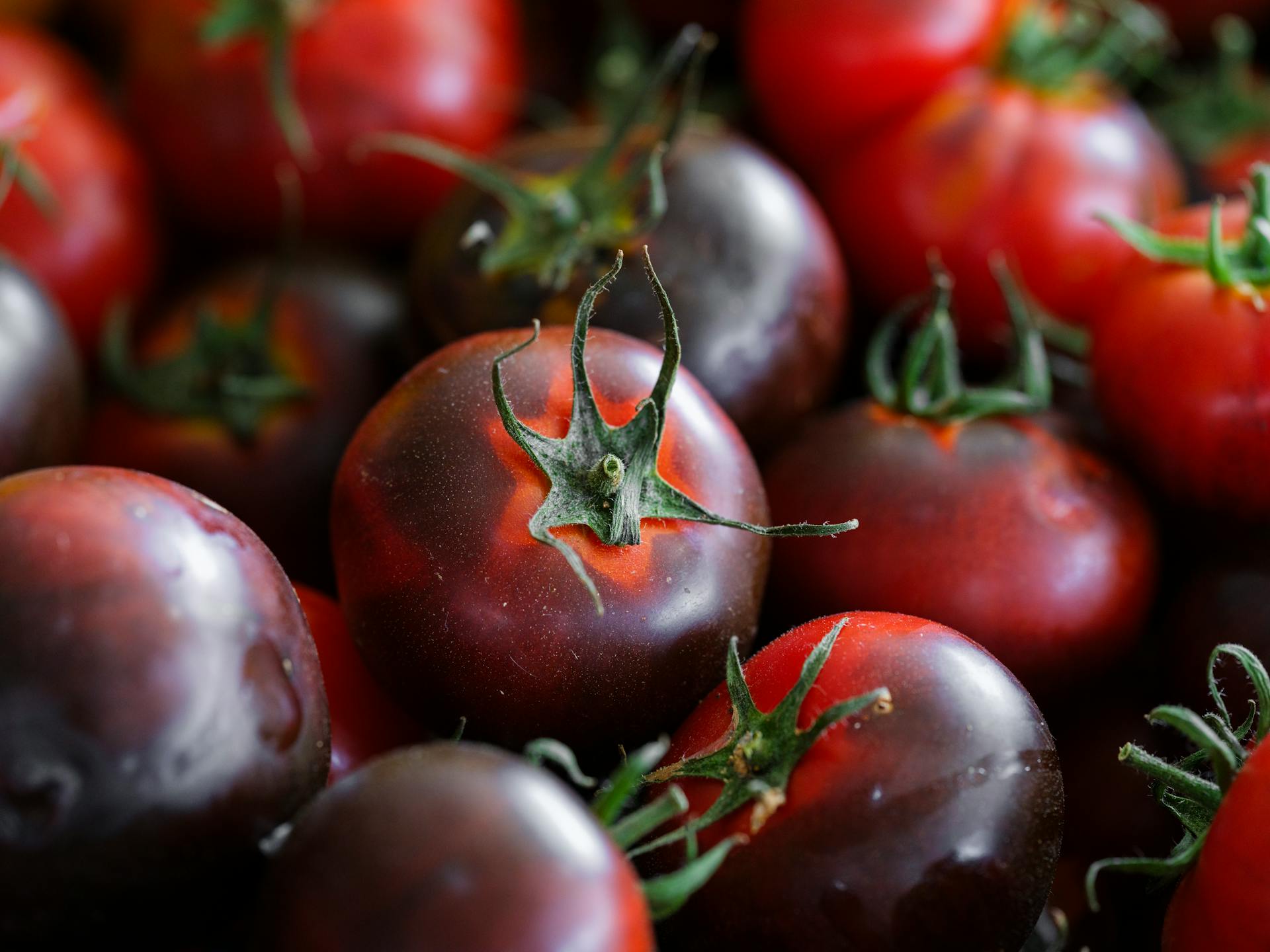
Nightshade AI poisoning is a serious threat to the integrity of artificial intelligence systems. It occurs when a malicious actor intentionally corrupts a model's data, causing it to produce biased or inaccurate results.
The risks of nightshade AI poisoning are real and have been demonstrated in several studies. In one experiment, researchers found that a single corrupted data point was enough to compromise the accuracy of a machine learning model.
As AI systems become increasingly integrated into our lives, the potential consequences of nightshade AI poisoning grow. This type of attack can have serious repercussions, from financial losses to compromised national security.
To mitigate the risks of nightshade AI poisoning, it's essential to implement robust defenses. These can include data validation and verification processes, as well as regular model audits to detect and address any potential issues.
On a similar theme: Ai Poisoning Attack
What is Nightshade AI Poisoning?
Nightshade AI poisoning is a tool that protects digital artwork from being used to train generative AI models without permission.
The software operates by tagging images at the pixel level using the open-source machine learning framework Pytorch.
These tags are not obvious to human viewers, but they cause AI models to misinterpret the content of the images, leading to the generation of incorrect or nonsensical images when these models are prompted to create new content.
