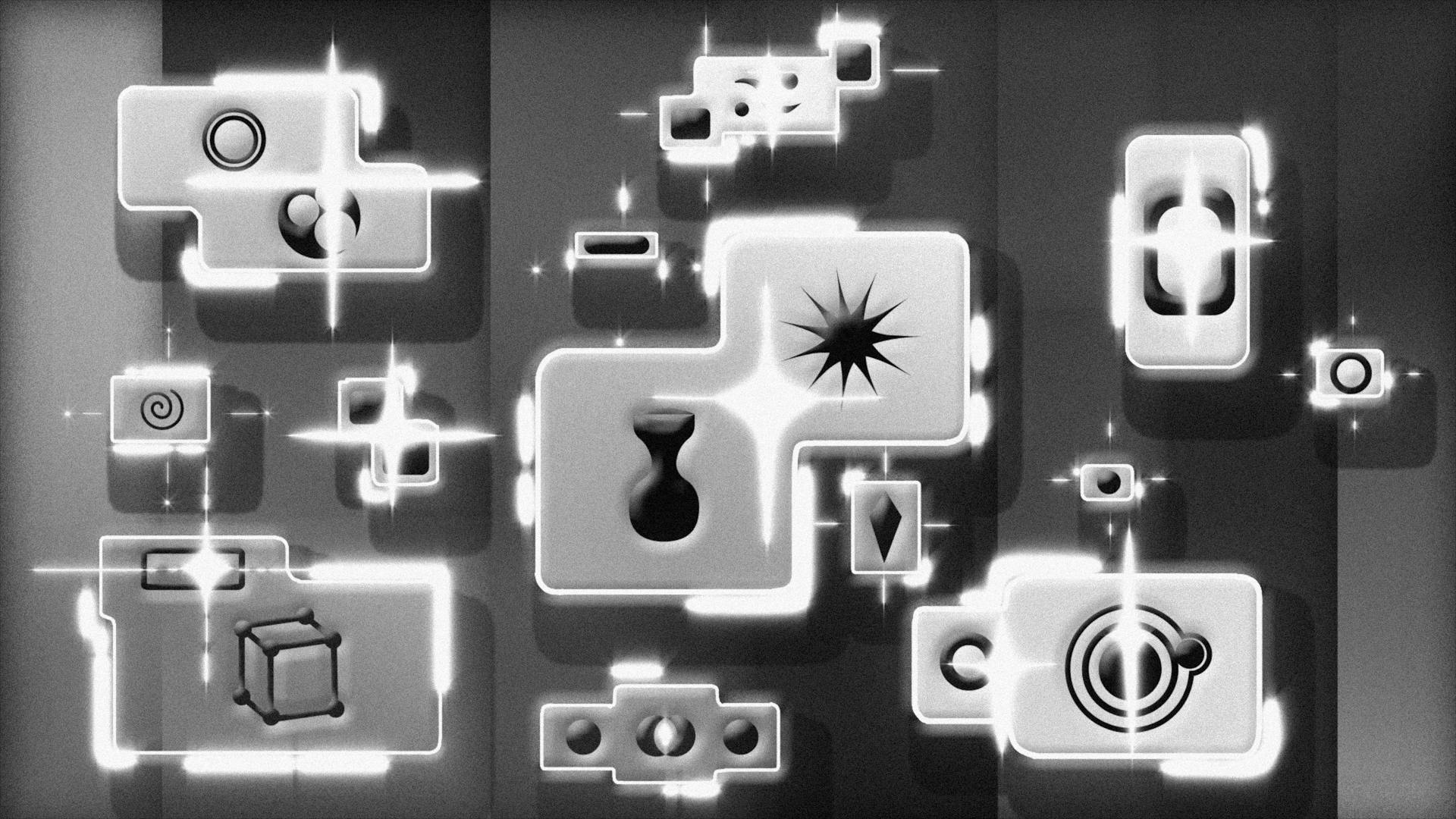
Generative AI research papers have been making waves in the tech world, and for good reason. They're pushing the boundaries of what's possible with AI, and the results are nothing short of impressive.
