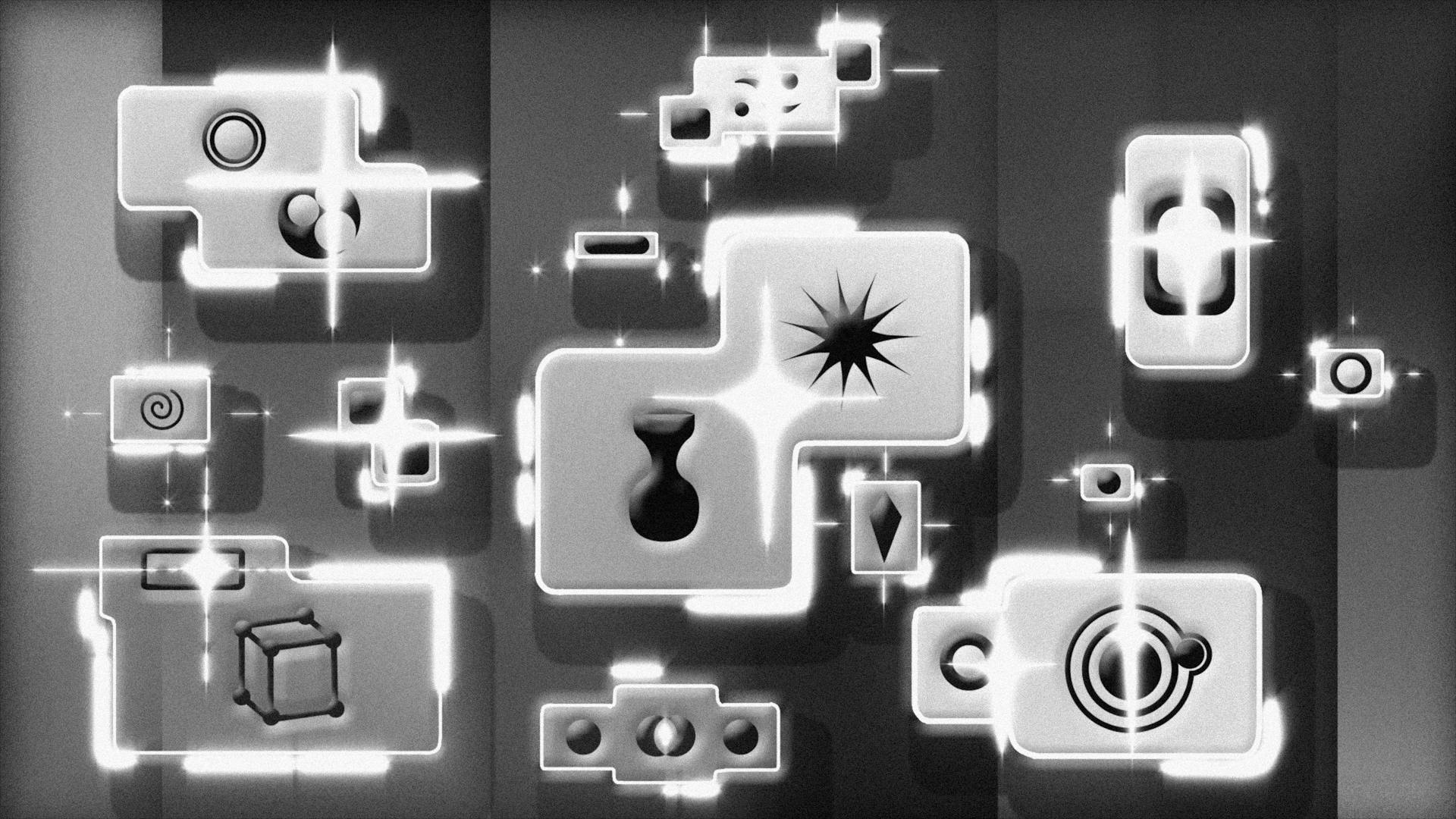
Generative AI is a type of artificial intelligence that can create new content, such as images, music, or text, based on a given prompt or set of parameters. This is in contrast to traditional AI, which is focused on processing and analyzing existing data.
Traditional AI systems are designed to perform specific tasks, like recognizing images or understanding natural language, but they don't have the ability to create new content. Generative AI, on the other hand, can generate entirely new data that didn't exist before.