The tool was developed as part of the Glaze Project, led by Professor Ben Zhao at the University of Chicago.
Nightshade is available for free, which aligns with the project's goal to increase the cost of training on unlicensed data and making licensing images from creators a more attractive option for AI companies.
The tags used by Nightshade are designed to make AI models see something completely differently while not changing much, if at all, of what humans actually see of the art.
This is achieved by leveraging the way AI models observe or extract features or patterns from images, which is very different from our own visual cortex.
On a similar theme: Ai Generative Models
Background
Nightshade AI poisoning is a form of attack that can occur in machine learning models, and it's essential to understand the background to grasp its implications. In this context, a poisoning attack refers to manipulating the training data to compromise the model's performance.
There are two primary training scenarios to consider: training a model from scratch or continuously training an existing model with additional data. This means that AI poisoning can happen at various stages of a model's development.
Types of Attacks
Nightshade AI poisoning can take many forms, and understanding the types of attacks is crucial to staying safe.
There are three primary types of attacks: Data Poisoning, Model Poisoning, and Adversarial Attacks.
Data Poisoning occurs when an attacker manipulates the training data, causing the model to learn incorrect patterns.
This can be done by adding fake data or altering existing data to mislead the model.
Model Poisoning involves an attacker manipulating the model itself, often by adding a backdoor that allows them to control the model's output.
Adversarial Attacks, on the other hand, involve an attacker creating inputs that are designed to mislead the model.
These inputs can be images, audio, or text, and are often crafted to be imperceptible to humans.
The goal of an Adversarial Attack is to cause the model to make incorrect predictions, often with malicious intent.
Explore further: Adversarial Ai
Attack Methods
Nightshade AI poisoning has two main attack goals: succeeding with fewer poison samples and avoiding human and automated detection.
The basic, dirty-label attack, which injects a mismatch between the image and text in each poison sample, falls short in this regard.
To increase poison potency, we considered extending existing designs to our problem context, but none proved to be effective.
We found that adding perturbations to images to shift their feature representations, used by existing works to disrupt style mimicry and inpainting, exhibited a limited poisoning effect.
In contrast, Nightshade is a highly potent and stealthy prompt-specific poisoning attack that reduces the poison samples needed for success by an order of magnitude.
Here are some key features of Nightshade:
- Succeeds with fewer poison samples
- Avoids human and automated detection
- Effectively corrupts the concept of an image
Nightshade achieves this by leveraging two intuitions: shifting the feature representation of the image and using a limited number of poison samples to corrupt the concept of the image.
Composability Attacks
Composability attacks are a type of attack that can be used to compromise AI models by poisoning them with malicious data. This can be done by adding changes to the pixels in a digital image that are invisible to the human eye, affecting both the image and the text or captions associated with it.
These attacks are particularly effective because they can be successful with a small number of samples, making them difficult to detect and filter out. In fact, Nightshade, a prompt-specific poisoning attack, can be successful with an order of magnitude fewer poison samples than basic dirty-label attacks.
The power of composability attacks lies in their ability to be used as a protection mechanism for intellectual property (IP). By applying a tool like Nightshade to copyrighted images, content owners can create a powerful disincentive for model trainers to respect opt-outs and do not crawl directives.
Here are some key characteristics of composability attacks:
By understanding the capabilities and limitations of composability attacks, we can better appreciate the importance of protecting our intellectual property and the role that tools like Nightshade can play in this effort.
Will It Work?
Nightshade is designed to give content creators a way to push back against unauthorized training of AI models. The aim is not to take down Big AI, but to force tech giants to pay for licensed work instead.
The tool's creators claim it can provide a powerful tool for content owners to protect their intellectual property. This is a response to model trainers that disregard or ignore copyright notices, don't-scrape/crawl directives, and opt-out lists.
Eva Toorenent, an illustrator, hopes Nightshade will change the status quo, making AI companies think twice before using others' work without consent. This is because they have the possibility of destroying their entire model by taking work without permission.
The real issue here is about consent and compensation. Autumn Beverly, another artist, believes Nightshade can help return the power back to artists for their own work.
Related reading: Generative Ai at Work
Frequently Asked Questions
What is an example of nightshade AI?
Nightshade AI creates false matches between images and text by subtly altering images, making them appear as one thing to humans but something else to AI, such as changing a dog into a cat. This technique can be used to confuse AI art generators and create unexpected results.
What does nightshade poisoning look like?
Nightshade poisoning symptoms include abdominal pain, vomiting, and skin irritation, progressing to severe symptoms like difficulty breathing, dilated pupils, and bloody urine in extreme cases
Sources
- https://theweek.com/tech/nightshade-data-poisoning-tool-ai
- https://arxiv.org/html/2310.13828v3
- https://venturebeat.com/ai/nightshade-the-free-tool-that-poisons-ai-models-is-now-available-for-artists-to-use/
- https://shellypalmer.com/2024/01/nightshade-poisoning-ai-training-sets/
- https://abc7chicago.com/generative-ai-image-generator-nightshade-university-of-chicago/14363327/
Featured Images: pexels.com