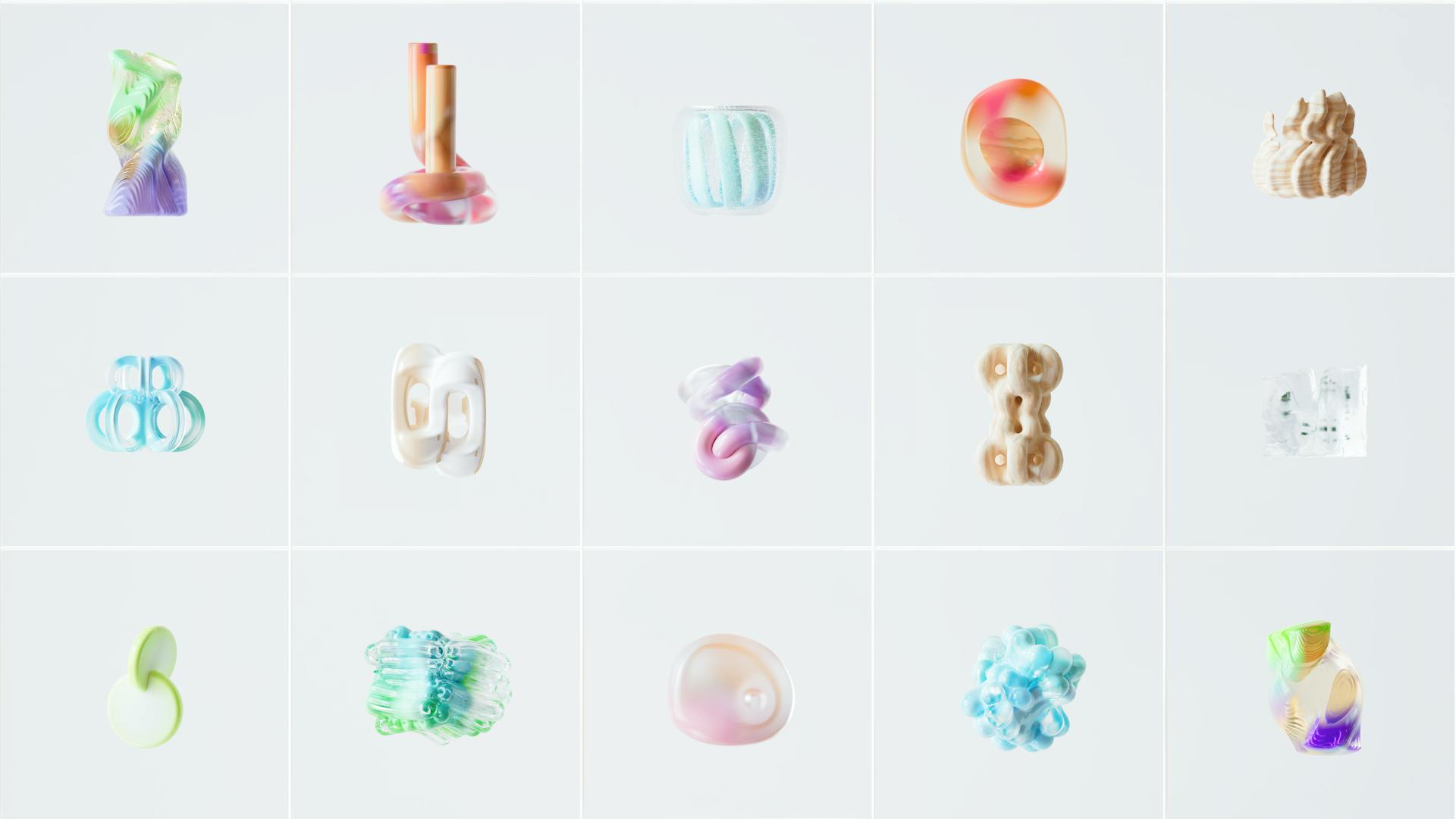
Intelligent machines are rapidly changing the way we live and work, with applications in healthcare, finance, and transportation.