The key difference between generative AI and traditional AI lies in their capabilities and applications. Traditional AI is often used in areas like customer service chatbots, image recognition, and natural language processing, while generative AI is being explored for its potential in creative fields like art, music, and writing.
Explore further: New Generative Ai
What Is
Generative AI is the next evolution of artificial intelligence, capable of producing text, video, images, and other types of content.
Generative AI models are fed vast quantities of existing content to train the models to produce new content, learning to identify underlying patterns in the data set based on a probability distribution.
Popular tools like ChatGPT, Bard, and DALL-E are all examples of generative AI, showcasing its ability to create something new.
Generative AI relies on machine learning to understand, predict, and create content from data, requiring a massive amount of data for it to function.
Machine learning provides the training that fuels the AI to produce its result, making it a crucial component of generative AI.
A unique perspective: Generative Ai for Content Creation
Types of AI
There are several types of AI, each with its own strengths and weaknesses. Narrow or weak AI is a type of AI that is designed to perform a specific task, such as image recognition or language translation.
This type of AI is often used in applications like virtual assistants and chatbots. For example, Siri and Alexa are examples of narrow AI, as they are designed to perform specific tasks like answering questions and controlling smart home devices.
The other main type of AI is general or strong AI, which is designed to perform any intellectual task that a human can. This type of AI is still in its infancy and is not yet widely available.
Readers also liked: Pre-trained Multi Task Generative Ai Models Are Called
Conversational
Conversational AI is a type of AI that allows us to interact with it using everyday language. It uses technologies like natural language processing (NLP) to understand questions and instructions, providing better responses. This makes interactions more natural and effortless, requiring no special training.
Conversational AI is not the same as generative AI, although they are related. Generative AI refers to AI systems that create new content based on learned patterns from existing data. Conversational AI, on the other hand, focuses on understanding and generating human-like responses to deliver meaningful interactions.
Large language models (LLMs) are a type of neural network that work by forecasting word sequences. They can now hold dialogues, write prose, and scrutinize enormous text quantities from the internet.
Conversational AI allows customers to interact with systems using normal human language, making technology more accessible and easier to use. Voice-activated assistants are prime examples of this.
Conversational AI can be used in various ways, including:
- Resolving customer queries and providing product information
- Guiding users through a process
- Allowing teams to interact with CRM systems naturally
Transformers are a type of deep learning model that are especially useful for processing language. They can analyze past behavior and provide personalized product recommendations, and generate automated but human-feeling responses.
Salesforce Artificial Intelligence
Salesforce Artificial Intelligence is a powerful tool that delivers trusted, extensible AI grounded in the fabric of the Salesforce Platform.
You can utilize Salesforce AI in your customer data to create customizable, predictive, and generative AI experiences that fit all your business needs safely.
Salesforce AI brings conversational AI to any workflow, user, department, and industry with Einstein.
With Salesforce AI, you can create AI experiences that are tailored to your specific business needs, making it a highly versatile tool.
Salesforce AI is designed to be safe and secure, ensuring that your business data is protected at all times.
The Salesforce AI platform is built on the Salesforce Platform, making it a seamless and integrated part of your existing business systems.
A unique perspective: Create with Confidence Using Generative Ai
Key Concepts
Generative AI is designed to create new content, such as text, images, and music, using existing data. This is in contrast to traditional AI, which focuses on analyzing and interpreting data to improve efficiency, accuracy, and decision-making.
Generative AI models, like GPT-4, require substantial computational resources and large datasets for training, making them more difficult to scale compared to traditional AI. Traditional AI, on the other hand, can often operate effectively with smaller datasets, depending on the complexity of the task and the model used.
Generative AI has different use cases than traditional AI. While traditional AI is found in finance, healthcare, and manufacturing, generative AI is used in music, design, and marketing. Generative AI is also used in scientific research where new hypotheses and models are needed.
Here are some key differences between generative AI and traditional AI:
- Focus and Output: Generative AI creates new content, while traditional AI analyzes and interprets data.
- Implementation: Generative AI is used in creative fields and scientific research, while traditional AI is used in applications like predictive analytics and natural language processing.
- Transparency: Traditional AI models are generally more transparent and interpretable, while generative AI models can function as "black boxes."
- Performance and Efficiency: Generative AI models require substantial computational resources and training time, while traditional AI can be more efficient.
- Data Requirements and Training: Generative AI typically requires large datasets for training, while traditional AI can often operate with smaller datasets.