One of the key methods being explored is Generative Adversarial Networks (GANs), which have been shown to produce remarkably realistic images and videos. Researchers have also been experimenting with Variational Autoencoders (VAEs) and Generative Moment Matching Networks (GMNNs) to improve the quality and diversity of generated content.
These methods are being evaluated using metrics such as Inception Score (IS) and Fréchet Inception Distance (FID), which measure the similarity between generated and real data. Researchers are also using human evaluation and self-supervised learning to assess the quality and coherence of generated outputs.
The applications of these techniques are vast and varied, from generating realistic faces and objects to creating new music and art. Researchers are also exploring the use of generative models for data augmentation and anomaly detection.
Curious to learn more? Check out: Generative Ai Photoshop Increase Quality
Research Methods
Researchers have employed various Generative Adversarial Network (GAN) methods for image translation tasks, including SAR image translation. Specifically, both paired and unpaired GANs have been used for this application.
The SAR-generated images are not very visually clear, but they can be captured at any time, making them a better technique compared to optical imaging. Particularly, when combined with GANs image translation methods, SAR-generated images can be converted into optical high-resolution clear images.
Researchers have proposed different GANs methods for unpaired image translation, such as CycleGAN, NICE GANs, Attn-CycleGAN, and paired GANs like Pix2Pix and Bicycle GANs, for Satellite image translation.
See what others are reading: Getty Images Nvidia Generative Ai Istock
Inclusion Criteria
To ensure the accuracy and reliability of our research, we focus on incorporating peer-reviewed papers, conference, and journal papers written in English. This helps us tap into the most credible sources of information.
We emphasize studies that highlight significant advancements or innovative applications in Generative AI, which allows us to focus on cutting-edge developments within this field. This ensures that our research stays current and impactful.
Our inclusion criteria are designed to provide a solid foundation for our research, allowing us to build upon the most relevant and influential studies in the field.
Curious to learn more? Check out: Guidance for Generative Ai in Education and Research
Exclusion Criteria
To ensure the accuracy and relevance of our research, we meticulously filter our sources. We exclude non-peer-reviewed materials, which are often unreliable or biased.
Papers not written in English are also excluded, as they may not be easily understood by our global audience. This helps us to maintain a consistent and accessible research discourse.
Studies that fall outside the 2012-2023 timeframe are deliberately omitted, allowing us to focus on the most recent and relevant developments in our field. This ensures that our research is up-to-date and applicable to current challenges.
Only papers that directly contribute to the advancement or understanding of Generative AI are included, ensuring a focused and relevant academic discourse. This helps us to avoid unnecessary distractions and stay on track with our research goals.
You might like: Generative Ai Not Working Photoshop
Evaluation Criteria
In evaluating the advancements in Generative AI techniques, researchers compare the performance of various models using standardized datasets commonly cited in the field.
These datasets provide a clear and consistent basis for assessing the progress and effectiveness of these techniques in their respective domains.
To conduct a comprehensive evaluation, researchers focus on how different state-of-the-art models perform on these datasets.
Here are the key evaluation criteria:
- Performance on standardized datasets
- Comparison of state-of-the-art models
- Assessment of progress and effectiveness
By using these evaluation criteria, researchers can gain a deeper understanding of the strengths and weaknesses of different Generative AI techniques and identify areas for future improvement.
Autoencoder
Autoencoders are a type of model in the field of generative AI. They consist of an encoder and a decoder, with the purpose of the encoder being to encode the given input in a lower dimension called latent space.
The foundational work of Variational Autoencoders was done by Kingma et al. in [75]. Variational Autoencoders is one of the oldest techniques of unsupervised learning and generative modeling. They combine probabilistic modeling with the basics of Autoencoders, allowing them to learn the properties of the latent space and generate new synthetic data samples.
Early variants of autoencoders include denoising autoencoders, which use denoising techniques while trained locally to get rid of corrupted versions of their inputs [150]. The pioneering work of Kingma et al. has paved the way for numerous subsequent developments and applications of VAEs in a wide range of domains.
