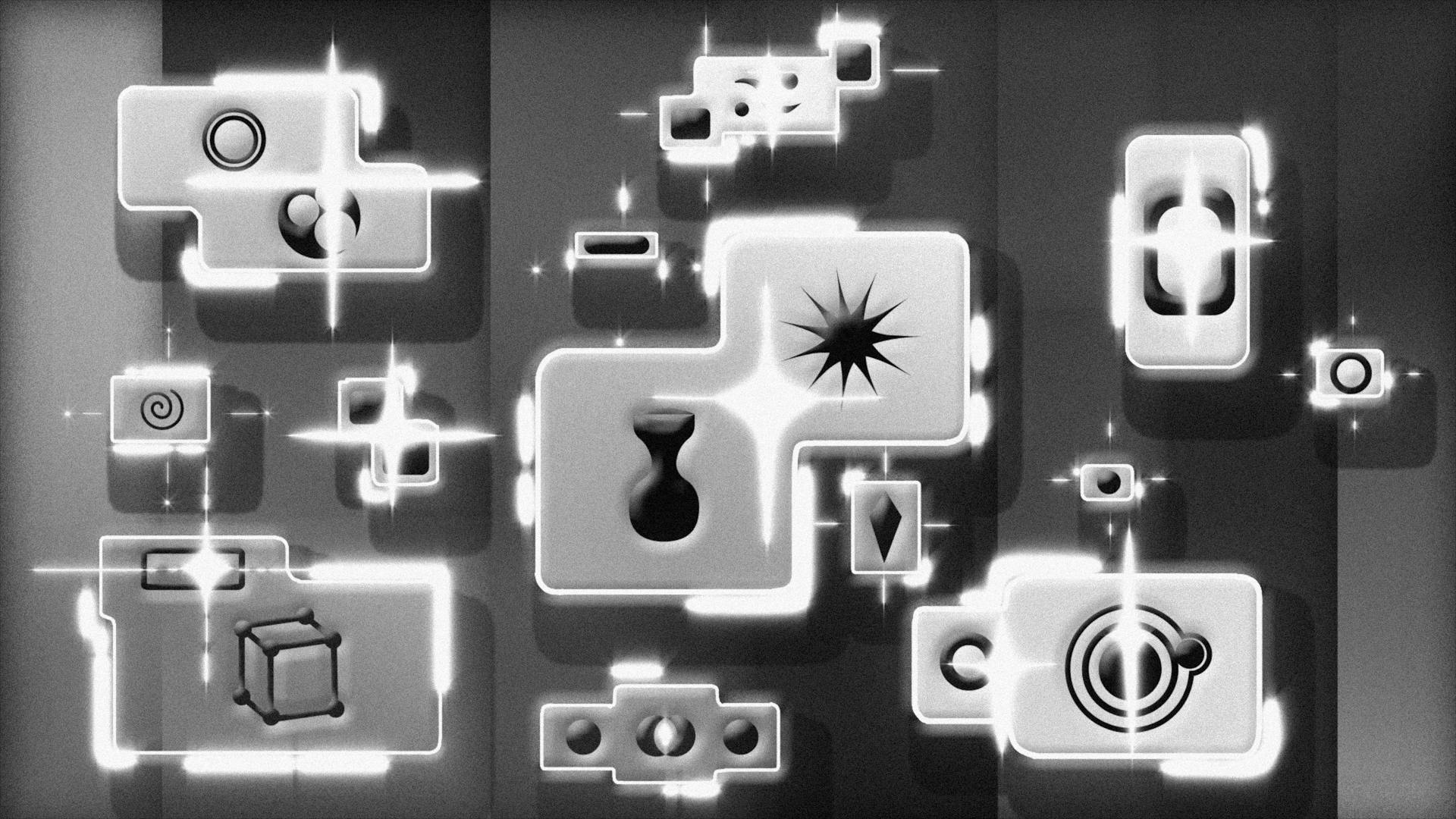
Generative AI has introduced new security risks, making it essential to stay informed about the latest threats.
The increased use of generative AI has led to a surge in deepfake attacks, which can be devastating for individuals and organizations.