These machines are already being used in hospitals to help doctors diagnose diseases more accurately.
Their ability to analyze large amounts of data and learn from experience makes them highly effective tools for medical professionals.
Machine learning algorithms are also being used in finance to detect and prevent cyber attacks.
In transportation, self-driving cars are being tested on public roads, with the potential to greatly reduce traffic accidents.
These intelligent machines are not just limited to these industries, but are also being used in education and customer service.
A unique perspective: How Is Ai Used in Robotics
History of Intelligent Machines
The field of artificial intelligence has a rich history that spans several decades. The first attempts to create intelligent machines date back to the beginning of computing.
Researchers in the 1950s and 1960s made significant strides in symbolic AI, which aimed to create intelligent machines using predefined rules and logical operations. This approach, however, faced limitations in handling real-world complexities.
The development of symbolic AI paved the way for the emergence of machine learning in the following decades. Machine learning focuses on the development of algorithms that allow machines to learn from data and improve their performance over time.
Expand your knowledge: Artificially Intelligent Art
What Makes Artificial Intelligence
Artificial intelligence is a field that's been around since 1956, when a conference at Dartmouth College rebranded the study of intelligent machines as "artificial intelligence".
There's no single definition of artificial intelligence that everyone agrees on, with over seventy different definitions provided by Legg and Hutter.
The field of artificial intelligence is concerned with the automation of intelligent behavior, as described by computer scientist Margaret Boden.
Boden also classifies five major types of AI, each with their own variations, including classical or symbolic "Good Old-Fashioned AI" (GOFAI) and artificial neural networks or connectionism.
Currently, none of these types of AI can approximate human intelligence in terms of general cognitive capacities, a level of intelligence referred to as artificial general intelligence or AGI.
AGIs should be capable of solving complex problems in various domains with autonomous control and thoughts, worries, feelings, strengths, weaknesses, and predispositions.
The AI we have now is of a narrower type, often called artificial narrow intelligence or ANI, with intelligence limited to the frame in which it is programmed.
Some intelligent machines can evolve autonomously through deep learning, but these are still a weak form of AI relative to human cognition.
Intriguing read: Artifical General Intelligence
Challenges and Critiques
Machine intelligence has made remarkable progress, but several challenges persist on the path to achieving truly intelligent systems. Data limitations are a major hurdle, as machine intelligence heavily relies on quality data for training and learning, but obtaining labeled and diverse datasets can be challenging.
Data scarcity or biases within the data can hinder the performance and generalizability of AI systems. This is a problem I've seen firsthand in my own work with AI, where a lack of diverse data can lead to biased results.
AI systems must be designed and deployed in ways that prioritize fairness, privacy, and human well-being. Addressing biases in training data, ensuring transparency, and establishing guidelines for responsible AI development are crucial to mitigate ethical challenges and potential societal risks.
Here are some of the key challenges in achieving machine intelligence:
- Data Limitations
- Interpretability and Trustworthiness
- Ethical Considerations
- Contextual Understanding and Common Sense
- Integration and Adoption
Challenges in Achieving
Achieving truly intelligent systems is a complex task, and several challenges persist on the path to getting there.