Variational Autoencoders have been used in image generation, natural language processing, and more. They learn the probabilistic distribution of the latent space, which gives them the ability to generate new synthetic data samples.
Intriguing read: New Generative Ai
Datasets and Evaluation
When evaluating generative AI models, especially in image translation, researchers use specialized datasets. These datasets are uniquely designed to challenge and evaluate the models' abilities to accurately and effectively translate images.
The performance of generative AI models is typically assessed using standardized datasets commonly cited in the field. Two notable datasets stand out in the field of image translation: ImageNet and ClebA.
Researchers must properly cite the source of the dataset in their work, and for datasets containing medical data, they are required to sign a Data Use Agreement. This agreement sets forth guidelines on the appropriate usage and security of the data and strictly prohibits any attempts to identify individual patients.
In the field of medical science, notable datasets used for image translation include MIMIC, BRATS, FastMRI, and ChestX-ray. These datasets are freely accessible to researchers for testing their models, under certain conditions.
Here are some notable datasets used for image translation:
- ImageNet
- ClebA
- MIMIC
- BRATS
- FastMRI
- ChestX-ray
Sar Image
Synthetic Aperture Radar (SAR) images are not visually clear, but they can be captured at any time, making them a better technique than optical imaging.
Researchers have used Generative Adversarial Networks (GANs) to translate SAR images into optical high-resolution clear images.
The techniques of paired and unpaired GANs are used for SAR image translation, with methods like CycleGAN, NICE GANs, and Pix2Pix being employed.
A new technique called Cross-Fusion Reasoning and Wavelet Decomposition GAN (CFRWD-GAN) was introduced to specifically translate unpaired SAR images to optical images.
CFRWD-GAN's primary objective is to retain structural intricacies and elevate the quality of high-frequency band details, achieved through a unique framework that integrates cross-fusion reasoning and discrete wavelet decomposition.
This method enables the translation of high-frequency components and addresses speckle noise inherent in SAR images.
CFRWD-GAN was evaluated using metrics like Root Mean Squared Error (RMSE) and Structural Similarity Index (SSIM), producing a better result than other state-of-the-art models.
Related reading: How Multimodal Used in Generative Ai
Artificial Intelligence
Generative AI is a type of machine learning that generates new data samples that mimic existing datasets. These models use advanced algorithms to learn patterns and generate new content such as text, images, sounds, videos, and code.
One of the foundational techniques in GenAI is the Variational Autoencoder (VAE), a type of neural network that learns to encode and decode data in a way that maintains its essential features. This technique was first introduced by Kingma & Welling in 2013.
Generative AI models have been trained on a diverse range of texts, including books, articles, and websites, allowing them to understand user input, generate responses, and maintain coherent conversations on a wide range of topics. ChatGPT, a conversational AI system developed by OpenAI, is a notable example of a GenAI model that has been trained on a large corpus of text data.
Generative AI encompasses various types, each tailored for specific tasks or forms of media generation. Some of the more well-known types include Generative Adversarial Networks (GANs), Transformer-based Models (TRMs), and Diffusion models (DMs).
For more insights, see: Can I Generate Code Using Generative Ai Models
What Is AI?
Artificial Intelligence (AI) refers to a broad range of technologies that enable machines to perform tasks that typically require human intelligence.
AI systems can be trained on vast amounts of data, allowing them to acquire an understanding of patterns and structures within that data.
Generative Artificial Intelligence is a type of AI that can create new data, such as text or images, by learning from existing data.
Generative models are the core component of Generative Artificial Intelligence, enabling AI systems to generate novel data with similar characteristics.
Some well-known types of Generative Artificial Intelligence include Generative Adversarial Networks (GANs), Transformer-based Models (TRMs), Variational Autoencoders (VAEs), and Diffusion models (DMs).
These types of AI are tailored for specific tasks or forms of media generation, and will be discussed in more detail in the following sections.
AI has the potential to revolutionize various industries, from healthcare to finance, by automating tasks and making predictions based on data.