- Adaptability and Flexibility: Generative AI can adapt to various domains and generate content across different fields, while traditional AI often needs specific training for each unique task or application.
Terms
Artificial intelligence (AI) is a broad field that encompasses various subfields, including traditional AI and generative AI. Traditional AI focuses on analyzing and interpreting existing data to improve efficiency, accuracy, and decision-making within predefined boundaries.
Generative AI, on the other hand, is designed to create new content, such as text, images, music, and models, based on existing data. It's used in creative fields like content creation, design, and entertainment, as well as scientific research where new hypotheses and models are needed.
Some key terms to understand in the context of AI include:
- Artificial neural network: a type of machine learning model inspired by the structure and function of the human brain
- Augmented intelligence: the use of AI to enhance human capabilities
- Deep learning: a subfield of machine learning that involves the use of neural networks with multiple layers
- Generative AI: a type of AI that creates new content based on existing data
- Generator: a type of model used in generative AI to generate new content
- GPT: a type of language model developed by OpenAI
- Machine learning: a type of AI that involves training models on data to make predictions or decisions
- NLP: a subfield of machine learning that involves the use of AI to process and understand human language
- Parameters: numeric values adjusted during training to minimize the difference between a model's predictions and actual outcomes
- Transformer: a type of neural network architecture used in natural language processing
Parameters play a crucial role in shaping the generated content and ensuring that it meets specific criteria or requirements. They define the model's structure and behavior, helping it to recognize patterns and predict what comes next when generating content.
Explore further: Generative Ai Content
Pre-Trained Transformer
A pre-trained transformer is a type of neural network that's trained to generate content based on large amounts of text data. This means it can create clear and relevant text based on user prompts or queries, making it a powerful tool for businesses.
One of the key benefits of pre-trained transformers is that they can be used to automate the creation of customer-facing content, such as chatbot responses or product recommendations. This can be especially helpful for businesses with limited resources or a large customer base.
Pre-trained transformers can also analyze customer feedback and extract insights, helping businesses to better understand their customers' needs and preferences.
Explore further: Generative Ai Text Analysis
Here are some key features of pre-trained transformers:
- Large context windows: Pre-trained transformers can process and consider a large number of tokens (words or parts of words) simultaneously, making them well-suited for handling large attachments or prompts.
- Improved customer interactions: By generating personalized and relevant content, pre-trained transformers can enhance the customer service experience and provide a more engaging form of communication.
- Automated content creation: Pre-trained transformers can be used to automate the creation of customer-facing content, freeing up human resources for more strategic tasks.
Overall, pre-trained transformers have the potential to revolutionize the way businesses interact with their customers and create content. By leveraging their ability to generate personalized and relevant text, businesses can build stronger relationships with their customers and stay ahead of the competition.
Hallucination
Hallucination is a phenomenon where generative AI produces new content that doesn't correspond to reality or its training data. This can happen when an AI model analyzes the content given to it but comes to an erroneous conclusion.
An example of hallucination is when an AI model trained on thousands of photos of animals generates a new image by combining the head of a giraffe with the trunk of an elephant. This is an undesirable outcome that indicates a problem in the generative model's outputs.
Monitoring for and addressing hallucinations is crucial for ensuring the accuracy and reliability of AI systems. Quality assurance will still be an important part of an AI team, and addressing hallucinations helps improve the customer experience and software reliability.
Discover more: What Are Some Factors That Can Cause Hallucinations in Ai
For customers, companies that monitor for and address hallucinations in their software provide a better and more reliable experience. For teams, addressing hallucinations ensures the accuracy and reliability of AI systems.
Here's a breakdown of what hallucination means for customers and teams:
- For customers: A better and more reliable experience due to companies monitoring and addressing hallucinations.
- For teams: Ensuring the accuracy and reliability of AI systems by monitoring for and addressing hallucinations.
Chain of Thought Prompting
Chain of Thought Prompting is a technique that improves the accuracy of answers generated by large language models (LLMs) by using a series of intermediate reasoning steps.
This technique is also known as Chain-of-thought prompting, and it's a way to help LLMs think more clearly and logically. By breaking down a problem into smaller steps, you can get more accurate and applicable answers.
The goal of Chain of Thought Prompting is to mimic the way humans think and reason, by asking a series of questions that lead to the final answer. This can be especially helpful when working with complex or open-ended questions.