Deepfakes can be used to create convincing fake videos, audio recordings, and even text documents, making it challenging to distinguish reality from fabrication.
As a result, organizations must implement robust security measures to detect and prevent deepfake attacks, including advanced AI-powered tools and human verification processes.
Generative AI has also made it easier for attackers to create highly realistic phishing emails and messages, making it difficult for users to identify legitimate communications.
To combat this, it's crucial to educate users about the dangers of phishing and to implement robust email authentication and verification protocols.
Worth a look: Create with Confidence Using Generative Ai
Risks and Concerns
Generative AI has introduced new security risks that users and providers should be aware of. These risks include information leakage and output of inappropriate information. To mitigate these risks, users should not input sensitive information and use services that don't use input information for learning. Providers should also consider multilayered countermeasures, such as limiting behavior with additional prompts and formal checks of I/O.
Discover more: Generative Ai Risks
Cyberattackers can intentionally generate inappropriate output by entering crafted prompts, known as adversarial prompts. This can lead to information leakage, inappropriate responses, and triggering unintended behavior of linked systems. To address these risks, providers should consider vulnerability testing, including implementation of adversarial prompts.
56% of organizations are vulnerable to gen AI-powered attacks, such as ransomware and phishing. Certain industries are more targeted due to their advanced technology landscapes. To address these risks, organizations need to update their security posture and embed security by design throughout their gen AI journey.
To assess the security risk level of generative AI, a comprehensive security assessment is necessary, informed by cyber intelligence. This evaluation should ensure alignment with industry best practices and consider the current security maturity within the gen AI environment.
The security risks of generative AI can be broken down into four distinct areas: information leakage, output of inappropriate information, malicious use, and hallucination. These risks can be mitigated by implementing multilayered countermeasures and ensuring that generative AI models have built-in protections against malicious use.
Explore further: Ai Security Training
CISO Role and Responsibilities
The CISO role has evolved significantly with the integration of new AI models into the mainstream workflow. As a result, the CISO is now responsible for managing security across a widely distributed network.
The CISO's job has grown to encompass handling generative AI security, which is a critical aspect of their role. In today's digital world, the CISO must stay up-to-date with the latest advancements in AI to ensure the security of their organization's systems and data.
Related reading: What Is the Role of Generative Ai in Drug Discovery
Balancing Security and Benefits
As generative AI continues to integrate into our daily lives, it's essential to strike a balance between its benefits and cybersecurity concerns. CISOs (Chief Information Security Officers) are facing new responsibilities to ensure the security of their organizations.
Generative AI models handle sensitive data, and CISOs must assess how these models comply with data protection laws and regulations. This is a critical concern, as data security and privacy are top priorities.
A different take: Generative Ai Security Risks
To mitigate risks, CISOs should implement robust access controls to ensure only authorized individuals have access to systems. This includes controlling who can create, modify, or delete data within the system.
Protecting AI models from tampering and reverse engineering is also crucial. This involves securely storing the models themselves and preventing unauthorized access. Think of it like locking your car – you wouldn't leave the keys in the ignition, right?
Logging and monitoring systems are essential for detecting and responding to security incidents. These systems help identify potential threats and allow teams to take swift action.
Training and awareness are also vital components of cybersecurity. CISOs should lead the charge on system training and raise awareness among employees and stakeholders about the potential risks and benefits of generative AI.
Here are the key areas CISOs should focus on to balance security and benefits:
- Data security and privacy
- Access control
- Model integrity and security
- Logging and monitoring
- Training and awareness
By prioritizing these areas, CISOs can ensure their organizations reap the benefits of generative AI while minimizing the associated risks.
Adoption and Implementation
Generative AI adoption in security has been slow due to concerns about model bias and lack of explainability.
Many organizations are still in the experimental phase, testing the waters with proof-of-concept projects to gauge the potential benefits and risks.
The use of generative AI in security is expected to increase as more organizations see the potential for improved threat detection and incident response.
Here's an interesting read: The Economic Potential of Generative Ai
Adoption Path Forward
Organizations should immediately start using methods like in-person trainings, online courses, and awareness workshops to educate employees on the potential risks of generative AI adoption.