Curious to learn more? Check out: How Are Modern Generative Ai Systems Improving User Interaction
Artificial Intelligence
Generative Artificial Intelligence refers to artificial intelligence systems with the capability to create text, images, or other forms of media through the utilization of generative models.
These models acquire an understanding of patterns and structures within their training data, subsequently generating novel data with akin characteristics.
Generative AI encompasses various types, each tailored for specific tasks or forms of media generation, such as Generative Adversarial Networks (GANs), Transformer-based Models (TRMs), Variational Autoencoders (VAEs), and Diffusion models (DMs).
ChatGPT is a conversational AI system that has caused a surge of interest in the use of GenAI in higher education since its release in November 2022.
It is a large language model that has been pre-trained on a large corpus of text data, allowing it to understand user input, generate responses, and maintain coherent conversations on a wide range of topics.
Generative AI models use advanced algorithms to learn patterns and generate new content such as text, images, sounds, videos, and code.
For more insights, see: Generative Ai by Getty
Some examples of GenAI tools include ChatGPT, Bard, Stable Diffusion, and Dall-E.
Its ability to handle complex prompts and produce human-like output has led to research and interest into the integration of GenAI in various fields such as healthcare, medicine, education, media, and tourism.
The technique of paired GANs is used for image translation, and the SAR-generated images are not very visually clear but they can be captured at any time.
Different GANs methods of unpaired image translation are used for Satellite image translation, such as CycleGAN, NICE GANs, Attn-CycleGAN, and paired GANs such as Pix2Pix, and Bicycle GANs.
The CFRWD-GAN model was evaluated using Root Mean Squared Error (RMSE), structural similarity index (SSIM), peak signal-to-noise ratio (PSNR), learned perceptual image patch similarity (LPIPS), and produced a better result than the other state of the art models.
Variational Autoencoders (VAE) is another technique for image generation and translation, developed by Kingma et al.
The best-performing model in a study comparing generative capabilities of Conditional Variation Autoencoders was the Bicycle GANs.
A unique perspective: Chatgpt Openai's Generative Ai Chatbot Can Be Used for
Adversarial Networks
Generative Adversarial Networks (GANs) are a type of neural network that consists of two networks working in competition to generate realistic data samples.
GANs are used in various applications, including image translation, where they can convert SAR-generated images into optical high-resolution clear images.
One example of a GAN used for image translation is the CycleGAN method, which is used for unpaired image translation.
Another example is the Pix2Pix method, a paired GAN used for image translation.
The CFRWD-GAN model, introduced by Wei et al., is a new technique specifically designed for the translation of unpaired SAR images to optical images.
This model uses a unique framework that integrates cross-fusion reasoning and discrete wavelet decomposition to effectively retain structural intricacies and elevate the quality of high-frequency band details.
The CFRWD-GAN model was evaluated using metrics such as Root Mean Squared Error (RMSE), structural similarity index (SSIM), and peak signal-to-noise ratio (PSNR), and produced a better result than other state-of-the-art models.
A fresh viewpoint: Which Is an Example Limitation of Generative Ai Interfaces
GANs, including AC-GAN, are also used in semi-supervised synthesis, where they can seamlessly integrate label information into the generator and adapt the discriminator's objective function accordingly.
AC-GANs have noticeable enhancements in the generative and discriminative capabilities of the GAN framework.
GANs are a powerful tool in the field of artificial intelligence, and their applications continue to expand into various fields, including image translation and semi-supervised synthesis.
Transformer
Transformers are a revolutionary step in the field of generative AI, specifically in natural language processing and generating synthetic content.
The concept of Transformers was introduced by Vaswani et al. [134], a breakthrough architecture that laid the foundation for various tasks, including machine translation and language generation.
Transformers use both self-attention and Multi-Head Attention mechanisms to learn the dependencies between objects regardless of distance, and to learn different relations and patterns between the input.
The paper's emphasis on attention mechanisms highlighted their pivotal role in sequence-to-sequence tasks, advancing the state of the art.