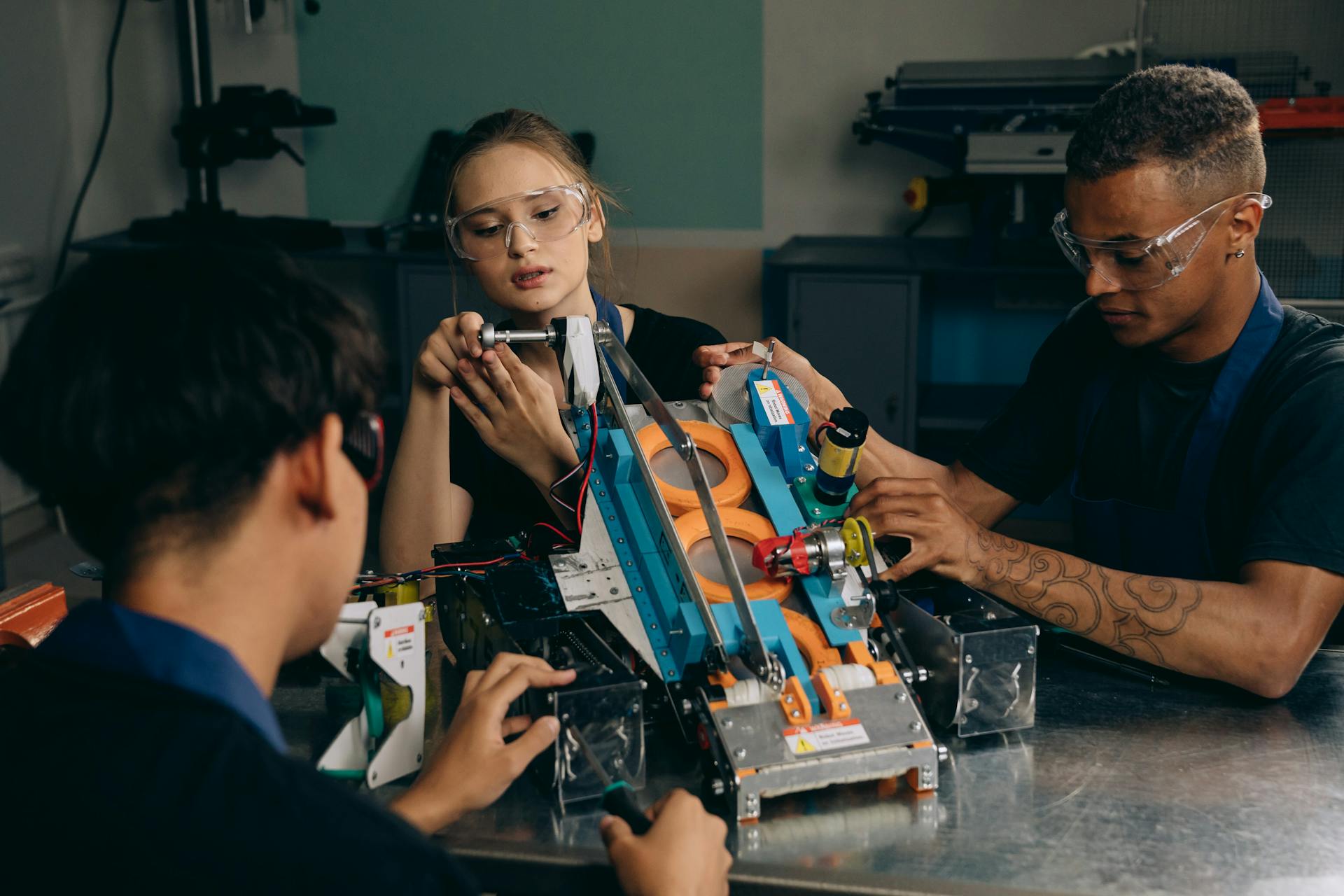
Data limitations are a major hurdle, as machine intelligence heavily relies on quality data for training and learning. Obtaining labeled and diverse datasets, particularly for complex tasks, can be challenging due to data scarcity or biases within the data.
Interpretability and trustworthiness are also significant concerns, as AI systems become less transparent in their decision-making processes. This lack of transparency makes it difficult for users to trust and rely on AI systems.
AI systems must be designed and deployed in ways that prioritize fairness, privacy, and human well-being. Addressing biases in training data, ensuring transparency, and establishing guidelines for responsible AI development are crucial to mitigate ethical challenges and potential societal risks.
The nervous system's continuous nature presents a challenge for discrete-state machines, which are often used to mimic its behavior. A small error in the nervous impulse can make a large difference in the outgoing impulse, making it difficult to predict the system's behavior.