Embedding these trainings into existing processes is a great way to ensure everyone is on the same page.
CISOs should prioritize establishing clear usage policies to evaluate the credibility of third-party AI solutions.
Assessment frameworks and diligence models are also essential for minimizing generative AI cybersecurity risk.
Clarifying what's acceptable versus unacceptable when using AI-generated content within the organization can help prevent larger issues.
Data Command Center Required
To effectively implement Generative AI, a Data Command Center is essential for ensuring the utmost privacy and security of sensitive data. This is crucial because Generative AI's ability to imitate human communication in almost any style prompts serious concerns about automated social engineering attacks.
A Data Command Center helps with a comprehensive inventory of data that exists, which is vital for responsible use of enterprise data. It's also necessary for contextual data classification to identify sensitive data/confidential data.
Having a Data Command Center enables contextual and automated controls around data, ensuring swift compliance with evolving laws. This is especially important for meeting data consent, residency, and retention requirements.
A Data Command Center also helps with inventorying all AI models to which data is being fed via various data pipelines, and governing entitlements to data through granular access controls, dynamic masking, or differential privacy techniques.
Here are the key components of a Data Command Center:
By implementing a Data Command Center, organizations can ensure the responsible use of Generative AI and protect sensitive data from unauthorized access or misuse.
Security Measures and Strategies
Implementing effective security measures is crucial when it comes to Generative AI systems. A robust AI governance framework is a great starting point, consisting of components like establishing ethical guidelines, ensuring data security and privacy, implementing accountability mechanisms, regulatory compliance, and proactive monitoring and assessment.
Organizations must prioritize data anonymization and encryption to safeguard private and sensitive data. Data anonymization removes or encrypts personally identifiable information from training datasets, while data encryption transforms data into unreadable text that can only be decoded using unique decryption keys.
Cybersecurity tools are essential for mitigating security risks posed by Generative AI. These tools can detect anomalies, unexpected events, or malicious activities in AI systems, allowing organizations to take preventative measures.
Here are the key components of a robust AI governance framework:
- Establish ethical guidelines
- Ensure data security and privacy
- Implement accountability mechanisms
- Regulatory compliance
- Proactive monitoring and assessment
Awareness training is also vital for organizations, covering the implications of GenAI model biases, ethical considerations around AI-generated content, and ways to identify security threats. This training helps prevent data breaches and misuse of GenAI tools.
Check this out: How Is Generative Ai Trained
Vulnerabilities
Gen AI exposes organizations to a broader threat landscape, making them more vulnerable to sophisticated attackers and new points of attack. 76% of executives believe attackers will have the advantage over defenders in the next two years.
Phishing attacks have increased by 76% in the last eighteen months, and cybercriminals are exploiting this trend by using Gen AI-powered phishing attacks. These attacks are often initiated through Gen AI-powered phishing, affecting local governments, education, manufacturing, and healthcare.
Gen AI-powered cyberattacks are on the rise, with a rise in ransomware attacks, and threat actors have been experimenting with dark LLMs to create python-based ransomware. This is distributed with high levels of obfuscation that increase its potential success.
Organizations are not prepared to handle the new risks associated with Gen AI, and most are not equipped to mitigate them. New capabilities such as shadow AI discovery, LLM prompt and response filtering, and specialized AI workload integration tests are now required to properly mitigate these new risks.
The key to gaining the upper hand will be embedding security by design, and organizations must quickly update their security posture to protect against AI-powered attacks and their own AI landscapes.
You might enjoy: Generative Ai Not Working Photoshop
Sources
- https://www.nttdata.com/global/en/insights/focus/security-risks-of-generative-ai-and-countermeasures
- https://www2.deloitte.com/us/en/pages/financial-services/articles/ciso-generative-ai-security.html
- https://www.accenture.com/us-en/insights/cybersecurity/secure-gen-ai
- https://www.ksolves.com/blog/artificial-intelligence/mitigating-generative-ai-security-risks-strategies-for-organizational-resilience
- https://securiti.ai/generative-ai-security/
Featured Images: pexels.com