Here's an example of how Chain of Thought Prompting can be used:
By using Chain of Thought Prompting, you can ask a large language model to generate a more accurate and applicable answer, which can lead to a better end-user experience.
Zone of Proximal Development (ZPD)
The Zone of Proximal Development (ZPD) is an education concept that helps students progress in their learning. It's all about gradually increasing the difficulty level to build skills and confidence.
In machine learning, ZPD is applied when models are trained on progressively more difficult tasks, allowing them to improve their ability to learn.
This concept can be applied to employee training, enabling employees to learn complex tasks or make better use of the CRM's features.
By training generative AI properly, you're more likely to get accurate results, which is great news for anyone using AI tools.
Emergent Behavior
Emergent Behavior is a fascinating phenomenon where AI systems exhibit unexpected skills and talents. Generative AI models, in particular, can showcase emergent behaviors such as coding, musical composition, and poetry crafting.
These talents are not explicitly programmed into the AI system, but rather emerge from the complex interactions of its components. For example, vast language models can create fictional narratives, a skill that was not anticipated by their developers.
Check this out: Generative Ai Not Working Photoshop
The emergence of these skills is a result of the AI system's ability to learn and improve through large datasets and significant computational resources. This is in contrast to traditional AI, which tends to focus on analyzing and interpreting data.
Here are some examples of emergent behaviors exhibited by AI systems:
- Coding: AI systems can generate code in various programming languages.
- Musical composition: AI models can create original music and even compose music in different styles.
- Poetry crafting: AI systems can generate original poetry and even create entire books of poetry.
- Fictional narrative creation: AI models can create entire stories and even entire novels.
These emergent behaviors have far-reaching implications for various industries, from music and design to marketing and more. As AI technology continues to advance, it's likely that we'll see even more unexpected and innovative applications of AI.
How it Works
Traditional AI systems are trained on large datasets of labeled data, learning to identify patterns and make predictions or generate outputs.
Traditional AI uses various approaches, including expert systems, decision trees, and natural language processing (NLP). Expert systems, for example, can be used to diagnose diseases or troubleshoot technical problems, while decision trees can be used to decide whether to approve a loan application.
Readers also liked: How Are Modern Generative Ai Systems Improving User Interaction
Here are some examples of traditional AI approaches:
- Expert systems: diagnose diseases, troubleshoot technical problems, or provide financial advice
- Decision trees: decide whether to approve a loan application or recommend a product to a customer
- Natural language processing (NLP): used in search engines, chatbots, and machine translation systems
These approaches can be used alone or in combination to achieve specific goals, but they have limitations and can't create new data or outputs on their own.
GAN Discriminator
In a Generative Adversarial Network (GAN), the discriminator is like a detective that has to guess which pictures are real and which are fake.
The discriminator's job is to get better at telling real from fake, while the generator tries to get better at creating fakes, like continuously building a better mousetrap.
The discriminator is shown pictures, or other data, from a dataset and fake ones created by the generator. It has to evaluate the quality of synthetic data or content.
Using discriminators in GANs leads to a more secure customer experience through improved fraud detection. This is because the discriminator gets better at telling real from fake.
Discriminators in GANs helps teams evaluate the quality of synthetic data or content and aid in fraud detection and personalized marketing.
Here are some key benefits of using discriminators in GANs:
- Improved fraud detection for a more secure customer experience
- Helps teams evaluate the quality of synthetic data or content
- Aids in fraud detection and personalized marketing
How Does Work?