Readers also liked: Generative Ai Transformers
Transformers are commonly used to build Generative AI Models such as Generative Pre-trained Transformers (GPT) models, which are capable of generating coherent and contextually relevant text.
Bidirectional Encoder Representations from Transformers (BERT) and Open AI GPT are based on transformers.
The basic concept of the Transformers was introduced by Sutskever et al. [125] as a sequence modelling technique.
The basic technique of pretraining transformers was introduced and used as a state-of-the-art technique by Qiu et al. [119].
This technique was used in answering different queries and also used as a chatbot to give results that are competitive and accurate.
Indeed, these early Transformer Developments paved the way for State-of-the-Art NLP Chatbots.
Worth a look: Generative Ai for Cybersecurity
Molecule
Artificial Intelligence has made tremendous progress in generating molecular structures. VAE was used for molecule generation, creating 3-Dimensional synthetic molecule structures.
Researchers have developed generative algorithms specifically for predicting the structure of molecules and proteins. This approach is considered the best in generative AI techniques for generating and predicting molecular architectures.
Jumper et al. developed an architecture for a generative algorithm that can predict molecular structures. This is a significant breakthrough in AI research.
Generative AI Models
Generative AI models have come a long way, with various techniques emerging to improve their performance. One such technique is the Diffusion Models, introduced by Salimans et al, which aims to learn complex probability distributions by transforming a simple base distribution into the target distribution through a series of invertible transformations.
The Diffusion Models have been designed to improve the performance of the Simple Generative Adversarial Network. A variant of the diffusion model called Inverse Autoregressive Flow (IAF) was introduced by Kingma et al as a building block for generative models. IAF is a type of normalizing flow that can learn complex probability distributions.
Other generative AI models, such as Variational Autoencoders (VAEs), have also been developed. VAEs consist of an encoder and a decoder, and their main goal is to achieve the output with a similar mean and variance as the given input after the introduction of the variance. This provides a structured way to learn meaningful representations of data and then generate new samples from that data distribution.
Check this out: Generative Ai in Performance Testing
Variational Autoencoders
Variational Autoencoders are a type of generative AI model that can learn meaningful representations of data and generate new samples from that data distribution. Introduced by Kingma et al., VAEs consist of an encoder and a decoder, which work together to compress and decompress data.
The encoder's purpose is to encode the given input in a lower dimension called latent space. This latent space is a lower-dimensional representation of the input data. Variation is introduced to the latent space by using the standard Gaussian distribution.
During the process, the main goal is to achieve an output with a similar mean and variance as the given input after the introduction of the variance. This provides a structured way to learn meaningful representations of data. The best-performing model in one study was the Bicycle GANs, which was compared to Conditional Variation Autoencoders and other generative techniques for image generation and translation.
VAEs can be used for image translation, as seen in the work of Kingma et al. and Zhu et al., who compared the generative capabilities of Conditional Variation Autoencoders and other generative techniques.
Diffusion Models
Diffusion Models are a type of generative model designed to improve the performance of Simple Generative Adversarial Networks.
They were introduced by Salimans et al and have been shown to be effective in learning complex probability distributions.
One variant of the diffusion model is Inverse Autoregressive Flow (IAF), a type of normalizing flow that aims to learn complex probability distributions by transforming a simple base distribution into the target distribution through a series of invertible transformations.
IAF was introduced by Kingma et al as a building block for generative models.
Diffusion-based models employ a sequential diffusion process to iteratively transform simple data distributions into complex, high-dimensional ones.
This process was first introduced by NICE, which introduced the concept of invertible transformations as a foundation for generative artificial intelligence.
Real NVP expanded on these ideas by incorporating neural networks into the transformation process.
Glow extended these ideas to high-resolution image generation, highlighting the potential of diffusion-based models in computer vision.
Diffusion Probabilistic Models (DPMs) leveraged the diffusion process to model the likelihood of data samples, making it an essential contribution to the development of diffusion-based generative models.
Gan
Generative AI models, specifically Generative Adversarial Networks (GANs), have been a game-changer in the field of artificial intelligence.