Here are some of the key challenges in achieving machine intelligence, grouped by category:
- Data Limitations: Data scarcity, biases in training data
- Interpretability and Trustworthiness: Lack of transparency in decision-making processes
- Ethical Considerations: Biases in training data, lack of transparency, and potential societal risks
- Contextual Understanding and Common Sense: Lack of broader contextual understanding and common sense reasoning
- Integration and Adoption: Technological, organizational, and cultural barriers
Critique of the New Problem
The critique of the new problem of distinguishing between real and fabricated facts is a pressing concern, especially with the rise of AI-generated content.
As the Internet becomes increasingly littered with AI-generated content, the need for curation and safeguarding of high-quality data and information is more crucial than ever.
Distinguishing between real and fabricated facts has long been a societal challenge, and AI-generated content only exacerbates the issue.
The standard machine learning paradigm for assessing reliability is being rethought in light of foundation models entering healthcare.
Foundation models violate several basic principles of the standard machine learning paradigm, making it necessary to rethink what guarantees are required to establish warranted trust in them.
Researchers are proposing a cumulative set of three essential criteria for the ethical use of LLMs in academic writing, and a statement that researchers can quote when submitting LLM-assisted manuscripts.
New multi-modal AI methods are being developed to fuse different biological data types that span multiple scales, offering promising clinical utility.
Additional reading: New Artificial Intelligence Program
Here are some of the key challenges in distinguishing between real and fabricated facts:
- AI-generated content is becoming increasingly prevalent on the Internet.
- The standard machine learning paradigm for assessing reliability is being rethought.
- Foundation models violate several basic principles of the standard machine learning paradigm.
- New multi-modal AI methods are being developed to fuse different biological data types.
Heads in the Sand Objection
The "Heads in the Sand" Objection is a common concern people have about machines thinking. It's the idea that the consequences of machines thinking would be too dreadful, and we'd rather not think about it.
This argument is often rooted in a desire to believe that humans are superior to the rest of creation. Many people value the power of thinking highly and base their belief in human superiority on this power.
Intellectual people are more likely to be swayed by this argument, as they tend to value thinking more than others. This feeling of superiority is closely tied to the popularity of theological arguments.
Suggestion: Can Machines Think
Argument from Consciousness
The Argument from Consciousness is a challenge to the idea of artificial intelligence, suggesting that conscious beings are inherently more valuable than non-conscious ones. This argument relies on the assumption that consciousness is a fundamental aspect of existence.
Conscious beings have subjective experiences, such as feelings, emotions, and sensations, which are not replicable in artificial systems. The article mentions that consciousness is a complex and multifaceted phenomenon that is still not fully understood.
Some critics argue that the creation of conscious artificial intelligence would raise significant ethical concerns, such as the potential for exploitation or harm. The article highlights the importance of considering these ethical implications.
However, others argue that consciousness is not a fixed or essential property, but rather an emergent property of complex systems. This perspective suggests that consciousness could potentially arise in artificial systems, just as it does in biological ones.
The article cites the example of integrated information theory, which attempts to quantify consciousness in terms of integrated information generated by the causal interactions within the brain. This theory has implications for our understanding of consciousness in both biological and artificial systems.
Consciousness is a complex and multifaceted phenomenon that is still not fully understood, and the development of artificial intelligence that is conscious in the same way as humans is still a topic of debate.