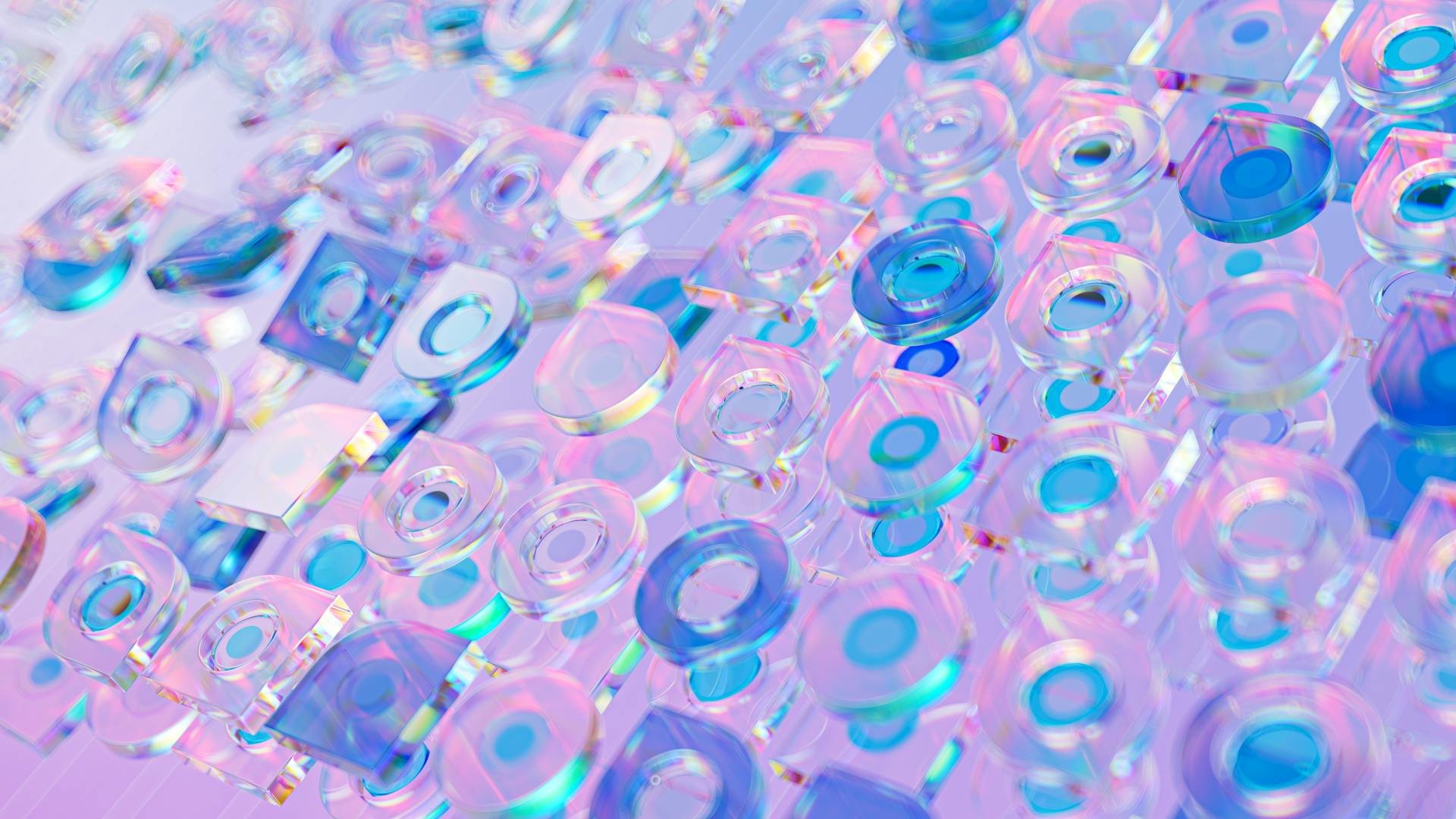
Traditional AI systems are trained on large datasets of labeled data, which helps them identify patterns and make predictions or generate outputs. This is how expert systems, decision trees, and natural language processing (NLP) systems work.
Expert systems, for instance, are designed to emulate the knowledge and expertise of human experts in a specific field, such as diagnosing diseases or troubleshooting technical problems. This can be incredibly helpful in fields like healthcare and technology.
Decision trees, on the other hand, use a set of rules to make decisions, like approving loan applications or recommending products to customers. I've seen decision trees used in customer service chatbots to provide personalized recommendations.
NLP systems, which are used in search engines, chatbots, and machine translation systems, are able to understand and generate human language. This is a key feature of many AI-powered tools that help us communicate more effectively.
Here are some examples of how traditional AI systems work:
- Expert systems: Diagnosing diseases, troubleshooting technical problems, or providing financial advice.
- Decision trees: Approving loan applications, recommending products to customers, or making other decisions based on rules.
- Natural language processing (NLP): Search engines, chatbots, and machine translation systems.
Sources
- https://researchguides.library.syr.edu/c.php
- https://www.salesforce.com/artificial-intelligence/generative-ai-glossary/
- https://www.eweek.com/artificial-intelligence/generative-ai-vs-ai/
- https://www.uschamber.com/co/run/technology/traditional-ai-vs-generative-ai
- https://mitsloanedtech.mit.edu/ai/basics/glossary/
Featured Images: pexels.com