GANs were initially plagued by the convergence problem, but researchers like Mescheder et al. [101] and Nagarajan et al. [106] worked to address this issue by analyzing local convergence and stability properties during training.
The Wasserstein GAN (W-GAN) was introduced by Goodfellow et al. [49] to improve GAN training stability by using the EarthMover distance instead of the Jensen-Shannon divergence.
Diffusion-based models, such as Non-Linear independent component estimation (NICE), have also been explored in the field of generative AI.
GANs have been used in various applications, including image translation, where the Cyclic Generative Adversarial Network (Cyclic GAN) was developed to address the challenge of unpaired image translation.
Variational Autoencoders (VAEs) have also been used in image translation, with Zhu et al. [177] comparing the generative capabilities of Conditional Variation Autoencoders.
GANs have shown promise in generating high-quality synthetic data, which can be used to train other AI systems and improve their performance and generalizability.
A fresh viewpoint: Generative Ai Training
Researchers have also explored the use of GANs in healthcare, such as in the creation of synthetic data for liver lesion classification using DCGAN.
GANs have also been used in image-to-image translation tasks, where the UVCGAN model produced better results than previous simple cyclic models.
GANs have the potential to revolutionize various fields, including computer vision, healthcare, and more, by generating high-quality synthetic data and improving the performance of other AI systems.
Explore further: Synthetic Data Generative Ai
Applications of Generative AI
Generative AI has numerous applications, particularly in data generation. It has shown significant promise in enhancing synthetic data generation through the use of Transformers and Generative Adversarial Networks (GANs).
In healthcare, researchers have utilized GANs to create synthetic data for liver lesion classification. This is a crucial step in developing AI models that can accurately diagnose medical conditions.
AI models can also generate synthetic data for counting the number of people in a crowd, as demonstrated by Wang et al. Their dataset, entirely synthetically generated, included various weather conditions, enabling their model to outperform state-of-the-art models.
Highlights
Generative AI is being explored in higher education settings, with a focus on its potential benefits and challenges.
A recent study surveyed 399 undergraduate and postgraduate students in Hong Kong, revealing a generally positive attitude towards generative AI in teaching and learning.
The integration of generative AI technologies, like ChatGPT, is gaining attention in higher education, with a need for well-informed guidelines and strategies for responsible implementation.
Researchers are advocating for a reevaluation of the roles played by human educators and AI within the educational realm, with a focus on adopting a forward-thinking outlook that considers the contributions of both technology and human educators.
A new and creative way of teaching is needed to effectively incorporate the progress brought by AI, with a focus on cultivating an ethical and personalized chatbot solution.
Here are some key takeaways from the study:
- Surveyed students showed a generally positive attitude towards generative AI in teaching and learning.
- A total of 399 undergraduate and postgraduate students from various disciplines in Hong Kong participated in the survey.
- The study aimed to explore students' perceptions of generative AI technologies in higher education.
Medical
Generative AI has been making waves in the medical field, particularly in image translation. Medical professionals can now discern critical information more effectively thanks to AI-driven image translation.
AI-powered image translation can produce images that are more polished and precise, allowing doctors to make more accurate diagnoses. This is achieved through the use of Generative Adversarial Networks (GANs) and Transformers, such as the Swin Transformers.
The Swin-based Transformers method has shown remarkable performance in converting T1 mode to T2 mode images using the clinical brain MRI dataset. It even outperformed other methods like Pix2Pix, CycleGAN, and RegGAN in translating images.
Conditional GANs have also been used to solve the problem of image translation in MRI, where the generator takes an input image and a target condition as input to generate an output image that adheres to the specified condition.
Generative AI has also shown promise in generating synthetic data for medical applications, such as liver lesion classification. This can help overcome privacy issues and the limitations of small or biased datasets.
Synthetic data can be artificially generated using Generative AI models, which can create high-quality synthetic data to train other AI systems. This can improve their performance and generalizability in various applications, including medical research.
Natural Language Processing
Natural Language Processing is a crucial area where Generative AI has made significant strides. Transformers, introduced by Vaswani et al., have revolutionized this field.