A different take: Computational Learning Theory
Current Issue
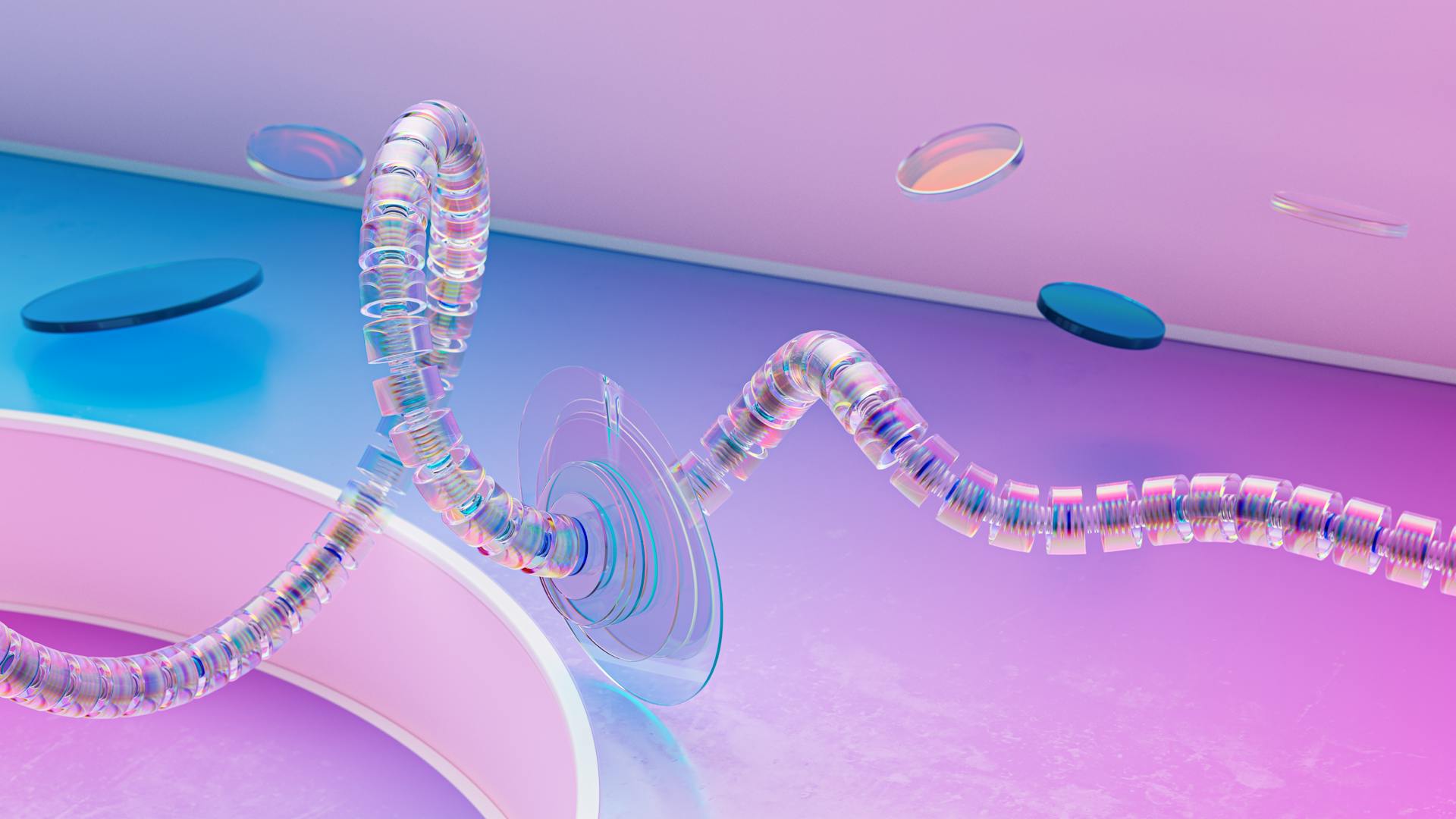
The Current Issue section of our publication highlights the ongoing discussions and debates in the field of artificial intelligence. The challenges in achieving machine intelligence are numerous, and it's essential to address them head-on.
Data limitations are a significant hurdle, as machine intelligence heavily relies on quality data for training and learning. Obtaining labeled and diverse datasets, particularly for complex tasks, can be challenging due to data scarcity or biases within the data.
The lack of interpretability and trustworthiness in AI systems is another pressing concern. As AI systems become more sophisticated, their decision-making processes become less transparent, making it difficult for users to trust and rely on them.
Ethical considerations are also a critical aspect of AI development. AI systems must be designed and deployed in ways that prioritize fairness, privacy, and human well-being. Addressing biases in training data, ensuring transparency, and establishing guidelines for responsible AI development are crucial to mitigate ethical challenges and potential societal risks.