Transformers use both self-attention and Multi-Head Attention mechanisms to learn dependencies between objects regardless of distance. These methods are commonly used in Natural Language Processing tasks.
The Transformers architecture has been influential in machine translation and language generation. Its emphasis on attention mechanisms has advanced the state of the art in sequence-to-sequence tasks.
Generative Pre-trained Transformers (GPT) models, capable of generating coherent and contextually relevant text, are based on Transformers. These models have been widely used in various applications.
Bidirectional Encoder Representations from Transformers (BERT) and Open AI GPT are also based on Transformers. They have been successful in various Natural Language Processing tasks.
For another approach, see: Introduction to Generative Ai with Gpt
Challenges and Opportunities
Generative AI presents both challenges and opportunities across various domains. One of the key challenges is ethical concerns, particularly interpretability.
The advancement of generative AI holds promising prospects, with significant growth expected through ongoing innovations in both its architecture and practical applications.
As research in generative AI continues to evolve, it's essential to address the challenges head-on to unlock its full potential.
Here's an interesting read: What Challenges Does Generative Ai Face
Challenges and Opportunities
Generative AI is a rapidly evolving field with both exciting opportunities and pressing challenges.
One of the key challenges is the issue of interpretability, which refers to the ability to understand how the AI model is making its decisions. This is a significant concern, as it can be difficult to trust a model if we don't know how it's working.
The advancement of generative AI holds promising prospects, with significant growth expected through ongoing innovations in both its architecture and practical applications.
There are various domains for which we can discuss both the challenges and opportunities of Generative AI. Let’s start with challenges and their proposed solutions followed by opportunities-.
Ethical concerns are a major challenge in the development of generative AI, and understanding the issues is crucial for creating responsible AI systems.
Challenges and Proposed Solutions
Generative AI is a powerful tool, but it's not without its challenges. More than half of participants in a study still have concerns about its reliability and impact. The good news is that there are proposed solutions to these challenges.
Establishing ethical governance structures, guidelines, and regulations can guide the responsible development and deployment of GenAI. This is especially important to prevent malicious uses, such as creating deepfakes for identity theft or misinformation.
Developing security measures to protect generative models from manipulation is crucial. This involves continuous research into adversarial robustness to prevent vulnerabilities.
Generative models may amplify and perpetuate biases in the training data, leading to discriminatory and unfair outputs. To mitigate this, extensive research and implementation of methods to detect and mitigate bias in training data are necessary.
Data protection regulations and privacy-preserving approaches are essential to protect personal privacy. This involves adhering to regulations and implementing methods to protect sensitive information.
The 'black boxes' nature of generative algorithms makes it difficult to understand their decision-making process. Research and development of explainable AI approaches can improve interpretability and transparency, allowing users to understand algorithm outputs.
Worth a look: Generative Ai for Research
Frequently Asked Questions
Which paper is influential in generative AI?
The influential paper "Reinforcement Learning with Human Feedback" explores offline RLHF, a challenging domain in generative AI. This research sheds new light on learning dynamic choices via pessimism.
Is there an AI that can write research papers?
Yes, Paperpal is a leading AI writing assistant used by over 20,000 academics worldwide to help with research paper writing. It's endorsed by 13 top publishers and supports over 400 journals globally.
How do I find AI research papers?
You can find AI research papers on reputable online platforms such as Academia.edu, arXiv.org, and GitHub, which host a vast collection of papers and research studies in the field of artificial intelligence. These platforms allow you to search, browse, and download papers for free, making it easy to stay up-to-date with the latest AI research and developments.
Sources
- https://www.oecd-ilibrary.org/science-and-technology/oecd-artificial-intelligence-papers_dee339a8-en
- https://educationaltechnologyjournal.springeropen.com/articles/10.1186/s41239-023-00411-8
- https://midas.umich.edu/research/research-resources/generative-ai-hub/generative-ai-research-resources/
- https://link.springer.com/article/10.1007/s11042-024-20016-1
- https://arxiv.org/html/2405.11029v1
Featured Images: pexels.com