A different take: Ethical Implications of Ai
Here are some recent developments in the field that highlight the need for responsible AI development:
The Current Issue section also highlights the challenges in integrating and adopting AI in various industries. Organizations face difficulties in identifying the right use cases, managing the transition, and upskilling their workforce to leverage AI effectively.
Machine Learning and Capabilities
Machine learning is a subset of artificial intelligence that enables intelligent machines to learn from data and improve their performance over time. This process allows machines to make decisions based on patterns and relationships in the data.
One of the key capabilities of machine learning is its ability to classify objects and predict outcomes. For example, a self-driving car can use machine learning to recognize traffic lights and pedestrians.
Machine learning can also be used for natural language processing, enabling intelligent machines to understand and respond to human language. This is achieved through complex algorithms that analyze patterns in language data.
Consider reading: Artificially Intelligent Robots
The accuracy of machine learning models can be improved through the use of large datasets and iterative training processes. This is why many intelligent machines rely on vast amounts of data to make informed decisions.
Machine learning can also be used to optimize complex systems, such as supply chains and logistics networks. By analyzing data and identifying patterns, intelligent machines can make recommendations to improve efficiency and reduce costs.
You might like: Intelligent Harmony Machine
Digital Computer Universality
Digital computers can solve any problem that can be solved by any other computer. This is known as digital computer universality.
The concept of universality was first proposed by Alan Turing in his 1936 paper "On Computable Numbers", where he described a theoretical machine called the Turing Machine. This machine can simulate the behavior of any other computer.
Turing's work laid the foundation for the development of modern computers and programming languages. Today, we have computers that can perform a wide range of tasks, from simple calculations to complex simulations.
If this caught your attention, see: Ai and Ml in Digital Marketing
The universality of digital computers is a key factor in their ability to learn and adapt. By using algorithms and data, computers can improve their performance over time, much like how humans learn from experience.
A computer's ability to solve any problem that can be solved by any other computer is a fundamental aspect of their design.
You might like: Learn to Rank Models
Applications and Future
Machine intelligence is transforming industries and enhancing human capabilities in numerous ways. It's amazing to see how AI is making a significant impact in various fields.
In healthcare, AI systems can analyze medical imagery like X-rays, MRIs, and CT scans to help diagnose diseases early on. This technology is a game-changer for patients and doctors alike.
AI systems in finance examine vast amounts of financial data to spot potential trends and abnormalities that can prevent fraud. Machine learning algorithms even predict market trends and optimize trading strategies.
Machine intelligence plays a vital role in the transportation sector, particularly in the development of autonomous vehicles. AI systems process sensor data, interpret traffic patterns, and make real-time decisions to improve road safety and efficiency.
AI systems in manufacturing analyze sensor data from machinery to identify potential failures and schedule maintenance proactively, minimizing downtime. Systems using machine vision check products for flaws to ensure high quality and cut down on waste.
Here are some of the major fields where machine intelligence is having a big impact:
- Healthcare: early disease diagnosis, patient engagement, and individualized healthcare suggestions
- Finance: fraud prevention, market trend prediction, and trading strategy optimization
- Transportation: autonomous vehicles, road safety, and efficiency
- Manufacturing: proactive maintenance, quality control, and inventory management
- Customer Service: personalized recommendations and bespoke experiences
- Entertainment: individualized content suggestions, content generation, and immersive experiences
The future of machine intelligence holds immense possibilities, with continued advancements in machine learning leading to breakthroughs in areas like natural language understanding, robotics, and cognitive computing.
Frequently Asked Questions
What is meant by intelligent machine?
An intelligent machine is one that can adapt to changing circumstances and make decisions in uncertain environments. It's able to sense its surroundings and adjust its actions accordingly, demonstrating a level of autonomy and problem-solving ability.
What is the most intelligent machine in the world?
Currently, the most intelligent machine in the world is a human brain, with its estimated 100 billion cells and unparalleled complexity.
Featured Images: pexels.com